xuebaunion@vip.163.com
3551 Trousdale Rkwy, University Park, Los Angeles, CA
留学生论文指导和课程辅导
无忧GPA:https://www.essaygpa.com
工作时间:全年无休-早上8点到凌晨3点
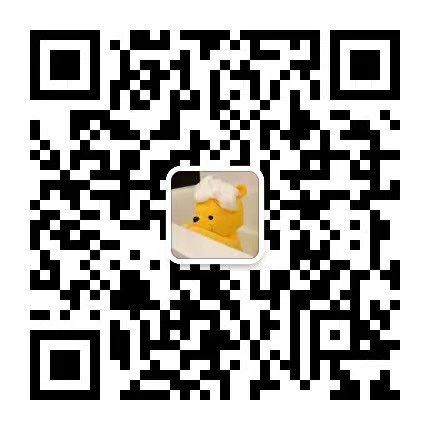
微信客服:xiaoxionga100
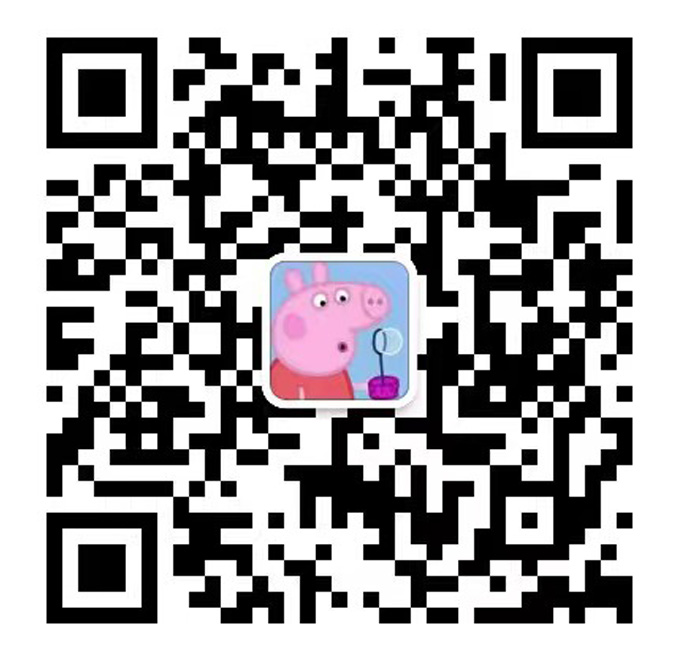
微信客服:ITCS521
SMU Classification: Restricted 1 SMU Classification: Restricted ISSS602 DATA ANALYTICS LAB ASSIGNMENT 1: Show Me the Numbers The Complete Journey An analysis of consumer shopping behaviour at supermarkets Prepared by: DATE: 28 JAN 2021 SMU Classification: Restricted 2 SMU Classification: Restricted Table of Contents 1 OVERVIEW ................................................................................................................................ 4 2 OBJECTIVES ............................................................................................................................... 4 3 DATA ......................................................................................................................................... 4 3.1 Data Used .......................................................................................................................... 4 3.2 Data Preparation ................................................................................................................ 4 3.3 Data Quality Issues Identified ............................................................................................. 5 3.3.1 Inaccurate data types ................................................................................................. 5 3.3.2 COUPON_UPC is not unique to household and campaign ........................................... 5 3.3.3 Large number of household keys unidentified ............................................................ 6 3.3.4 No indication of study start day or date ...................................................................... 6 3.3.5 Missing/unknown values ............................................................................................ 6 3.3.6 Too many levels for categorical variables .................................................................... 7 3.3.7 Inconsistent and invalid data ...................................................................................... 9 3.3.8 Extreme Outliers ........................................................................................................ 9 4 INSIGHTS ................................................................................................................................. 11 4.1 Shopping Behaviours ........................................................................................................ 11 4.1.1 The peak period for the stores is between late afternoon to evening........................ 11 4.1.2 Transactions and total sales value are higher on day 4 and 5 of the week ................. 11 4.1.3 50% of the households have shopped more than 74 times ....................................... 12 4.1.4 75% of the households made a purchase in the last 20 days ..................................... 13 4.1.5 90% of the households are customers for more than 567 days ................................. 13 4.1.6 Sales improved initially but flat after week 16........................................................... 14 4.2 Household Demographics and their shopping behaviours ................................................ 15 4.2.1 Bigger households spend more on average per basket ............................................. 15 4.2.2 Middle aged households spend more on average per basket .................................... 15 4.2.3 Higher income households spend more on average per basket ................................. 16 4.2.3.1 Does average spending differ for different household income? (Hypothesis Testing) . 16 4.2.4 Elderly shop at the supermarket earlier and are more flexible with their timing ....... 19 4.3 Marketing Campaigns and their impact on shopping behaviours ...................................... 19 4.3.1 Type A campaigns have higher coupon redemption rate ........................................... 19 4.3.1.1 Does coupon redemption rate differ for campaign types? (Hypothesis testing) ......... 20 4.3.2 Product transactions with coupon discount have higher Sales Value ......................... 20 4.3.3 Households that have loyalty card discount spends more in a basket ....................... 22 4.3.3.1 Is sales value of basket correlated to loyalty card discount? (Hypothesis testing) ...... 24 5 INTERPRETATION OF RESULTS ................................................................................................ 25 SMU Classification: Restricted 3 SMU Classification: Restricted Appendix A – Dataset Used ......................................................................................................... 26 Appendix B – Preparation Log of Original Data ........................................................................... 28 Appendix C – Preparation Log of New Tables.............................................................................. 32 SMU Classification: Restricted 4 SMU Classification: Restricted 1 OVERVIEW Digitalisation have changed shopping behaviours and supermarket chains are facing disruption from e-commerce. This trend was accelerated by the recent pandemic outbreak where market share of online grocery jumped from 2.7% in 2018 to 10.2% in 2020, and this is projected to increase to 21.5% by 2025 (Mercatus, 2020)1. Supermarket operators can however stay ahead of this disruption by understanding their customers and making business decisions with these customer insights. Retailers have been collecting valuable information on transactions, customer demographics and habits but these need to be translated into actual insights that can be leveraged upon to improve operations and sales. 2 OBJECTIVES The main objective is to understand customers’ shopping behaviours at supermarkets and allow operators to act on these insights to improve their operations and achieve higher return on their marketing efforts. We would be examining the transactions behaviour of customers to identify any relationships between shopping habits and their demographics or marketing campaigns. This analysis was conducted with JMP Pro 15 for data preparation, discovery of insights using interactive data exploration techniques, followed by validation of our findings with confirmative data analysis. 3 DATA 3.1 Data Used The original datasets were extracted from Dunnhumby’s dataset, “The Complete Journey”2 which contained eight datasets that tracked transactions of 2,500 households at a supermarket chain. In addition, four new datasets were created using JMP Pro 15 to facilitate the analysis. A description of all datasets used is found in APPENDIX A. 3.2 Data Preparation Given that the eight original datasets are not identical in structures, all the datasets were imported into JMP to be cleaned and transformed individually. A preliminary inspection of the summary statistics and distribution of all the variables was conducted to discover any potential data issues. We removed variables that are not important to our study and excluded any entries with missing value or extreme outliers. Some variables were recoded to be more meaningful for our analysis. A detailed preparation log of these variables is found in APPENDIX B. 1 Mercatus (2020). EGrocery Adoption: The New Reality for Grocery Shopper Behavior. Retrieved February 02, 2021, from https://info.mercatus.com/egrocery-shopper-behavior-report 2 https://www.dunnhumby.com/source-files Commented [KTS1]: Avoid painting a picture which is not in line with the original intent of the data and study. SMU Classification: Restricted 5 SMU Classification: Restricted The datasets were then transformed using Join, Summary and Update functions of JMP Pro 15 to create new datasets that helped to identify relationships from different perspectives. A detailed preparation log for these new datasets is found in APPENDIX C. 3.3 Data Quality Issues Identified 3.3.1 Inaccurate data types Many variables when imported into JMP from the original csv file have wrong modelling types. The values are in numeric format but should be categorical modelling types as they did not have any meaning as measurements. We changed these to either nominal or ordinal modelling type depending on whether the variable can be ordered. Table 1: Changing Modelling Type of Variables 3.3.2 COUPON_UPC is not unique to household and campaign Based on the data description, COUPON_UPC should be unique to household and campaign. However, our analysis revealed that this is not the case for the coupon and coupon_redempt datasets. We retained these 2 datasets as it is the best available data that we have on coupons, but we only used variables campaign and household_key to identify coupon redemptions. Example of a coupon with non-unique value: Table 2: COUPON_UPC are not unique to house_key and campaign SMU Classification: Restricted 6 SMU Classification: Restricted 3.3.3 Large number of household keys unidentified We have transaction data for 2500 households but only have demographics data on 801 households. This limits our analysis to less than a third of the 2500 households that have been identified as frequent shoppers and may skew our analysis. 3.3.4 No indication of study start day or date As there were no start dates or day given, we cannot identify whether a transaction occured on a weekday or weekend. We created a new column by taking the remainder of the DAY variable to categorise all transactions into different days of the week. Figure 1: Formula for Day of Week 3.3.5 Missing/unknown values There were many variables with missing or unknown values in hh_demographics and transaction_data. Due to the large percentage and nature of these missing/unknown values, the respective columns and rows were excluded from our analysis. • 344 records in MARITAL_STATUS_CODE are unknown (42.9% of available data) Figure 2: Distribution of MARITAL_STATUS_CODE • 233 records in HOMEOWNER_DESC are unknown (29.1% of distribution). Remaining data are heavily skewed towards homeowners. Figure 3: Distribution of HOMEOWNEER_DESC SMU Classification: Restricted 7 SMU Classification: Restricted • 558 records in KID_CATEGORY_DESC are none/unknown (69.7% of available data). Figure 4: Distribution of KID_CATEGORY_DESC • 14446 records in Quantity have 0 quantity. All transactions should be >0. • 18879 records in SALES_VALUE are 0 or <0.01. All transactions should be >0 in sales value. We will exclude values <0.01 as they are small numbers that are close to zero. 3.3.6 Too many levels for categorical variables Some categorical variables have too many levels and may impede our analysis. We excluded those variables that are not important and recoded those that are useful into lesser levels. • DEPARTMENT has 43 levels: information not used • COMMODITY_DESC has 307 levels: information not used • SUB_COMMODITY_DESC has 2382 levels: information not used • CURR_SIZE_OF_PRODUCT has 4344 levels: information not used • INCOME_DESC has 12 levels: recoded to 5 levels Figure 5: Distribution of INCOME_DESC Figure 6: Distribution of INCOME_DESC_Recoded Commented [KTS2]: If you refer to this figure,then the logical interval should be 0-24k, 25-49k,50-74k, 75-99, and 100 and above. This will give the first four bins a 25k interval. Less worry, no marks will be deducted. It is just my responsibility to point out area for further improvement. SMU Classification: Restricted 8 SMU Classification: Restricted • display has 10 levels: recoded to 2 levels Figure 7: Distribution of display Figure 8: Distribution of display_Recoded • mailer has 11 levels: recoded to 2 levels Figure 9: Distribution of mailer Figure 10: Distribution of mailer_Recoded SMU Classification: Restricted 9 SMU Classification: Restricted 3.3.7 Inconsistent and invalid data Some data are inconsistent or invalid and needs to be excluded from the analysis. • HH_COMP_DESC is inconsistent with MARITAL_STATUS_CODE and HOUSEHOLD_SIZE_DESC. Single Female and Male are recorded as married or having household size >1. As the same information can be represented with HOUSEHOLD_SIZE_DESC, HH_COMP_DESC will be excluded. Figure 11: Scatterplot of HH_COMP_DESC grouped by MARITAL_STATUS_CODE Figure 12: Scatterplot of HH_COMP_DESC grouped by HOUSEHOLD_SIZE_DESC • Customer_Paid has 5819 entries <0. This is invalid as it meant that customers got paid for buying the product. • RETAIl_DISC has 36 rows with values >0. All discount values should be negative. • COUPON_MATCH_DISC has 23 rows with values greater than COUPON_DISC. Retailer should only be matching up to the discount given by manufacturer. 3.3.8 Extreme Outliers • Quantity has 23,136 rows with value >100. Upon investigation, these transactions belong to products under Gasoline, and we suspect that the high quantity is due SMU Classification: Restricted 10 SMU Classification: Restricted to different mode of measurement. 99% of the data have quantity <100 so we will exclude all entries >100. Figure 13: Distribution of Quantity • Item_Unit_Price has 42,070 rows with value <0.01. These are excluded as each unit of product is expected to be at least 0.01. Figure 14: Distribution of Item_Unit_Price • SALES_VALUE has 1779 rows with values >=50. These are excluded as 99.5% is <=29. Figure 15: Distribution of SALES_VALUE Commented [KTS3]: This can’t be considered outliers. They are in different unit of measurements. SMU Classification: Restricted 11 SMU Classification: Restricted 4 INSIGHTS 4.1 Shopping Behaviours 4.1.1 The peak period for the stores is between late afternoon to evening We grouped all transactions in transaction_data by basket to analyse each basket transaction. Looking at the distribution of basket transaction timings, we observed that the stores have the most transactions in late afternoon to evening with the peak between 5pm and 6pm. There are also little transactions after midnight, with transactions only picking up after 6am. This insight is consistent with an editorial (Cain & Jiang, 20203) published on in- store traffic data for grocery stores, where the studies reviewed found that grocery stores are least crowded in early morning and most crowded between 3pm to 7pm. Figure 16: Distribution of transaction timing of all the baskets 4.1.2 Transactions and total sales value are higher on day 4 and 5 of the week The dataset did not state any start dates or the day of the week. However, when analysing the transactions grouped by baskets, there were 2 consecutive days of the week that had higher transaction counts and total sales value. Although there is a high chance that these 2 days fall on the weekend, we cannot conclude without further evidence. 3 Cain, Á, & Jiang, I. (2020). The best time to shop for groceries during the coronavirus pandemic is early morning, studies show [Editorial]. Business Insider. Retrieved January 29, 2021, from https://www.businessinsider.com/morning-is-best-time-to-shop-groceries-without-crowds-2020-3 SMU Classification: Restricted 12 SMU Classification: Restricted Figure 17: Transaction counts by day of the week Figure 18:Transaction Sales Value by day of the week 4.1.3 50% of the households have shopped more than 74 times Most of the households return to the supermarket chain to purchase their groceries, with 50% going back at least 74 times in 711 days. This meant that these households on average will return to make a purchase every 10 days. Figure 19: Distribution of the total transactions count of each household SMU Classification: Restricted 13 SMU Classification: Restricted 4.1.4 75% of the households made a purchase in the last 20 days We observed that 75% of households had purchased groceries in the last 20 days. This is important for the business as customers who made purchases recently are more likely to purchase again.4 Figure 20: Distribution of the number of days from the last shopping visit of households 4.1.5 90% of the households are customers for more than 567 days 90% of the households are long-time customers, having shopped at the supermarket for more than 567 days. Figure 21: Distribution of the duration that households have shopped at the supermarket chain 4 Segal, T. (2019). Inside Recency, Frequency, Monetary Value (RFM). Retrieved January 29, 2021, from https://www.investopedia.com/terms/r/rfm-recency-frequency-monetary-value.asp SMU Classification: Restricted 14 SMU Classification: Restricted 4.1.6 Sales improved initially but flat after week 16 We noticed that weekly sales picked up strongly initially but stayed flat after week 16. Average sales value per basket transaction remained largely the same over time. Figure 22: Supermarket sales over time Figure 23: Average Sales per transaction over time SMU Classification: Restricted 15 SMU Classification: Restricted 4.2 Household Demographics and their shopping behaviours 4.2.1 Bigger households spend more on average per basket For the next part of the analysis, we joined the transaction dataset with the demographic dataset to explore any insights between shopping behaviours and household demographics. We noticed that bigger households spend more on average per basket. Households with 4 and 5+ people have an average basket spending of $46.1 and $45.4 respectively compared to $32.2 for households with 1 person. Figure 24: Boxplot of Household size and Average Sales 4.2.2 Middle aged households spend more on average per basket Households between age of 25 to 44 spend more on average compared to the other households. Households between 35-44 have the highest average basket spending at $41.6 while households between 19-24 have the lowest at $29. Figure 25: Boxplot of Household Age Group and Average Sales SMU Classification: Restricted 16 SMU Classification: Restricted 4.2.3 Higher income households spend more on average per basket The average basket spending for households seemed to increase with household income, with households earning <24K having an average spending of $29.1 compared to households earning >150K spending $54.6. Figure 26: Boxplot of Household Income and Average Sales 4.2.3.1 Does average spending differ for different household income? (Hypothesis Testing) We are interested to test if households with different income have different average spending. To determine the appropriate hypothesis testing method, we will check if the distributions of the average spending in various income groups are normal. We used the Shapiro-Wilk Goodness-of-Fit Test with confidence level of 95% to test the null hypothesis that average spending is normally distributed. Figure 27: Normal Distribution Test on Average Sales for household income below 24K SMU Classification: Restricted 17 SMU Classification: Restricted Figure 28: Normal Distribution Test on Average Sales for household income between 25K to 49K Figure 29: Normal Distribution Test on Average Sales for household income between 50K to 99K Figure 30: Normal Distribution Test on Average Sales for household income between 100K to 149K SMU Classification: Restricted 18 SMU Classification: Restricted Figure 31: Normal Distribution Test on Average Sales for household income above 150K Based on the results above, we reject that distribution is normal for the first 4 income groups as p-values < critical value 0.05, while we will accept that average spending for households earning >150K is normally distributed as p-value 0.0777 > critical value 0.05. Given that at least one of the distributions is not normally distributed, we will conduct our hypothesis testing using non-parametric Kruskal-Wallis test with 95% confidence level. Our hypothesis is defined as: • H0 = There is no difference between average basket spending and household income • H1 = There is difference between average basket spending and household income Figure 32: Kruskal-Wallis Test for Average Sales vs Household Income The result has a p-value smaller than 0.0001 < critical value 0.05. Hence, we have statistical evidence to reject the null hypothesis and conclude that average basket spending differs between households with different income. Commented [KTS4]: The hypothesis should be “There is no different between the average basket spending by household income groups”. Note, I am not correcting your English. I am mainly focus on the meaning of the statement. Commented [KTS5]: In statistics, the appropriate term is “infer”. SMU Classification: Restricted 19 SMU Classification: Restricted 4.2.4 Elderly shop at the supermarket earlier and are more flexible with their timing Households in age group 65+ have average median transaction time at 1464 which is earlier than other age groups. They also have a wider range of transaction timing, indicating that they are more flexible. Households in the age group of 19-24 have the latest average median transaction time at 1700 and also seemed to be the least flexible. Figure 33: Boxplot of Household Age group and Median Transaction Time 4.3 Marketing Campaigns and their impact on shopping behaviours 4.3.1 Type A campaigns have higher coupon redemption rate Based on the data description, Type A coupons are sent based on customers’ prior purchase behaviour while the other 2 types of campaigns sent all coupons to participating customers. From Campaign_Consolidated dataset, we observed that Type A campaigns have higher coupon redemption rate at 16% compared to the other 2 campaign types. Figure 34: Mosaic Plot of Campaign Types and Coupon Redemption Rate SMU Classification: Restricted 20 SMU Classification: Restricted 4.3.1.1 Does coupon redemption rate differ for campaign types? (Hypothesis testing) Given that both variables are categorical, we test the hypothesis using Chi-Square with confidence level of 95%. We defined our hypothesis as: • H0 = Coupon redemption is independent of campaign types • H1 = Coupon redemption is not independent of campaign types All the counts within our contingency table are >5 and the test result for Likelihood Ratio have p-value < critical value 0.05, so we will reject our null hypothesis and conclude that coupon redemption is not independent of campaign types. Figure 35: Contingency table and Chi-square test of campaign types and coupon redemption rate 4.3.2 Product transactions with coupon discount have higher Sales Value When we looked at the product transactions data, only slightly more than 1% of the transactions have coupon discounts (Figure 36). While product transactions have increased over time, transactions with coupon discounts did not increase at the same pace (Figure 37). Despite this, product transactions with coupon discounts have higher average sales value than transactions without coupon discounts (Figure 38). SMU Classification: Restricted 21 SMU Classification: Restricted Figure 36: Transactions with coupon discount Figure 37: Transactions with coupon discount over time Figure 38: Average sales value between Transactions with or without coupon discounts Commented [KTS6]: Avoid using bar chart when showing mean. Boxplot is more appropriate. SMU Classification: Restricted 22 SMU Classification: Restricted 4.3.3 Households that have loyalty card discount spends more in a basket Based on our observations, approximately half of the product transactions have loyalty card discounts but more than 80% of basket transactions have at least 1 product with loyalty card discounts (figure 39 and 40). The average sales value is similar between product transactions with or without loyalty card discounts (figure 41). However interestingly, basket transactions with at least 1 product enjoying loyalty card discounts have higher average sales value at $33.7(figure 42). We also observed a possible linear relationship between loyalty card discount given and total sales value of a basket (figure 43). Figure 39: Loyalty Discount on product transactions Figure 40: Loyalty Discount on Basket transactions SMU Classification: Restricted 23 SMU Classification: Restricted Figure 41: Average Sales Value of products with or without loyalty card discounts Figure 42: Average Sales Value of baskets with or without loyalty card discounts Figure 43: Scatterplot of Basket Sales Value and Loyalty Card Discounts SMU Classification: Restricted 24 SMU Classification: Restricted 4.3.3.1 Is sales value of basket correlated to loyalty card discount? (Hypothesis testing) We want to test if the linear relationship between loyalty card discount and sales value of a basket is statistically significant. We will conduct a correlation analysis between these 2 continuous variables using 95% confidence level, and define our hypothesis as: • H0 = R is equal to 0 • H1 = R is not equal to 0 Based on our test results, the 2 variables have a correlation of -0.78498 with p-value < 0.0001. Therefore, we will reject our null hypothesis and conclude that the sales value of a basket is negatively correlated to the loyalty card discount given. Since loyalty card discounts were recorded as negative values, we can then conclude that the more loyalty card discounts that customers received, the more they will spend in that basket. Figure 44: Correlation Analysis of Basket Sales Value and Loyalty Card Discount SMU Classification: Restricted 25 SMU Classification: Restricted 5 INTERPRETATION OF RESULTS Customers have a preference on when to buy groceries The stores are busiest between 3pm to 8pm as well as day 4 and 5 of the week. Elderly households are more flexible on the time for grocery shopping and on average transacted earlier at 1464. Customers return to the supermarket chain to buy their groceries 90% of households have been customers for more than 567 days. 75% made a purchase in the last 20 days and 50% transacted at least 74 times in 711 days. Average sales per basket have remained largely the same Although total sales have improved over time, this is attributed to an increase in transaction volume as average basket sales remain around $29. Demographics affect the spending behaviour of these customers Households with 4 and 5+ people have an average basket spending of $46.1 and $45.4 respectively compared to $32.2 for households with only 1 person. Households between age of 35-44 have highest average basket spending at $41.6 while households between age of 19- 24 have lowest at $29. Average basket spending increases with household income, with households earning <24K spending $29.1 on average compared to households earning >150K spending $54.6. Targeted campaigns have better results Type A campaigns which sent coupons based on prior purchasing behaviour have higher coupon redemption rate at 16% compared to the other 2 types at 7.9% and 7.7%. Coupon discounts have an impact on sales value Product transactions with coupon discounts have average sales value of $4.04 compared to $2.88 for transactions without coupon discounts. Loyalty card discounts influence the customers behaviour 80% of basket transactions received loyalty card discounts, and these transactions have higher average sales value of $33.7 compared to $8.61 for baskets without loyalty card discounts. Sales value of a basket is also inversely correlated to the amount of loyalty card discounts received. Commented [KTS7]: Well summarised. Can be improved by referencing to the section, sub-section or/and figure. Please do remember to include recommendation to address data issues and direction for further investigation. SMU Classification: Restricted 26 SMU Classification: Restricted Appendix A – Dataset Used S/N Filename Brief Description Usage 1 hh_demographic Demographic data on 801 households. Contains 8 variables and the key variables used are household_key, AGE_DESC, INCOME_DESC and HOUSEHOLD_SIZE_DESC. Joined with transaction data for further analysis on shopping behaviours 2 transaction_data Transaction data over 2 years on 2500 households with 12 variables. Primary dataset for analysis and is joined with other data for further analysis 3 campaign_table List the campaign received by all the household in this study. Contains 3 variables. Joined with other campaign and coupon dataset to analysis the coupon redemption rate of these campaign 4 campaign_desc Provides the length of time that each campaign runs. Contains 4 variables Joined with other campaign and coupon dataset to analysis the coupon redemption rate of these campaign 5 product Information on all the products that are sold by the supermarket chains. Contains 7 variables. Product behaviours are outside the scope of this study and hence information was used for reference purposes only. 6 coupon List all the coupons available for a campaign and the respective products. Contains 3 variables. Joined with other campaign and coupon dataset to analysis the coupon redemption rate of these campaign 7 coupon_redempt List all the coupons redeemed by household during the study period. Contains 4 variables Joined with other campaign and coupon dataset to analysis the coupon redemption rate of these campaign 8 causal_data Identifies whether a product was featured in a mailer or was part of an in-store display. Contains 5 variables. Mailer and Display behaviours are outside the scope of this study and hence information was used for reference purposes only. SMU Classification: Restricted 27 SMU Classification: Restricted 9 Campaign_Consolidated Created by Joining campaign_table and campaign_desc by matching CAMPAIGN column; script name: Join campaign_table and campaign_DESC Identifies whether a campaign has any coupon redemption. 10 Consolidated transaction_data by Basket_ID Created by Summary of transaction_data grouped by BASKET_ID; script name: Summary by Basket ID Groups all the transactions by Basket to analyse shopping behaviours from a Basket transaction perspective. 11 Consolidated transaction_data By household_key Created by Summary of Consolidated transaction_data by Basket_ID grouped by household_key; script name: Summary by household Groups all the transactions by household to analyse shopping behaviours from a household perspective. 12 Joined Data of Demographics and Transactions Created by Joining hh_demographic and Consolidated transaction_data By household_key by matching household_key column; script name: Join with Transactions Used to analyse household demographics and their shopping behaviours SMU Classification: Restricted 28 SMU Classification: Restricted Appendix B – Preparation Log of Original Data S/N DataSet Variable Name Comments Action 1 hh_demographic AGE_DESC - - 2 hh_demographic MARITAL_STATUS_CODE Categorical variable with 3 levels; 344 records with unknown value (42.9%) Excluded due to large number of records with unknown value 3 hh_demographic INCOME_DESC Categorical variable with 12 levels; Recoded to 5 levels for analysis needs in hypothesis: Added new Column INCOME_DESC_Recoded Hidden and excluded 4 hh_demographic HOMEOWNER_DESC Categorical variable with 5 levels; 233 records with unknown value (29.1%) Distribution heavily skewed to homeowners (504 records) for records with known value (568 records) Hidden and excluded due to very skewed distribution and large number of records with unknown value 5 hh_demographic HH_COMP_DESC Categorical variable with 6 levels; 73 records with unknown value (9.1%) Some data is inconsistent with MARITAL_STATUS_CODE and HOUSEHOLD_SIZE_DESC Hidden and excluded due to inconsistencies; Similar info can be represented by HOUSEHOLD_SIZE_DESC 6 hh_demographic HOUSEHOLD_SIZE_DESC - - 7 hh_demographic KID_CATEGORY_DESC Categorical variable with 4 levels; 558 records with none/unknown value (69.7%) Hidden and excluded due to large number of records with unknown value Similar info can be represented by HOUSEHOLD_SIZE_DESC 8 hh_demographic household_key Wrong modelling type Changed modelling type from continuous to nominal 9 transaction_data household_key Wrong modelling type Changed modelling type from continuous to nominal 10 transaction_data BASKET_ID Wrong modelling type Changed modelling type from continuous to nominal 11 transaction_data DAY Wrong modelling type Changed modelling type from continuous to ordinal SMU Classification: Restricted 29 SMU Classification: Restricted DAY 278 and 643 has very low values compared to the others, 6 and 2 respectively No mentioning of starting day or date hence unable to determine weekdays and weekends Created a new column Day_of_Week by using Modulo(DAY,7) (modelling type Ordinal) 12 transaction_data PRODUCT_ID Wrong modelling type Changed modelling type from continuous to nominal 13 transaction_data Quantity 14,446 rows with 0 Quantity 23,136 rows with outlier value where value is 100 or higher Hide and Exclude rows with 0 Quantity Further investigation on the outliers shows that most of these belong to certain products under Gasoline, and it can be suspected to be a different mode of measurement for quantity for example ml or litres; 99% of the rows have quantity below 100, so we will hide and exclude all rows with values greater or equal to 100; Created a new Column Item_Unit_Price by taking SALES_VALUE divided by Quantity; 14 transaction_data Item_Unit_Price Extreme outliers with 42070 rows with unit price below 0.01 Extreme outliers with 565 rows with unit price greater than 40 Based on our understanding of supermarket, we would expect unit price of items to be above 0.01, so we would be hiding and excluding all rows with value below 0.01 99.5% of the distribution is lesser than 16, but we know that it is possible for supermarket to have items that sells at around $16 per unit so we will be hiding and excluding these rows with value greater than 40 instead 15 transaction_data SALES_VALUE 18879 rows with value lesser than 0.01; 1779 with extreme outlier values of more than or equal to 50 These values do not reflect the actual price that the customer paid These rows with value lesser than 0.01 have been hidden and excluded by using a new column Item_Unit_Price with values lesser than 0.01; 99.5% of the distribution is lesser than 29 so we will be hiding and excluding these rows with values greater than 50 Created a new column Customer_Paid by taking SALES_VALUE + COUPON_DISC, (COUPON DISC is already a negative value) 16 transaction_data Customer_Paid 5819 rows with negative value Negative value means client get paid for buying the item so we will be hiding and excluding all rows with negative value SMU Classification: Restricted 30 SMU Classification: Restricted 17 transaction_data STORE_ID Wrong modelling type Changed modelling type from continuous to nominal 18 transaction_data RETAIL_DISC 36 rows with values above 0 Discount values should be negative, so all rows with value higher than 0 are hidden and excluded 19 transaction_data Have_RETAIL_DISC Recoded from RETAIL_DISC with 0 value as No, and non-zero values as Yes Modelling type: nominal Data type: Character 20 transaction_data TRANS_TIME - - 21 transaction_data WEEK_NO Wrong modelling type Changed modelling type from continuous to ordinal 22 transaction_data COUPON_DISC - - 23 transaction_data Have_COUPON_DISC Recoded from COUPON_DISC with 0 value as No, and non-zero values as Yes Modelling type: nominal Data type: Character 24 transaction_data COUPON_MATCH_DISC 23 rows where Coupon_Match_Disc is more than Coupon_Disc Retailer should only be matching up to what the manufacturing coupon discount is so we will hide and exclude these 23 rows 25 campaign_table DESCRIPTION - - 26 campaign_table household_key Wrong modelling type Changed modelling type from continuous to nominal 27 campaign_table CAMPAIGN Wrong modelling type Changed modelling type from continuous to nominal 28 campaign_desc DESCRIPTION - - 29 campaign_desc CAMPAIGN Wrong modelling type Changed modelling type from continuous to nominal 30 campaign_desc START_DAY Wrong modelling type Changed modelling type from continuous to ordinal 31 campaign_desc END_DAY Wrong modelling type Changed modelling type from continuous to ordinal 32 product PRODUCT_ID Wrong modelling type Changed modelling type from continuous to nominal 33 product MANUFACTURER Wrong modelling type Changed modelling type from continuous to nominal 34 product DEPARTMENT Categorical variable with 43 levels 15 rows with missing values (0.02%) Retained for reference purpose only. Information not used in study purpose 35 product BRAND - - 36 product COMMODITY_DESC Categorical variable with 307 levels 15 rows with missing values (0.02%) Retained for reference purpose only. Information not used in study purpose SMU Classification: Restricted 31 SMU Classification: Restricted 37 product SUB_COMMODITY_DESC Categorical variable with 2382 levels 15 rows with missing values (0.02%) Retained for reference purpose only. Information not used in study purpose 38 product CURR_SIZE_OF_PRODUCT Categorical variable with 4344 levels 30607 rows with missing values (33.1%) Hidden and excluded due to large number of missing data and info is not relevant to study purpose 39 coupon COUPON_UPC Wrong modelling type Changed modelling type from continuous to nominal 40 coupon PRODUCT_ID Wrong modelling type Changed modelling type from continuous to nominal 41 coupon CAMPAIGN Wrong modelling type Changed modelling type from continuous to nominal 42 coupon_redempt household_key Wrong modelling type Changed modelling type from continuous to nominal 43 coupon_redempt DAY Wrong modelling type Changed modelling type from continuous to ordinal 44 coupon_redempt COUPON_UPC Wrong modelling type; Data description states that this value should be unique to each household and campaign, however it is found that different household under the same campaign can have the same COUPON_UPC value and different campaign can also have the same COUPON_UPC Changed modelling type from continuous to nominal Retained as this is the best available data to identify coupon redemption 45 coupon_redempt CAMPAIGN Wrong modelling type Changed modelling type from continuous to nominal 46 casual_data PRODUCT_ID Wrong modelling type Changed modelling type from continuous to nominal 47 casual_data STORE_ID Wrong modelling type Changed modelling type from continuous to nominal 48 casual_data WEEK_NO Wrong modelling type Changed modelling type from continuous to ordinal 49 casual_data display Categorical data with 10 levels; Distribution frequency is low in categories which are not 0 Recoded to 2 levels for potential analysis needs: Added new column display_Recoded 50 casual_data mailer Categorical data with 11 levels; Distribution frequency is concentrated in category 0 and A Recoded to 2 levels for potential analysis needs: Added new column mailer_Recoded SMU Classification: Restricted 32 SMU Classification: Restricted Appendix C – Preparation Log of New Tables DataSet Variable Name Modelling Type Comments Campaign_Consolidated DESCRIPTION Nominal Source: campaign_table Campaign_Consolidated household_key Nominal Source: campaign_table Campaign_Consolidated CAMPAIGN Nominal Source: campaign_table and campaign_desc Campaign_Consolidated START_DAY Ordinal Source: campaign_desc Campaign_Consolidated END_DAY Ordinal Source: campaign_desc Campaign_Consolidated Any_Redemptions Nominal Created by Updating Campaign_Consolidated with coupon_redempt by matching household_key and campaign; only update DAY column from coupon_redempt to identify if there was any redemption; Recoded to No for missing values and Yes for all present values Consolidated transaction_data by Basket_ID BASKET_ID Nominal Source: transaction_data Consolidated transaction_data by Basket_ID household_key Nominal Source: transaction_data Consolidated transaction_data by Basket_ID DAY Ordinal Source: transaction_data Consolidated transaction_data by Basket_ID Day_of_Week Ordinal Source: transaction_data Consolidated transaction_data by Basket_ID WEEK_NO Ordinal Source: transaction_data Consolidated transaction_data by Basket_ID TRANS_TIME Continuous Source: transaction_data SMU Classification: Restricted 33 SMU Classification: Restricted Consolidated transaction_data by Basket_ID Num_of_Products Continuous Source: transaction_data; number of products transacted in 1 basket Consolidated transaction_data by Basket_ID SALES_Value_of_Basket Continuous Source: transaction_data; Sum of SALES_VALUE of all products in 1 basket Consolidated transaction_data by Basket_ID Retail_DISC_of_Basket Continuous Source: transaction_data; Sum of RETAIL_DISC of all products in 1 basket Consolidated transaction_data by Basket_ID Have_Retail_DISC Nominal Recoded from Retail_DISC_of_Basket with 0 value as No, and non-zero values as Yes Consolidated transaction_data By household_key household_key nominal Source: Consolidated transaction_data by Basket_ID Consolidated transaction_data By household_key Number_of_Transactions Continuous Source: Consolidated transaction_data by Basket_ID; number of basket transactions Consolidated transaction_data By household_key First_Day_of_Transaction Continuous Source: Consolidated transaction_data by Basket_ID; MIN value of DAY Consolidated transaction_data By household_key Last_Day_of_Transaction Continuous Source: Consolidated transaction_data by Basket_ID; MAX value of DAY Consolidated transaction_data By household_key Days_from_last_visit Continuous Formula: 711 - Last_Day_of_Transaction Consolidated transaction_data By household_key Duration_as_Customer Continuous Source: Consolidated transaction_data by Basket_ID: Range Value of Day Consolidated transaction_data By household_key Median_Day_of_Week Ordinal Source: Consolidated transaction_data by Basket_ID; Median value of Day_of_Week SMU Classification: Restricted 34 SMU Classification: Restricted Consolidated transaction_data By household_key Median_Time Continuous Source: Consolidated transaction_data by Basket_ID; Median value of TRANS_TIME Consolidated transaction_data By household_key Average_Sales_per_basket Continuous Source: Consolidated transaction_data by Basket_ID; Mean value of SALES_Value_of_Basket Joined Data of Demographics and Transactions household_key Nominal Source: hh_demographic and Consolidated transaction_data By household_key Joined Data of Demographics and Transactions AGE_DESC Nominal Source: hh_demographic Joined Data of Demographics and Transactions INCOME_DESC_Recoded Nominal Source: hh_demographic Joined Data of Demographics and Transactions HOUSEHOLD_SIZE_DESC Nominal Source: hh_demographic Joined Data of Demographics and Transactions Number_of_Transactions Continuous Source: Consolidated transaction_data By household_key Joined Data of Demographics and Transactions First_Day_of_Transaction Continuous Source: Consolidated transaction_data By household_key Joined Data of Demographics and Transactions Days_from_last_visit Continuous Source: Consolidated transaction_data By household_key Joined Data of Demographics and Transactions Duration_as_Customer Continuous Source: Consolidated transaction_data By household_key Joined Data of Demographics and Transactions Median_Day_of_Week Ordinal Source: Consolidated transaction_data By household_key SMU Classification: Restricted 35 SMU Classification: Restricted Joined Data of Demographics and Transactions Median_Time Continuous Source: Consolidated transaction_data By household_key Joined Data of Demographics and Transactions Average_Sales_per_basket Continuous Source: Consolidated transaction_data By household_key