xuebaunion@vip.163.com
3551 Trousdale Rkwy, University Park, Los Angeles, CA
留学生论文指导和课程辅导
无忧GPA:https://www.essaygpa.com
工作时间:全年无休-早上8点到凌晨3点
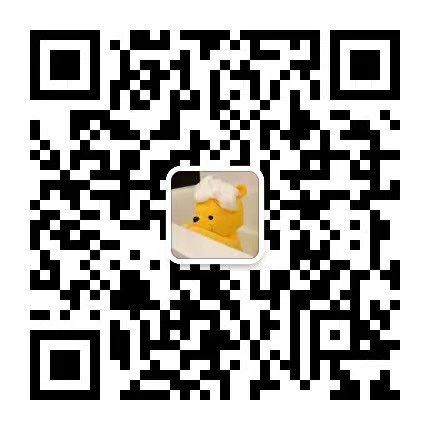
微信客服:xiaoxionga100
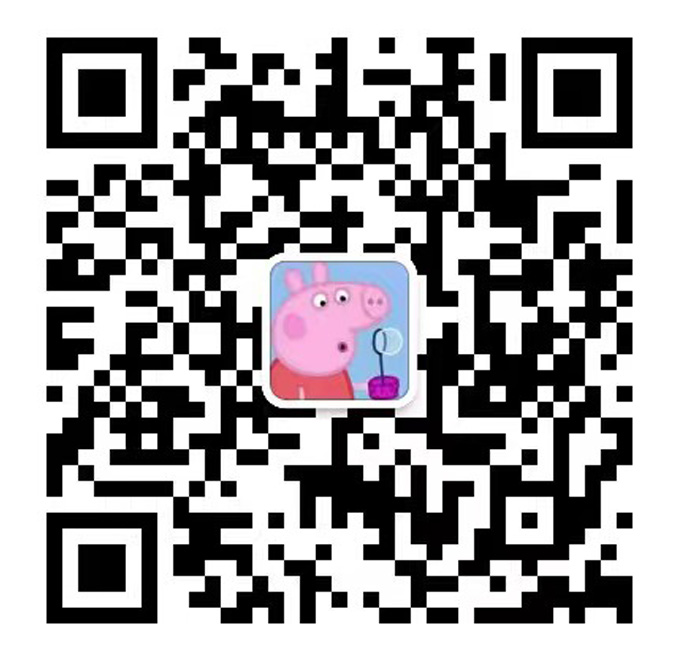
微信客服:ITCS521
1 CHAPTER 1: CLAIM TRIANGLES In many types of insurance, there are IBNR (Incurred but not reported) claims. It could be that claims take months or even years to be reported and then paid. Insurance companies need a good way to determine the “ultimate” number of claims given the information they have at any given moment. For instance, imagine the following table represents claims from a particular set of policies by “policy year” and “development year”. I’ve simulated these values so I know the true number of ultimate claims in each year. Development Year Year 0 1 2 3 4 5 6 7 8 9 10 2008 3031 1108 409 153 47 17 6 5 2 1 0 2009 2871 1117 401 159 65 22 6 3 1 1 2010 2962 1080 407 169 57 26 6 3 0 2011 3411 1262 450 160 65 12 9 4 2012 3239 1191 452 152 65 26 9 2013 3166 1185 425 150 64 16 2014 3295 1265 453 191 70 2015 3122 1119 443 160 2016 3148 1212 403 2017 3139 1121 2018 3301 The basic method works like this: The first step is to create the cumulative claims table: Development Year Year 0 1 2 3 4 5 6 7 8 9 10 2008 3031 4139 4548 4701 4748 4765 4771 4776 4778 4779 4779 2009 2871 3988 4389 4548 4613 4635 4641 4644 4645 4646 2010 2962 4042 4449 4618 4675 4701 4707 4710 4710 2011 3411 4673 5123 5283 5348 5360 5369 5373 2012 3239 4430 4882 5034 5099 5125 5134 2013 3166 4351 4776 4926 4990 5006 2014 3295 4560 5013 5204 5274 2015 3122 4241 4684 4844 2016 3148 4360 4763 2017 3139 4260 2018 3301 2 Every development year has a development factor computed for that year. To compute that development factor, you take all calendar years in which the ending cumulative claims are known. You take the ending cumulative claims and divide by the beginning cumulative claims to get that year’s development factor. Year-by-year, these factors are: 0|1 4139 3988 4042 4673 4430 4351 4560 4241 4360 4260 3031 2871 2962 3411 3239 3166 3295 3122 314 1.3715268 3 8 3139 9d 1|2 4548 4389 4449 5123 4882 4776 5013 4684 4763 4139 3988 4042 4673 4430 4351 4560 4241 1.09908725 4 2 360 d 2|3 4701 4548 4618 5283 5034 4926 5204 4844 4548 4389 4449 5123 4882 4776 5013 1.03417494 4684 2d 3|4 4748 4613 4675 5348 5099 4990 5274 4701 4548 4618 5283 5034 4926 520 1.0126 5 4 187 6d 4|5 4765 4635 4701 5360 5125 5006 4748 4613 4675 5348 1.004037594 5099 4990 d 5|6 4771 4641 4707 5369 5134 4765 4635 4701 5360 512 1.00146424 5 8d 6|7 4776 4644 4710 5373 4771 4641 4707 5369 1.000769704d 7|8 4778 4645 4710 4776 4644 4710 1.000212314d 8|9 4779 4646 4778 1.000212247 4645 d 9|10 4779 4779 1.000000000d We can then use these factors to determine the ultimate claims for each year. For instance the cumulative claims for 2018 would be filled in as follows: Year 1 3301 1.371526893 4527.410273 Year 2 4527.410273 1.099087252 4976.018918 And so on giving ultimate claims of 5245.97165 Similarly, for 2017, the Year 2 claims are 4260 1.099087252 4682.111696 and ultimate claims are 4936.119742 . 3 The fully developed table is (values rounded to two decimal places): Development Year Year 0 1 2 3 4 5 6 7 8 9 10 2008 3031 4139 4548 4701 4748 4765 4771 4776 4778 4779 4779 2009 2871 3988 4389 4548 4613 4635 4641 4644 4645 4646 4646.00 2010 2962 4042 4449 4618 4675 4701 4707 4710 4710 4711.00 4711.00 2011 3411 4673 5123 5283 5348 5360 5369 5373 5374.14 5375.28 5375.28 2012 3239 4430 4882 5034 5099 5125 5134 5137.95 5139.04 5140.13 5140.13 2013 3166 4351 4776 4926 4990 5006 5013.33 5017.19 5018.25 5019.32 5019.32 2014 3295 4560 5013 5204 5274 5295.29 5303.05 5307.13 5308.26 5309.38 5309.38 2015 3122 4241 4684 4844 4905.13 4924.93 4932.14 4935.94 4936.99 4938.03 4938.03 2016 3148 4360 4763 4925.78 4987.93 5008.07 5015.40 5019.27 5020.33 5021.40 5021.40 2017 3139 4260 4682.11 4842.12 4903.22 4923.02 4930.23 4934.02 4935.07 4936.12 4936.12 2018 3301 4527.41 4976.02 5146.07 5211.01 5232.05 5239.71 5243.75 5244.86 5245.97 5245.97 The final ultimate claims is the sum of the last column: 4779 4646.00 4711.00 5375.28 5140.13 5019.32 5309.38 4938.03 5021.40 4936.12 5245.97 55,121.64 The current claims is the sum of the diagonal: 4779 4646 4710 5373 5134 5006 5274 4844 4763 4260 3301 52,090 Therefore, the company needs to hold an IBNR reserve for the difference of $3,031.64. It turns out in my simulation that the actual future claims will be $3,022 and the company will be slightly over-reserved. How do we “weight” experience in different years? In the method above we weighted by number of claims, but we could weight equally by year, so that we just take the arithmetic average. That is: 0|1 1 4139 3988 4042 4673 4430 4351 4560 4241 4360 4260 10 3031 2871 2962 3411 3239 3166 3295 3122 3 1.371567792 148 3139 d 1|2 1 4548 4389 4449 5123 4882 4776 5013 4684 4763 9 4139 3988 4042 4673 4430 4351 4560 4241 436 1.09914427 0 7d 2|3 1 4701 4548 4618 5283 5034 4926 5204 4844 8 4548 4389 4449 5123 4882 4776 5013 46 1.0342 84 35931d 4 3|4 1 4748 4613 4675 5348 5099 4990 5274 7 4701 4548 4618 5283 5034 49 1. 26 520 0126 16 4 13 6d 4|5 1 4765 4635 4701 5360 5125 5006 6 4748 4613 4675 53 1.004076 48 5099 4990 727d 5|6 1 4771 4641 4707 5369 5134 5 4765 4635 1.0014530 4701 5360 5125 41d 6|7 1 4776 4644 4710 5373 4 4771 4641 4707 536 1.0007691 4 9 9d 7|8 1 4778 4645 4710 3 4776 4644 4710 1.000211364d 8|9 1 4779 4646 2 4778 46 1.0002122 5 8 4 9d 9|10 4779 4779 1.000000000d And the resulting table looks like this: Development Year Year 0 1 2 3 4 5 6 7 8 9 10 2008 3031 4139 4548 4701 4748 4765 4771 4776 4778 4779 4779 2009 2871 3988 4389 4548 4613 4635 4641 4644 4645 4646 4646.00 2010 2962 4042 4449 4618 4675 4701 4707 4710 4710 4711.00 4711.00 2011 3411 4673 5123 5283 5348 5360 5369 5373 5374.14 5375.28 5375.28 2012 3239 4430 4882 5034 5099 5125 5134 5137.95 5139.04 5140.13 5140.13 2013 3166 4351 4776 4926 4990 5006 5013.27 5017.13 5018.19 5019.26 5019.26 2014 3295 4560 5013 5204 5274 5295.50 5303.20 5307.27 5308.40 5309.52 5309.52 2015 3122 4241 4684 4844 4905.10 4925.09 4932.25 4936.05 4937.09 4938.14 4938.14 2016 3148 4360 4763 4926.07 4988.20 5008.53 5015.81 5019.67 5020.73 5021.80 5021.80 2017 3139 4260 4682.35 4842.66 4903.74 4923.73 4930.89 4934.68 4935.72 4936.77 4936.77 2018 3301 4527.55 4976.43 5146.80 5211.72 5232.96 5240.57 5244.60 5245.71 5246.82 5246.82 The final ultimate claims is the sum of the last column: 4779 4646.00 4711.00 5375.28 5140.13 5019.26 5309.52 4938.14 5021.80 4936.77 5246.82 55,123.70 The current claims is the sum of the diagonal: 4779 4646 4710 5373 5134 5006 5274 4844 5 4763 4260 3301 52,090 Therefore, the company needs to hold an IBNR reserve for the difference of $3,033.70. In practice, the reserves will not usually be this close together. Taking Inflation into account The inflation rates for the years 2008 to 2018 in the US were: 2008 2009 2010 2011 2012 2013 2014 2015 2016 2017 2018 3.84% -0.36% 1.64% 3.16% 2.07% 1.46% 1.62% 0.12% 1.26% 2.13% 2.53% These rates can be used to adjust the claims in the initial table (claims per year, not cumulative claims per year). Three quick examples: The 2008 inflation adjusted claims in development year 0 would be: 3031 1 0.0384 1 0.0036 1 0.0164 1 0.0316 1 0.0207 1 0.0146 1 0.0162 1 0.0012 1 0.0126 1 0.0213 3582.98 The 2008 inflation adjusted claims in development year 1 would be: 1108 1 0.0036 1 0.0164 1 0.0316 1 0.0207 1 0.0146 1 0.0162 1 0.0012 1 0.0126 1 0.0213 1261.34 The 2009 inflation adjusted claims in development year 0 would be: 2871 1 0.0036 1 0.0164 1 0.0316 1 0.0207 1 0.0146 1 0.0162 1 0.0012 1 0.0126 1 0.0213 3268.34 And so on… The claim table then looks like this… 6 Development Year Year 0 1 2 3 4 5 6 7 8 9 10 2008 3582.98 1261.34 467.29 171.98 51.21 18.15 6.31 5.18 2.07 1.02 0.00 2009 3268.34 1276.18 450.75 173.25 69.39 23.15 6.21 3.10 1.02 1.00 2010 3384.12 1214.00 443.48 180.42 59.97 26.92 6.21 3.06 0.00 2011 3834.22 1375.13 480.40 168.35 67.30 12.41 9.19 4.00 2012 3529.35 1271.45 475.59 157.38 67.22 26.55 9.00 2013 3379.85 1246.84 440.05 155.13 65.36 16.00 2014 3466.94 1309.79 468.48 195.07 70.00 2015 3232.55 1157.23 452.44 160.00 2016 3255.56 1237.82 403.00 2017 3205.86 1121.00 2018 3301.00 The cumulative claims table looks like this …. Development Year Year 0 1 2 3 4 5 6 7 8 9 10 2008 3582.98 4844.33 5311.61 5483.60 5534.81 5552.96 5559.27 5564.45 5566.52 5567.54 5567.54 2009 3268.34 4544.52 4995.28 5168.53 5237.92 5261.07 5267.28 5270.38 5271.41 5272.41 2010 3384.12 4598.12 5041.60 5222.02 5281.99 5308.91 5315.12 5318.18 5318.18 2011 3834.22 5209.35 5689.75 5858.10 5925.40 5937.81 5947.00 5951.00 2012 3529.35 4800.80 5276.39 5433.77 5500.99 5527.54 5536.54 2013 3379.85 4626.68 5066.73 5221.86 5287.22 5303.22 2014 3466.94 4776.74 5245.21 5440.28 5510.28 2015 3232.55 4389.78 4842.22 5002.22 2016 3255.56 4493.38 4896.38 2017 3205.86 4326.86 2018 3301.00 The development factors are then computed by one of the two methods above. The resulting completed table looks like this: 7 Development Year Year 0 1 2 3 4 5 6 7 8 9 10 2008 3582.98 4844.33 5311.61 5483.60 5534.81 5552.96 5559.27 5564.45 5566.52 5567.54 5567.54 2009 3268.34 4544.52 4995.28 5168.53 5237.92 5261.07 5267.28 5270.38 5271.41 5272.41 5272.41 2010 3384.12 4598.12 5041.60 5222.02 5281.99 5308.91 5315.12 5318.18 5318.18 5319.17 5319.17 2011 3834.22 5209.35 5689.75 5858.10 5925.40 5937.81 5947.00 5951.00 5952.14 5953.25 5953.25 2012 3529.35 4800.80 5276.39 5433.77 5500.99 5527.54 5536.54 5540.39 5541.45 5542.48 5542.48 2013 3379.85 4626.68 5066.73 5221.86 5287.22 5303.22 5310.32 5314.01 5315.02 5316.02 5316.02 2014 3466.94 4776.74 5245.21 5440.28 5510.28 5531.00 5538.40 5542.25 5543.31 5544.34 5544.34 2015 3232.55 4389.78 4842.22 5002.22 5061.79 5080.81 5087.61 5091.15 5092.12 5093.07 5093.07 2016 3255.56 4493.38 4896.38 5057.14 5117.37 5136.60 5143.48 5147.05 5148.03 5148.99 5148.99 2017 3205.86 4326.86 4744.51 4900.30 4958.65 4977.29 4983.95 4987.41 4988.37 4989.30 4989.30 2018 3301.00 4506.81 4941.83 5104.09 5164.87 5184.29 5191.23 5194.83 5195.83 5196.80 5196.80 And the sum of the last column minus the sum of the diagonal elements gives: 58,943.36 55,985.63 2957.73 . At this point, the book suggests that since this reserve is lower than the reserve previously computed, neglecting inflation might cause a company to underreserve. On the other hand, this analysis neglects future inflation. If we assume that future inflation will be 2% per year, it will increase all of the red values in the table above. We first need to deaccumulate the table to get the claims in specific years: Year 0 1 2 3 4 5 6 7 8 9 10 2008 3582.98 1261.34 467.29 171.98 51.21 18.15 6.31 5.18 2.07 1.02 0.00 2009 3268.34 1276.18 450.75 173.25 69.39 23.15 6.21 3.10 1.02 1.00 0.00 2010 3384.12 1214.00 443.48 180.42 59.97 26.92 6.21 3.06 0.00 0.99 0.00 2011 3834.22 1375.13 480.40 168.35 67.30 12.41 9.19 4.00 1.14 1.11 0.00 2012 3529.35 1271.45 475.59 157.38 67.22 26.55 9.00 3.85 1.06 1.03 0.00 2013 3379.85 1246.84 440.05 155.13 65.36 16.00 7.10 3.69 1.02 0.99 0.00 2014 3466.94 1309.79 468.48 195.07 70.00 20.71 7.40 3.85 1.06 1.03 0.00 2015 3232.55 1157.23 452.44 160.00 59.57 19.03 6.80 3.53 0.97 0.95 0.00 2016 3255.56 1237.82 403.00 160.77 60.22 19.24 6.87 3.57 0.98 0.96 0.00 2017 3205.86 1121.00 417.65 155.78 58.35 18.64 6.66 3.46 0.95 0.93 0.00 2018 3301.00 1205.81 435.02 162.26 60.78 19.42 6.94 3.61 0.99 0.97 0.00 Then we need to inflate the yearly projections: Year 0 1 2 3 4 5 6 7 8 9 10 2008 3582.98 1261.34 467.29 171.98 51.21 18.15 6.31 5.18 2.07 1.02 0.00 8 2009 3268.34 1276.18 450.75 173.25 69.39 23.15 6.21 3.10 1.02 1.00 0.00 2010 3384.12 1214.00 443.48 180.42 59.97 26.92 6.21 3.06 0.00 1.01 0.00 2011 3834.22 1375.13 480.40 168.35 67.30 12.41 9.19 4.00 1.16 1.15 0.00 2012 3529.35 1271.45 475.59 157.38 67.22 26.55 9.00 3.92 1.10 1.10 0.00 2013 3379.85 1246.84 440.05 155.13 65.36 16.00 7.24 3.84 1.08 1.07 0.00 2014 3466.94 1309.79 468.48 195.07 70.00 21.13 7.70 4.08 1.15 1.14 0.00 2015 3232.55 1157.23 452.44 160.00 60.76 19.80 7.22 3.83 1.08 1.07 0.00 2016 3255.56 1237.82 403.00 163.98 62.65 20.41 7.44 3.94 1.11 1.10 0.00 2017 3205.86 1121.00 426.01 162.07 61.93 20.18 7.35 3.90 1.10 1.09 0.00 2018 3301.00 1229.93 452.60 172.19 65.79 21.44 7.81 4.14 1.16 1.16 0.00 And then reaccumulate: Year 0 1 2 3 4 5 6 7 8 9 10 2008 3582.98 4844.33 5311.61 5483.60 5534.81 5552.96 5559.27 5564.45 5566.52 5567.54 5567.54 2009 3268.34 4544.52 4995.28 5168.53 5237.92 5261.07 5267.28 5270.38 5271.41 5272.41 5272.41 2010 3384.12 4598.12 5041.60 5222.02 5281.99 5308.91 5315.12 5318.18 5318.18 5319.19 5319.19 2011 3834.22 5209.35 5689.75 5858.10 5925.40 5937.81 5947.00 5951.00 5952.16 5953.32 5953.32 2012 3529.35 4800.80 5276.39 5433.77 5500.99 5527.54 5536.54 5540.47 5541.57 5542.67 5542.67 2013 3379.85 4626.68 5066.73 5221.86 5287.22 5303.22 5310.46 5314.30 5315.38 5316.45 5316.45 2014 3466.94 4776.74 5245.21 5440.28 5510.28 5531.41 5539.11 5543.19 5544.34 5545.48 5545.48 2015 3232.55 4389.78 4842.22 5002.22 5062.98 5082.77 5089.99 5093.82 5094.89 5095.96 5095.96 2016 3255.56 4493.38 4896.38 5060.36 5123.01 5143.43 5150.87 5154.81 5155.92 5157.03 5157.03 2017 3205.86 4326.86 4752.87 4914.94 4976.87 4997.04 5004.40 5008.30 5009.39 5010.48 5010.48 2018 3301.00 4530.93 4983.52 5155.71 5221.51 5242.94 5250.76 5254.90 5256.06 5257.22 5257.22 And the sum of the last column minus the sum of the diagonal elements gives: 59,037.75 55,985.63 3052.11 , which is back to the normal range. Average Cost per Claim Method To implement this method, we need to know both the number and the average size of claims. Assume the table we’ve been using is for “Number of Claims”. We complete it as we have been doing. Assume the total dollar amount of claims are as in this table: Claims Development Year Year 0 1 2 3 4 5 6 7 8 9 10 9 2008 $4,407,650 $1,658,410 $579,745 $187,091 $55,899 $17,053 $5,469 $3,271 $1,428 $719 $0 2009 $4,506,494 $1,789,747 $564,700 $205,554 $82,425 $22,025 $6,936 $2,718 $867 $601 2010 $4,738,519 $1,634,353 $641,033 $216,606 $65,814 $30,819 $6,718 $2,617 $0 2011 $5,613,005 $1,888,078 $635,756 $179,344 $88,885 $13,504 $11,011 $2,839 2012 $4,910,583 $1,836,558 $669,448 $185,873 $89,627 $29,472 $9,903 2013 $5,102,072 $1,814,010 $598,353 $214,152 $85,185 $15,528 2014 $4,786,811 $1,840,727 $626,993 $253,640 $78,915 2015 $4,808,567 $1,813,809 $582,155 $192,541 2016 $5,418,338 $1,945,527 $505,725 2017 $5,274,933 $1,722,629 2018 $5,385,714 10 The Cumulative dollar amounts are then: Cumulative Development Year Year 0 1 2 3 4 5 2008 $4,407,650 $6,066,060 $6,645,805 $6,832,896 $6,888,795 $6,905,848 2009 $4,506,494 $6,296,241 $6,860,941 $7,066,495 $7,148,920 $7,170,945 2010 $4,738,519 $6,372,872 $7,013,905 $7,230,511 $7,296,325 $7,327,144 2011 $5,613,005 $7,501,083 $8,136,839 $8,316,183 $8,405,068 $8,418,572 2012 $4,910,583 $6,747,141 $7,416,589 $7,602,462 $7,692,089 $7,721,561 2013 $5,102,072 $6,916,082 $7,514,435 $7,728,587 $7,813,772 $7,829,300 2014 $4,786,811 $6,627,538 $7,254,531 $7,508,171 $7,587,086 2015 $4,808,567 $6,622,376 $7,204,531 $7,397,072 2016 $5,418,338 $7,363,865 $7,869,590 2017 $5,274,933 $6,997,562 2018 $5,385,714 6 7 8 9 10 $6,911,317 $6,914,588 $6,916,016 $6,916,735 $6,916,735 $7,177,881 $7,180,599 $7,181,466 $7,182,067 $7,333,862 $7,336,479 $7,336,479 $8,429,583 $8,432,422 $7,731,464 11 And the “Average Claim Size” can be found by dividing the two tables: Avg Claim Size Development Year Year 0 1 2 3 4 5 2008 $1,454.19 $1,465.59 $1,461.26 $1,453.50 $1,450.88 $1,449.29 2009 $1,569.66 $1,578.80 $1,563.21 $1,553.76 $1,549.73 $1,547.13 2010 $1,599.77 $1,576.66 $1,576.51 $1,565.72 $1,560.71 $1,558.64 2011 $1,645.56 $1,605.20 $1,588.30 $1,574.14 $1,571.63 $1,570.63 2012 $1,516.08 $1,523.06 $1,519.17 $1,510.22 $1,508.55 $1,506.65 2013 $1,611.52 $1,589.54 $1,573.37 $1,568.94 $1,565.89 $1,563.98 2014 $1,452.75 $1,453.41 $1,447.14 $1,442.77 $1,438.58 2015 $1,540.22 $1,561.51 $1,538.12 $1,527.06 2016 $1,721.20 $1,688.96 $1,652.23 2017 $1,680.45 $1,642.62 2018 $1,631.54 0.9932835 0.9912125 0.9942143 0.997837 0.9987964 12 6 7 8 9 10 $1,448.61 $1,447.78 $1,447.47 $1,447.32 $1,447.32 $1,546.62 $1,546.21 $1,546.06 $1,545.86 $1,558.08 $1,557.64 $1,557.64 $1,570.05 $1,569.41 $1,505.93 0.9996022 0.9996207 0.9999004 0.9998811 1 The development factors are listed below the tables. They are less than one, implying that average claim size *decreases* with time. The average claim size triangle would be completed like this: Development Year Year 0 1 2 3 4 5 2008 1454.19 1465.5859 1461.2588 1453.4984 1450.8835 1449.286 2009 1569.66 1578.7966 1563.2128 1553.7588 1549.7334 1547.1294 2010 1599.7701 1576.663 1576.5127 1565.7235 1560.7112 1558.6352 2011 1645.56 1605.1964 1588.2957 1574.1403 1571.6283 1570.6291 2012 1516.08 1523.0567 1519.1702 1510.2229 1508.5485 1506.646 2013 1611.5199 1589.5385 1573.3742 1568.9377 1565.8862 1563.9832 2014 1452.7499 1453.4075 1447.1436 1442.7692 1438.5829 1436.85 2015 1540.2201 1561.5129 1538.1151 1527.0586 1523.76 1521.92 2016 1721.2001 1688.9599 1652.2339 1642.67 1639.12 1637.15 2017 1680.4501 1642.6202 1628.19 1618.77 1615.26 1613.32 2018 1631.5401 1620.58 1606.34 1597.05 1593.59 1591.67 13 6 7 8 9 10 1448.6097 1447.7781 1447.4709 1447.3185 1447.318477 1546.6238 1546.2099 1546.0637 1545.8603 1545.86 1558.0756 1557.6389 1557.6389 1557.45 1557.45 1570.0471 1569.4067 1569.25 1569.06 1569.06 1505.9338 1505.36 1505.21 1505.03 1505.03 1563.36 1562.77 1562.61 1562.43 1562.43 1436.28 1435.74 1435.59 1435.42 1435.42 1521.32 1520.74 1520.59 1520.41 1520.41 1636.50 1635.88 1635.71 1635.52 1635.52 1612.68 1612.07 1611.91 1611.71 1611.71 1591.04 1590.44 1590.28 1590.09 1590.09 And multiplied by the completed “number of claims” table on page 3 to get: Completed Triangle Development Year Year 0 1 2 3 4 5 2008 $4,407,650 $6,066,060 $6,645,805 $6,832,896 $6,888,795 $6,905,848 2009 $4,506,494 $6,296,241 $6,860,941 $7,066,495 $7,148,920 $7,170,945 2010 $4,738,519 $6,372,872 $7,013,905 $7,230,511 $7,296,325 $7,327,144 2011 $5,613,005 $7,501,083 $8,136,839 $8,316,183 $8,405,068 $8,418,572 2012 $4,910,583 $6,747,141 $7,416,589 $7,602,462 $7,692,089 $7,721,561 2013 $5,102,072 $6,916,082 $7,514,435 $7,728,587 $7,813,772 $7,829,300 2014 $4,786,811 $6,627,538 $7,254,531 $7,508,171 $7,587,086 $7,608,551 2015 $4,808,567 $6,622,376 $7,204,531 $7,397,072 $7,474,212 $7,495,358 2016 $5,418,338 $7,363,865 $7,869,590 $8,091,446 $8,175,827 $8,198,958 2017 $5,274,933 $6,997,562 $7,623,347 $7,838,261 $7,920,002 $7,942,409 2018 $5,385,714 $7,337,039 $7,993,184 $8,218,523 $8,304,230 $8,327,724 14 6 7 8 9 10 $6,911,317 $6,914,588 $6,916,016 $6,916,735 $6,916,735 $7,177,881 $7,180,599 $7,181,466 $7,182,067 $7,182,067 $7,333,862 $7,336,479 $7,336,479 $7,337,164 $7,337,164 $8,429,583 $8,432,422 $8,433,372 $8,434,159 $8,434,159 $7,731,464 $7,734,480 $7,735,352 $7,736,074 $7,736,074 $7,837,645 $7,840,703 $7,841,586 $7,842,318 $7,842,318 $7,616,661 $7,619,632 $7,620,491 $7,621,202 $7,621,202 $7,503,347 $7,506,274 $7,507,120 $7,507,821 $7,507,821 $8,207,697 $8,210,899 $8,211,824 $8,212,591 $8,212,591 $7,950,875 $7,953,976 $7,954,873 $7,955,615 $7,955,615 $8,336,601 $8,339,853 $8,340,792 $8,341,571 $8,341,571 The sum of the diagonal is $80,665,491 and the sum of the final column is $85,087,317 leading to a reserve of $4,421,826. If, instead, we had taken the overall average claim size of existing claims as a stable $1,548.58 and multiplied that by the 3031.64 expected ultimate claims, we would have a reserve of $4,694,731. Bornhuetter-Ferguson The “Bornhuetter-Ferguson” method combines two relatively independent measures of anticipated claims. The first is based the claim ladder result we’ve already discussed. So, as a first step, compute the claim ladder result including development factors. The second measure is based on the “loss ratio”. In general, claims should be a stable proportion of premiums. Let’s assume claims are expected to be 90% of premiums. The second step is then to find the “emerging liability” which is the expected total claims computed from the loss ratio minus the expected claims at the moment Let’s assume the claim table on Page 1. Assume premiums are $5,000 in 2008 and increase by $100 every year to reach $6,000 in 2018. Finally, assume a loss ratio of 90% expected in all years. As a reminder, the development factors on page 1 are: Development Year 0 1 2 3 4 5 6 7 8 9 10 1.371527 1.099087 1.034175 1.012619 1.004038 1.001464 1.00077 1.000212 1.000212 1 You can get the percentage completed at each duration by assuming 100% at time 10 and then dividing by the development factors at each time. For instance, at time 9: 15 9 1/1 1PctComplete At time 8: 8 1/1.000212 0.999788PctComplete At time 7: 7 0.999788 /1.000212 0.999576PctComplete And so on, giving: Development Year 0 1 2 3 4 5 Factor 1.371527 1.099087 1.034175 1.012619 1.004038 PctComp 0.629245 0.863026 0.948541 0.980957 0.993336 0.997346 PctIncomp 0.370755 0.136974 0.051459 0.019043 0.006664 0.002654 Development Year 6 7 8 9 10 Factor 1.001464 1.00077 1.000212 1.000212 1 PctComp 0.998807 0.999576 0.999788 1 1 PctIncomp 0.001193 0.000424 0.000212 0 0 The Percentage Incomplete is 1-PctComp. The reserves can be found by multiplying premium by loss ratio by PctIncomp and then adding up, like this: Times Emerging Premium Loss Ratio Liability 5000 4500 5100 4590 0.00 5200 4680 0.99 5300 4770 2.02 5400 4860 5.80 5500 4950 13.14 5600 5040 33.59 5700 5130 97.69 5800 5220 268.62 5900 5310 727.33 6000 5400 2002.08 3151.26 The reserve is $3,151.26 compared to an original reserve of $3,031.64. 16 For CT6 exam purposes, the loss ratio is often calculated from the most recent completed row in the claim lag table. In this case, the loss ratio would be 4779 0.9558 5000 . Using this instead of 0.90 would give: Times Emerging Premium Loss Ratio Liability 5000 4779 5100 4874.58 0.00 5200 4970.16 1.05 5300 5065.74 2.15 5400 5161.32 6.16 5500 5256.9 13.95 5600 5352.48 35.67 5700 5448.06 103.75 5800 5543.64 285.27 5900 5639.22 772.43 6000 5734.8 2126.21 3346.63 17 CHAPTER 2: CLASSICAL LOSS DISTRIBUTIONS The distributions we might use to fit claims are discussed below. We’ll give the CDF, PDF, mean, variance, standard deviation and (often) the moment generating function in terms of the parameters, as well as interesting features of the distributions. The Exponential Distribution ( ) 1 xF x e for 0x ( ) xf x e for 0x 1E X 2 1( )Var X 1( )x ( )XM t t for t The exponential distribution has a “memoryless” property, meaning Pr | PrX M x X M X x The Pareto Distribution ( ) 1F x x for 0x 1 ( )f x x for 0x 1 E X 2 2( ) 1 2 Var X ( ) 1 2 x The Pareto distribution remains Pareto when subject to an “excess” or “deductible”: Pr | MX M x X M M x 18 The Gamma Distribution , ( ) x F x for 0x 1( ) xf x x e for 0x E X 2( )Var X 1( )x ( )XM t t for t If events are occurring at a uniform (Poisson) rate per unit time, the time to the rth event follows a gamma distribution with parameters r and . The Weibull Distribution ( ) 1 cxF x e for 0x 1( ) cxf x c x e for 0x 11 11E X c 2 2 1 2 1( ) 1 1Var X c 2 1 1 2 1( ) 1 1x c It has heavier tails than the exponential distribution, but not so heavy as Pareto distribution 19 The Lognormal Distribution ln( ) xF x N for 0x 2 2 (ln ) 21( ) 2 x f x e x for 0x 2 2E X e 2 22( ) 1Var X e e 2 2 2( ) 1x e e This is used for stock values, for instance Mixture Distributions Discrete: 1 2( ) ( ) (1 ) ( )F x pF x p F x Continuous: 1 1 1( ) ( | ,... ) ( ,... ) , ...n n nf x f x g d d Example 1: x is Poisson, with parameter distributed as a gamma distribution… 1 0 ( ) ! ( ) xe ef x d x 11 0( ) ! x e d x 1 0 1 ( ) ! 1 x uu e du x 1 0( ) ! 1 x u x u e dux ( ) 1 ( ) ! 1 1 x x x which is a negative binomial distribution with parameters and 1 p . Example 2: x is exponential, with parameter distributed as a gamma distribution… 1 0 ( ) ( ) x ef x e d ( ) 0( ) xe d 0( ) uu due x x 1 0( ) uu e du x 1 ( 1) ( ) x 1x Which is a Pareto distribution with parameters and . Fitting distributions using Method of Moments… If there is a single parameter, set expected value equal. For instance, let M be the mean of the actual data. 20 For the exponential distribution, 1E X M so 1 M . If there are two parameters, set expected value and variance (or standard deviation or 2E X ) equal to each other. For instance, let M be the mean of the actual data and S be the standard deviation of the actual data. For the Pareto Distribution, 1 E X M and 2 2 2 2( ) 21 2 Var X S M . This implies 2 22 S M 2 2 2 S M 2 2 2 22 1 S S M M 2 2 2 2S S M . We can then find 1 M For the gamma distribution, E X M and 2 2( )Var X S . This implies 2 M S and 2 2 MM S For the Weibull Distribution the method is too difficult to apply by hand, although a computer could do it. For the Lognormal Distribution, 2 2E X e M and 2 2 22 2 2( ) 1 1Var X e e M e S This implies 2 2ln 1 M S and 2 ln 2 M The Maximum Likelihood Method involves maximizing 1 ( ) | n i i L f x or 1 ( ) ln | n i i l f x . Take the derivatives with respect to the parameters and set equal to “0”. For the exponential distribution, 1 ln i n x i l e 1 ln n i i x 1 ln n i i n x Now: 1 0 n i i l n x implies 1 1 n i i n Mx , the same as the method of moments. 21 For the Pareto Distribution, 11 , ln n i l x 1 ln ln 1 ln n i i x 1 ln ln 1 ln n i i n n x . Therefore 1 ln ln 0 n i i l n n x implying 1 ln 1 n i i n x Similarly, 1 11 0 n i i l n x implying 1 1 1n i i n i i i x x x . Setting them equal gives a non-linear equation for that can only be solved numerically. 1 1 1 0 ln 1 n i i n i i i i x n x x x . The value of and then be used to find . For the gamma distribution, numerical methods are again necessary. For the Weibull distribution, numerical methods are again necessary. For the Lognormal distribution, you would find the MLE parameters to be: 1 ln n i i x n and 2 1 ln n i i x n The book discusses various test, such as Kolmogorov-Smirnov, Anderson-Darling, Chi-Square and AIC. These are not part of the CT6 syllabus and are beyond the scope of ACTS 336. Types of reinsurance 1. Excess of Loss Insurer pays the first M and the reinsurer pays 0 if X M and X M otherwise. The mean amount paid by the insurer is 0 ( ) ( ) M M xf x dx M f x dx The mean amount paid by the reinsurer is ( ) M x M f x dx 22 The pdf of the reinsurers payout *conditional* on payout occurring is ( )( ) 1 ( ) f w Mg w F M MLE can be done by either the insurer or the reinsurer. For the reinsurer, just use ( | )g w for the likelihood of a payout w and for the insurer use 1 ( )F M for the likelihood of each claim that exceeds the deductible (assuming you don’t know the actual amount). For example: Exponential Distribution Assume there are n values of ix as well as m values of M . In this case 1 1 ln lni n m x M i i l e e 1 ln n i i n x mM . Now 1 0 n i i l n mM x implies 1 n i i n mM x 2. Proportional In this case, the company pays X and the reinsurer pays 1 X . The means, variances, and maximum likelihood estimators are straightforward to determine. While they are not discussed specifically in this chapter, we will use the following discrete distributions later: Binomial: Pr 1 n iiN i p p for 0,1,..,i n ; E X np ; ( ) (1 )Var X np p ; ( ) 1 ntSM t pe p Negative Binomial: 1 !Pr (1 ) !( 1)! k nk nN n p p n k for 0,1,2...n ; 1E X pk p ; 2( ) 1r X p V ka p ; ( ) 1 (1 ) kk tSM t p p e . The Geometric Distribution is the special case 1k . And the following continuous distribution will be used later: Beta: 11( ) 1f x x x for 0 1x ; E X ; 2 ( ) 1 Var X ; the moment generating function is too complicated to include. 23 Chapter 3: RISK THEORY Let S be a random loss from a business line, reflected in two random variables: the number of claims (or “Frequency”) N , and N values of the loss per claim (or “Severity”) iX so that 1 N i i S X . In general: E S E N E X 2( ) ( ) ( )Var S E N Var X E X Var N And ( ) ln ( )S N XM t M M t Some Specific Cases: Compound Poisson Distribution: Pr ! neN n n for 0,1,2...n E S E X 2( )Var S E X ln ( ) 1 ( ) 1( ) M tX X e M t SM t e e A sum of independent compound poisson random variables is also compound poisson. The new parameters 1 n i i and 1 1( ) ( ) i n X i X i F x F x . For example: Let 1S be compound poisson with 1 and a severity distribution of 100% chance of $20,000. Let 2S be compound poisson with 2 and a severity distribution of 100% chance of $10,000. 1 2S S S is also compound poisson. 1 2 3 and 1 2 1 2( ) ( ) ( ) 3 3 F x F x F x . In this case: 1( ) 0F x if 20,000x and 1( ) 1F x if 20,000x 2 ( ) 0F x if 10,000x and 2 ( ) 1F x if 10,000x Leading to: ( ) 0F x if 10,000x 2( ) 3 F x if 10,000 20,000x ( ) 1F x if 20,000 x . In order to check this, we can add 1S and 2S directly via convolution: 24 1S 2S 1 2S S S Value Prob Prob Prob $0 1e 2e 1 2 0.049787068e e $10,000 0 22e 1 22 0.099574137e e $20,000 1e 22e 1 2 1 22 0.149361205e e e e $30,000 0 2 4 3 e 1 2 1 24 2 0.1659568953e e e e $40,000 1 2 e 22 3 e 1 1 2 1 2 22 2 0.15765905 3 2 ee e e e e $50,000 0 2 4 15 e 1 1 2 1 2 24 4 2 0.129446378 15 3 2 ee e e e e $60,000 1 6 e 24 45 e 1 1 1 2 1 2 2 24 2 2 45 3 2 6 e ee e e e e e 0.095701809 Or, we can determine the probabilities from the compound distribution: 0N 1N 2N 3N 4N 5N 6N Prob 3e 33e 3 9 2 e 39 2 e 327 8 e 381 40 e 381 80 e Value ( | )f s N ( | )f s N ( | )f s N ( | )f s N ( | )f s N ( | )f s N ( | )f s N $0 1 0 0 0 0 0 0 $10,000 0 2/3 0 0 0 0 0 $20,000 0 1/3 4/9 0 0 0 0 $30,000 0 0 4/9 8/27 0 0 0 $40,000 0 0 1/9 12/27 16/81 0 0 $50,000 0 0 0 6/27 32/81 32/243 0 $60,000 0 0 0 1/27 24/81 80/243 64/729 25 Value Total $0 3 0.049787068e ✓ $10,000 32 3 0.0995741373 e ✓ $20,000 3 31 4 93 0.1493612053 9 2e e ✓ $30,000 3 3 4 9 8 9 0.165956895 9 2 27 2 e e ✓ $40,000 3 3 3 1 9 12 9 16 27 0.15765905 9 2 27 2 81 8 e e e ✓ $50,000 3 3 3 6 9 32 27 32 81 0.129446378 27 2 81 8 243 40 e e e ✓ $60,000 3 3 3 3 1 9 24 27 80 81 64 81 0.095701809 27 2 81 8 243 40 729 80 e e e e ✓ Compound Binomial Distribution: !Pr (1 ) !( )! i n inN i p p i n i for 0,1, 2...,i n E S npE X 22 2( )Var S npE X np E X ln ( )( ) 1 ( ) 1X n nM tS XM t pe p pM t p Compound Negative Binomial Distribution: 1 !Pr (1 ) !( 1)! k nk nN n p p n k for 0,1,2...n 1 pE S k E X p 2 221 1( ) p pVar S k E X k E X p p ln ( )( ) 1 (1 ) 1 (1 ) ( )X k kM tk S X pM t p p e p M t 26 Interestingly, one can get a distribution recursively if two conditions hold: 1. Pr( ) Pr( 1) N i ba N i i 2. The severity distribution is restricted to positive integers. Condition one holds for the Poisson distribution, the Binomial Distribution and the Negative Binomial distribution and these are the only non-negative random variables that do. For the Poisson Distribution, 0a and b For the binomial distribution, 1 pa p and ( 1) 1 pb n p For the negative binomial distribution, 1a p and ( 1)(1 )b k p If these conditions hold, 1 ( ) ( ) ( ) x s s i bif x a p i f x i x Let’s test this out on our compound poisson distribution above, where 2( ) 3 p i if $10,000i , 1( ) 3 p i if $20,000i and ( ) 0p i otherwise. 1 ( ) ( ) ( ) x s s i if x p i f x i x Now, start off with: 3(0) 0.049787068sf e ✓ 3(10,000) 2(10,000) (0) 0.099574137 10,000 3s s f f ✓ If x is divisible by 10,000 and 20,000 we have: 3(10,000) 2 3(20,000) 1( ) ( 10,000) ( 20,000) 3 3s s s f x f x f x x x 20,000 20,000( 10,000) ( 20,000)s sf x f xx x 20,000 ( 10,000) ( 20,000)s sf x f xx So 2(20,000) (10,000) (0) 0.149361205 2s s s f f f ✓ 2(30,000) (20,000) (10,000) 0.165956895 3s s s f f f ✓ 2(40,000) (30,000) (20,000) 0.15765905 4s s s f f f ✓ 27 2(50,000) (40,000) (30,000) 0.129446378 5s s s f f f ✓ 2(60,000) (50,000) (40,000) 0.095701809 6s s s f f f ✓ And so on… In fact, we can get 2(70,000) (60,000) (50,000) 0.064328053 7s s s f f f without any convolutions at all! Proportional and Excess of Loss Reinsurance works just as before, with the caveat that the Excess of Loss could be on the total S or on each iX individually. The “Individual Risk” model We now assume there are n individual policies, each one producing either zero claims or one claim (with probability p ). As before, the severity distribution is ( ) iX F x which is the CDF conditional on a claim arising. The loss is 1 n i i S X where the upper limit is no longer random. In a sense, this is just a compound binomial model with: E S npE X 22 2( )Var S npE X np E X Compound Poisson approximations to the Individual Risk Model. Set the means equal, that is: E S npE X n E X . If p means are the same. 22 2 2( )Var S n E X npE X np E X Difference should be small if p is small. Variability in a heterogenous portfolio: Example: Assume Compound Poisson where the Poisson parameter is 0.02 or 0.04 with equal probability. There is a new draw of for each policy. Also assume claim severity is an exponential distribution with mean $1000. 1. Find the mean and variance of the claim number on a single policy. 0.5(0.02) 0.5(0.04) 0.03E N 2 2 20.5(0.02 0.02 ) 0.5(0.04 0.04 ) 0.031E N 28 2( ) 0.031 0.03 0.0301Var N The variance is slightly larger than the variance for the average 0.03 2. Find the mean and variance of the loss amount on a randomly selected policy: 0.03(1,000) $30E S E N E X 2 2 2( ) ( ) ( ) 0.03(1,000) (1,000) (0.0301) 60,100Var S E N Var X E X Var N The standard deviation for one policy is $245.15 3. Find the mean and variance of the total loss on 200 policies: The 200 policies are independent, since the draws are independent. The mean is 200(30) $6,000 . The variance is 200(60,100) 12,020,000 The standard deviation on the portfolio is $3,466.99 or $17.33 per policy. Now assume a compound binomial where the probability is 0.02p or 0.04p with equal probability. There is a new draw of p for each policy. Again assume claim severity is an exponential distribution with mean $1000. 4. Find the mean and variance of the claim number on a single policy. 0.5(0.02) 0.5(0.04) 0.03E N 2 2 20.5 0.02(0.98) 0.02 0.5 0.04(0.96) 0.04 0.03E N 2( ) 0.03 0.03 0.0291Var N The variance is *the same* as you would get for the average 0.03p 5. Find the mean and variance of the loss amount on a randomly selected policy: 0.03(1,000) $30E S E N E X 2 2 2( ) ( ) ( ) 0.03(1,000) (1,000) (0.0291) 59,100Var S E N Var X E X Var N The standard deviation for one policy is $243.10 6. Find the mean and variance of the total loss on 200 policies: The 200 policies are independent, since the p draws are independent. The mean is 200(30) $6,000 . The variance is 200(59,100) 11,820,000 The standard deviation on the portfolio is $3,438.02 or $17.19 per policy. We’ve already seen on page 17 some interesting features of the continuous version of this problem… 29 Variability in a homogenous portfolio. Example: Assume Compound Poisson where the Poisson parameter is 0.02 or 0.04 with equal probability. The value of drawn applies to all policies. Also assume claim severity is an exponential distribution with mean $1000. 7. Find the mean and variance of the claim number on a single policy. 0.5(0.02) 0.5(0.04) 0.03E N 2 2 20.5(0.02 0.02 ) 0.5(0.04 0.04 ) 0.031E N 2( ) 0.031 0.03 0.0301Var N This is the same as before 8. Find the mean and variance of the loss amount on a randomly selected policy: 0.03(1,000) $30E S E N E X 2 2 2( ) ( ) ( ) 0.03(1,000) (1,000) (0.0301) 60,100Var S E N Var X E X Var N The standard deviation for one policy is $245.15 This is the same as before. 9. Find the mean and variance of the total loss on 200 policies: The 200 policies are no longer independent, since they all depend on the same draw. The mean is 0.5(200)(20) 50(200)(40) $6,000 . The 2 2 20.5 | 0.02 0.5 | 0.04E S E S E S 22 | 0.02 | 0.02 | 0.02E S E S Var S 2| 0.02 ( ) ( )Var S E N Var X E X Var N 2 24(1,000) 4(1,000) 8,000,000 So 22 | 0.02 4,000 8,000,000 24,000,000E S And 2| 0.04 ( ) ( )Var S E N Var X E X Var N 2 28(1,000) 8(1,000) 16,000,000 So 22 | 0.04 8,000 16,000,000 80,000,000E S So 2 2 20.5 | 0.02 0.5 | 0.04E S E S E S 52,000,000 The variance is 22 16,000,000E S E S The standard deviation on the portfolio is $4,000 or $20 per policy. 30 This is noticeably larger than the standard deviation for the heterogeneous case. For a generic number of policies n . You would find the standard deviation per policy is 60010 1 n where as in the heterogeneous case you would find 60110 n Now assume a compound binomial where the probability is 0.02p or 0.04p with equal probability. The value of p drawn applies to all policies. Again assume claim severity is an exponential distribution with mean $1000. 10. Find the mean and variance of the claim number on a single policy. 0.5(0.02) 0.5(0.04) 0.03E N 2 2 20.5 0.02(0.98) 0.02 0.5 0.04(0.96) 0.04 0.03E N 2( ) 0.03 0.03 0.0291Var N The variance is *the same* as you would get for the average 0.03p and is the same as before. 11. Find the mean and variance of the loss amount on a randomly selected policy: 0.03(1,000) $30E S E N E X 2 2 2( ) ( ) ( ) 0.03(1,000) (1,000) (0.0291) 59,100Var S E N Var X E X Var N The standard deviation for one policy is $243.10 This is the same as before. 12. Find the mean and variance of the total loss on 200 policies: The 200 policies are no longer independent, since they all depend on the same draw. The mean is 0.5(200)(20) 50(200)(40) $6,000 . The 2 2 20.5 | 0.02 0.5 | 0.04E S E S p E S p 22 | 0.02 | 0.02 | 0.02E S p E S p Var S p 2| 0.02 ( ) ( )Var S p E N Var X E X Var N 2 24(1,000) 3.92(1,000) 7,920,000 So 22 | 0.02 4,000 7,920,000 23,920,000E S p And 2| 0.04 ( ) ( )Var S p E N Var X E X Var N 2 28(1,000) 7.68(1,000) 15,680,000 So 22 | 0.04 8,000 15,680,000 79,680,000E S p 31 So 2 2 20.5 | 0.02 0.5 | 0.04E S E S p E S p 51,800,000 The variance is 22 15,800,000E S E S The standard deviation on the portfolio is $3,974.92 or $19.87 per policy. Again, this is noticeably larger than the standard deviation for the heterogeneous case. For a generic number of policies n . You would find the standard deviation per policy is 59010 1 n where as in the heterogeneous case you would find 59110 n . Premiums and Reserves based on mean/variance analysis and a survival probability… Let’s use the original compound poisson heterogeneous policyholder model above. 1. How much premium should be charged to be 99% sure of survival on if you issue 20,000 policies? If 20,000n then the mean of the total loss is 20,000(30) $600,000 . The standard deviation is 60110 $34,699.87n n . The 99th percentile of a normal distribution is 2.236 so you should charge 600,000 34,669.87(2.236) $677,521.83 in total or $33.88 per policy. 2. If you only issue 15,000 policies with the premium in part 1, what reserve would you need to hold to be 99% sure of survival? You would receive 15,000(33.87609165) $508,141.37 in premiums. The losses would be mean $450,000 and standard deviation 60110 $30,024.99n n . Assuming you hold a reserve of V , the total loss is mean 58,141.37 V and standard deviation $30,024.99 . We want the 99th percentile to be greater than 0, so 58,141.37 2.236(30,024.99) 0V which solves for $8,994.51V One could also work out cases where the parameters of the severity distribution are uncertain (or all parameters are uncertain). The math will be much more complicated in these cases. However, the general form of the standard deviation, 1 ba n and 1 ba n would remain the same. How would you 32 work out a and b ? I’m glad you asked. a is the standard deviation of the policy means conditional on the parameters and b is what’s necessary to set 1a b equal to the standard deviation of losses on one randomly selected policy. For example… Assume Compound Poisson where the Poisson parameter is 0.02 or 0.04 with equal probability. There is a new draw of for each policy as before. Also assume claim severity is an exponential distribution, but now if 0.02 the mean of the severity distribution has a 70% chance of being $1,000 and a 30% chance of being $3,000. If 0.02 the mean of the severity distribution has a 20% chance of being $1,000 and a 80% chance of being $3,000. This means there are four types of policies: 35% chance of a mean $20, variance 2 2 2( ) ( ) ( ) 0.02(1,000) (1,000) (0.02) 40,000Var S E N Var X E X Var N 15% chance of a mean $60, variance 2 2 2( ) ( ) ( ) 0.02(3,000) (3,000) (0.02) 360,000Var S E N Var X E X Var N 10% chance of a mean $40, variance 2 2 2( ) ( ) ( ) 0.04(1,000) (1,000) (0.04) 80,000Var S E N Var X E X Var N 40% chance of a mean $120, variance 2 2 2( ) ( ) ( ) 0.04(3,000) (3,000) (0.04) 720,000Var S E N Var X E X Var N The mean is 0.35(20) 0.15(60) 0.10(40) 0.40(120) $68 . a is the standard deviation of the policy means conditional on the parameters, that is 2 2 2 20.35(20 68) 0.15(60 68) 0.10(40 68) 0.40(120 68)a 44.45222154 The standard deviation of the loss for one policy is 221a b E S E S . 2 2 2 2 20.35 20 40,000 0.15 60 360,000 0.10 40 80,000 0.40 120 720,000E S 2 0.35 40, 400 0.15 363,600 0.10 81,600 0.40 734, 400 370,600E S So 21 370,60044.45222 68 604.9595028154 b Implying 2 1 44.4522215 604.9595028 184.2105775 4 b With 200 heterogeneous policies, the standard deviation per policy would be 184.2105775 1 $42.78 200 44.45222154 . With 200 homogeneous policies, the standard deviation per policy would be 184.2105744.452221 751 $61.61 200 54 . 33 Chapter 4: Ruin Theory. First, we’ll consider the “Compound Poisson Process”. Suppose claims arrive via a memoryless process and a new claim is equally likely regardless of how recently the last claim occurred. If the claims arrive at a rate per unit time, it can be shown that the time between claims is exponential with mean and parameter 1 . In any given time interval ,t t T the number of claims is Compound Poisson with mean T . The process ( )S t for the losses is a “Compound Poisson” process. We then define the “Surplus Process” ( ) ( )U t u ct S t , premiums being level as a function of time. A potential path would look something like this… Ruin occurs at time 1.358218462 . We’re interested in the distribution of things like the time-to-ruin, probability of ruin, negative surplus at time of ruin and so on. Many of the equations are not easily solveable and we have only approximations. 34 Let’s see how far we get… Define the time-to-ruin min( : 0 & ( ) 0)T t t U t . T If ( ) 0U t for all 0t ( )u is the probability of ruin in an infinite time given an initial surplus u ( , )u t is the probability of ruin sometime in the interval (0, ]t given an initial surplus u We won’t prove this, but it turns out: ( ) ( ) | Ru RU T eu E e T where R is the unique positive solution to ( ) 0XM r cr . If (1 )c E X then ( ) 1 (1 )XM r E X r has a solution independent of . In practice, one usually needs to find the “adjustment coefficient” R numerically and approximate ( ) |RU TE e T in some way. The denominator is always greater than 1 so ( ) Ruu e called the “Lundberg Upper Bound” There are some special cases that can be solved exactly. For instance… Exponential claim distribution: Let’s say ( ) xf x e reparameterizing to avoid confusion. ( )XM r r So solve 1 (1 ) r r 2 (1 )r r 2 2 2(1 ) (1 )r r r r So 2(1 ) 0r r 1 r Now we need to find ( ) |RU TE e T which requires the distribution function for ( ) |U T T . Well, for a given ( )U T just before ruin, ( )U T is distributed exponentially with parameter due to the memoryless property of the exponential distribution. Therefore, the distribution of ( )U T is independent of ( )U T and distributed exponentially with parameter . I might put in a more formal proof later. Therefore ( ) |RU T RzE e T E e where z is exponentially distributed. 35 0 1 1 1 1 Rz Rz xE e e e dx R So 11( ) 1 u u e Numerical Example: If 3,000 and 1 $10,000 . That is, our portfolio produces 3,000 claims with mean claim amount $10,000. Also assume we have 30,000 policies and charge $1,200 per policy. How much capital do we need to have a 95% chance of solvency over the long haul? 30,000($1, 200) $36,000,000C 3,000($10,000) $30,000,000E X So 36,000,000 1 0.2 30,000,000 0.2 1 1.2 10,000 0.0000166661( ) 0.83333 1.2 u uu e e We want 0.000016666( ) 0.83333 0.05uu e So 0.000016666 0.06ue 0.000016666 ln 0.06 2.813410717u $168,804.64u This next stuff is not in the book, but is valuable… First Surplus Below the Initial Level. I may eventually put a proof of this in an appendix: Theorem: The probability that surplus u will ever fall below its initial level and will be between u y and u y dy when it does is: 1 ( )1 ( ) (1 ) X X F yF y dy c E X for 0y . Given this theorem, what is the probability that the surplus will ever fall below the initial level. That is, what is (0) ? Well… 36 0 1(0) 1 ( ) (1 ) X F x dx E X Integrate by parts with 1 ( )Xu F x v x ( )Xdu f x dv dx 0 1(0) 1 ( ) (1 ) X x F x E X 0 1( ) 1Xxf x dx This is independent of and the distribution of claims! Let 1L be the amount by which surplus falls below its initial level given that is does… 1 1 ( ) (1 ) 1 ( )( ) 1 1 X X L F y E X F yf y E X for 0y So we now have a pdf for the for the ( ) |U T T when 0u . Example: If X is distributed uniformly on [0,1], what is the distribution of surplus level the first time surplus drops below its initial level? ( ) 0XF x for 0x ( )XF x x for 0 1x ( )XF x x for 1 x And 0.5E X So we have 1 ( ) 0Lf y for 0y 1 ( ) 2(1 )Lf y y for 0 1y 1 ( ) 0Lf y for 1 y Interestingly… 1 1 (0) ( ) 1 1(0) ( )| R RU T RL L e M RE e T E e Also, we can get the moment generating function for 1L 1 0 1( ) 1 ( )rx rxL XM r E e e F x dxE X Integrate by parts with 1 ( )Xu F x /rxv e r ( )Xdu f x rxdv e dx 37 1 00 1 1( ) 1 ( ) ( ) rx rx L X X eM r F x e f x dx E X r r 1 1 10 ( )XM rE X r r 1 ( ) 1XM rrE X Substituting this into 1 1(0) ( )LM R we find: (0) ( ) 1X RE X M R And from the definition ( ) 1 (1 )XM R E X R we again find: 1(0) 1 Now consider the “Maximal Aggregate Loss” 0 max ( ) t L S t ct . This is also the maximum of ( )u U t This implies ( ) Pru L u Looking at the process ( )S t ct , after each “record high”, the probability that surplus will drop below this amount is (0) . Therefore, the probability that this is the overall record high is 1 (0) . So 1 2 ... NL L L L where N is the total number of new record highs. Now Pr( 0) 1 (0)N Pr( 1) (0) 1 (0)N 2Pr( 2) (0) 1 (0)N And so on. So N is a geometric distribution and, knowing what we know about moment generating functions, ( ) 1N r M r e That makes the distribution of L a compound geometric distribution, so: 1 1 ( ) ln ( ) 1 ( )L N L L M r M M r M r 11 ( ) 1XM rrE X 1 1 ( )X E X r E X r M r Or, equivalently… ( ) 11( ) 1 1 1 1 ( ) X L X M r M r E X r M r 38 This can be decomposed into a point mass at “0” (i.e. a probability of 1 that the company survives) plus the moment generating function for * | 0L L L , * ( ) 1 ( ) 1 1 ( ) X L X M r M r E X r M r This gives us a procedure for finding ( )u … 1) Find the moment generating function * ( ) 1 ( ) 1 1 ( ) X L X M r M r E X r M r 2) Invert it to get * ( )Lf t . This is beyond the scope of this course except for a few interesting cases. 3) Set *( ) (0) ( )Lu f u 4) Integrate to get * 0 0 1( ) ( ) (0) 1 ( ) 1 u u L u t dt f t dt Let’s try the exponential distribution this way, which we already know from other methods… ( )XM r r ; 1E X So * * * 1 1( ) 11 1 1 L r M r rr r r defining * 1 . This means the maximal aggregrate loss is exponential and * * *( ) t L f t e so ** 0 1( ) 1 1 u tu e dt *1 1 1 1 ue 11 1 u e as before!!! This process allows us to solve some other examples. For instance, here is a mixture of exponentials. 2 101 2( ) 2 103 3 x xf x e e We’ll assume a loading 0.2 . 1 2 2 10 60 22( ) 3 2 3 10 3(2 )(10 )X rM r r r r r 1 1 2 1 0.233333333 3 2 3 10 E X So 39 * 60 220.2 1 3(2 )(10 ) ( ) 60 221 1.2 0.233333 3(2 )(10 ) L r r r M r rr r r 2 3.33333333 0.714285714 3.33333333 8.42857142 r r r Now do a “partial fractions decomposition”. The denominator has roots: 28.42857142 8.42857142 4 1 3.33333333 2 1 r That is, 0.41601364r or 8.012557788 so * 3.33333333 0.714285714( ) (0.41601364 )(8.012557788 )L rM r r r 0.41601364 8.012557788 0.41601364 8.012557788 A B r r Multiplying both sides by the denominator and grouping terms gives: 3.33333333 3.333333333 0.41601364 8.012557788 3.33333333 0.714285714A B A B r r Giving two equations in two unknowns… 1A B and 0.41601364 8.012557788 0.714285714A B These solve for: 0.96073582A And 0.03926418B So * * 1 2 * * * 1 2( ) 0.96073582 0.03926418 t t L f t e e with *1 0.41601364 and *2 8.012557788 Now * *1 2* *1 201( ) 1 0.96073582 0.039264181 u t tu e e dt * *1 21( ) 1 0.96073582 0.96073582 0.03926418 0.039264181 u uu e e 0.41601364 8.0125577885 0.96073582 0.03926418 6 u ue e Or, a simple Gamma distribution: 31( ) 9 xf x xe Again assume a loading 0.2 . 40 23( ) 3X M r r 2 3 E X So * 2 2 30.2 1 3 ( ) 31 1.2 0.66666667 3 L r M r r r 2 1.5 2.5 1.5 4.75 r r r Again do a “partial fractions decomposition”. The denominator has roots: 24.75 4.75 4 1 1.5 2 1 r That is, 0.340147425r or 4.409852575 so * 1.5 2.5( ) (0.340147425 )(4.409852575 )L rM r r r 0.340147425 4.409852575 0.340147425 4.409852575 A B r r Multiplying both sides by the denominator and grouping terms gives: 1.5 1.5 0.340147425 4.409852575 1.5 2.5A B A B r r Giving two equations in two unknowns… 1A B and 0.340147425 4.409852575 2.5A B These solve for: 1.022150849A And 0.022150849B So * * 1 2 * * * 1 2( ) 1.022150849 0.022150849 t t L f t e e with *1 0.340147425 and * 2 4.409852575 Now * *1 2* *1 201( ) 1 1.022150849 0.0221508491 u t tu e e dt * *1 21( ) 1 1.022150849 1.022150849 0.022150849 0.0221508491 u uu e e 41 0.3401474254 4.4098525755 1.022150849 0.022150849 6 u ue e Interestingly, it turns out in both of these cases that the smaller exponent is the adjustment coefficient. While I have no proof of this, I strongly suspect it is true. By the way, here’s a decent approximation to ( )u : *[ ]E L is the derivative of the MGF at 0r , so let’s find it. Remember, * ( ) 1 ( ) 1 1 ( ) X L X M r M r E X r M r And by definition 2 2( ) 1 ... 2X E X M r E X r r so * 2 2 2 2 ... 2( ) 1 ... 2 L E X E X r r M r E X E X r E X r r 2 2 2 2 ... 2 ... 2 E X E X r r E X E X r r 2 2 1 ... 2 1 ... 2 E X r E X E X r E X 2 2 1 ... 1 ... 2 2 E X E X r r E X E X 2 1 11 ... 2 2 E X r E X 2 11 ... 2 E X r E X 42 Therefore, 2 * 1 2 E X E L E X If you assume *L is exponential with this mean, you’ll find: 2 2 11( ) 1 E X u E X u e This is exact in the case of the exponential distribution. How good is it generally? For our mixture distribution, we would find: 1 1 2 1 0.233333333 3 2 3 10 E X And 2 2 2 1 2 2 2 0.1733333 3 2 3 10 E X The approximation is therefore: 0.4487179495( ) 6 uu e Recall the exact answer: 0.41601364 8.1025577885 0.96073582 0.03926418 6 u ue e Here’s a comparison: u ( )u Approx 0.00 0.833333 0.833333 0.01 0.827463 0.829602 0.10 0.782542 0.796767 0.25 0.725846 0.744903 0.50 0.650829 0.665857 1 0.528151 0.532038 2 0.348400 0.339678 4 0.151612 0.138457 10 0.012494 0.009377 100 6.85772E-19 2.71173E-20 Here’s another nice approximation when things are discrete, using the Panjer Recursion derived earlier. This is hard to implement by hand, but can be done well in a spreadsheet. Suppose, for example, that you have a loading 0.2 and a severity distribution where which is 70% chance of a $1 loss, 20% chance of a $2 loss and 10% chance of a $3 loss. 43 0.7(1) 0.2(2) 0.1(3) 1.4E X and 1 ( )Lf y can be reasonably approximated by a discrete pdf: 1 1 0(0.5) 0.714285714 1.4L f 1 1 0.7(1.5) 0.214285714 1.4L f 1 1 0.9(2.5) 0.071428571 1.4L f Remember L , the maximum aggregate loss, follows a compound geometric distribution with 1 1(0) 1 (0) 1 1 6L f . For the Panjer Recursion, 51 (0) 6 a p and ( 1)(1 ) 0b k p . The Recursion says: 1 1 5( ) ( ) ( ) 6 x L L L i f x f i f x i . We let i move in half-integer steps: 1 5(0.5) (0.5) ( 0.0992063490) 6L L L f f f 1 5(1) (0.5) (0.5 0.059051398) 6L L L f f f 1 1 5(1.5) (0.5) (1) (1.5) (0 0.064911 47 6 5)L L L L Lf f f f f 1 1 5(2) (0.5) (1.5) (1.5) (0. 0.0563532) 6 455L L L L Lf f f f f And, if 2.5x , 1 1 1 5( ) (0.5) ( 0.5) (1.5) ( 1.5) (2.5) ( 2.5) 6L L L L L L L f x f f x f f x f f x The c.d.f. can be found by adding up the pdf, and 1 ( )Lu F u . A relevant table is included on the next page: 44 u ( )Lf u ( )LF u u 0 0.166666667 0.166667 0.833333 0.5 0.099206349 0.265873 0.734127 15.5 0.003802 0.964443 0.035557 1 0.059051398 0.324924 0.675076 16 0.003435 0.967878 0.032122 1.5 0.064911547 0.389836 0.610164 16.5 0.003103 0.970981 0.029019 2 0.056353245 0.446189 0.553811 17 0.002803 0.973784 0.026216 2.5 0.054009126 0.500198 0.499802 17.5 0.002532 0.976316 0.023684 3 0.049644777 0.549843 0.450157 18 0.002288 0.978604 0.021396 3.5 0.043128506 0.592972 0.407028 18.5 0.002067 0.980671 0.019329 4 0.039179999 0.632152 0.367848 19 0.001867 0.982538 0.017462 4.5 0.035540926 0.667693 0.332307 19.5 0.001687 0.984225 0.015775 5 0.032071661 0.699764 0.300236 20 0.001524 0.985749 0.014251 5.5 0.029041749 0.728806 0.271194 20.5 0.001377 0.987125 0.012875 6 0.026200522 0.755006 0.244994 21 0.001244 0.988369 0.011631 6.5 0.023654774 0.778661 0.221339 21.5 0.001124 0.989492 0.010508 7 0.021381781 0.800043 0.199957 22 0.001015 0.990507 0.009493 7.5 0.019314943 0.819358 0.180642 22.5 0.000917 0.991424 0.008576 8 0.017449732 0.836808 0.163192 23 0.000828 0.992253 0.007747 8.5 0.015764475 0.852572 0.147428 23.5 0.000748 0.993001 0.006999 9 0.014240735 0.866813 0.133187 24 0.000676 0.993677 0.006323 9.5 0.012865377 0.879678 0.120322 24.5 0.000611 0.994288 0.005712 10 0.011622746 0.891301 0.108699 25 0.000552 0.99484 0.00516 10.5 0.010499964 0.901801 0.098199 25.5 0.000498 0.995338 0.004662 11 0.009485729 0.911287 0.088713 26 0.00045 0.995788 0.004212 11.5 0.008569421 0.919856 0.080144 26.5 0.000407 0.996195 0.003805 12 0.007741635 0.927598 0.072402 27 0.000368 0.996563 0.003437 12.5 0.006993827 0.934592 0.065408 27.5 0.000332 0.996895 0.003105 13 0.006318244 0.94091 0.05909 28 0.0003 0.997195 0.002805 13.5 0.005707921 0.946618 0.053382 28.5 0.000271 0.997466 0.002534 14 0.005156554 0.951774 0.048226 29 0.000245 0.997711 0.002289 14.5 0.004658447 0.956433 0.043567 29.5 0.000221 0.997932 0.002068 15 0.004208456 0.960641 0.039359 30 0.0002 0.998132 0.001868 The implication is that to be 95% certain of survival you would need $14 of capital. The ratio between consecutive integer values rapidly approaches 0.816137281 this implies an adjustment coefficient of approximately ln 0.816137281 0.203172701R . It turns out the true value of the adjustment coefficient is 0.201374171 which can be found from, say, EXCEL solver. The approximation 45 2 2 1 1.4 1 0.194444443 2.41 5 5( ) 1 6 6 E X u uE X uu e e e is worse. If we use this approximation to solve for the 95% safety level, we find 0.19444444 5 0.05 6 ue 14.4689694u which is still pretty close. Now we turn to an analysis of Reinsurance and the probability of ruin. We will use the adjustment coefficient as a proxy for safety. We’d like to enter into reinsurance transactions in order to maximize the adjustment coefficient. We’ll start with proportional reinsurance and move on to excess-of-loss reinsurance… Proportional Reinsurance: Assume the insurer retains a proportion and the reinsurer has a loading . The equation for the adjustment coefficient is now: ( ) 1 (1 ) (1 )(1 )XM r E X E X r And ( ) ( )X XM r M r so ( ) 1 ( ) (1 )XM r E X r We could try implicit differentiation, but we can hopefully get an answer for an exponential distribution with mean 1 1 ( ) (1 ) r r 2 1 r r 2 2 21 1r r r r So 21 0r r 21r We would then take the derivative w.r.t. and set it equal to zero. Let’s do a numerical example: Use our exponential from page 32… 46 1 $10,000 and 0.2 . Let’s assume we can get reinsurance at 0.3 . It will turn out that is irrelevant, only and matter. 2 0.1 0.3 0.1 1.3 r 2 22 0.1 1.3 0.3 0.1 0.3 0.1 2.6 0 0.1 1.3 r 20.1 1.3 0.3 0.1 0.3 0.1 2.6 0 2 20.03 0.39 0.01 0.29 0.78 0 20.39 0.26 0.01 0 20.26 0.26 4(0.39)(0.01) 2(0.39) 0.625686006 or 0.04098066 There is an important constraint that the insurer’s premium net of reinsurance must exceed the insurer’s claims net of reinsurance. That is… (1 ) (1 )(1 ) Or 1 In this case, must be greater than 1/3 so that 0.625686006 is the safest retention level. At this value of , what would be the amount of capital needed to be 95% sure of survival? Claims are still exponential so we can use 1( ) 1 Ruu e . The effective value of the loading is ( ) (1 ) 0.1 1.3(0.625686006)1 1 0.140175425 0.625686006 5 2 0.1 0.3 1.964915343 10 0.1 1.3 R We solve: 51.964915343 101( ) 0.05 1.140175425 uu e 51.964915343 10 0.057008771ue 51.964915343 10 ln(0.057008771) 2.864550141u $145,784.91u . For comparison, this is less than the answer for no reinsurance found earlier $168,804.64u Maximization with Excess-of-Loss Reinsurance… Here, the relevant parameter is M , the retention limit. 47 The insurer’s premium net of reinsurance is * (1 ) (1 )c E X E Z where 0,Z Max X M . The equation defining the adjustment coefficient R is therefore: * 0 ( ) 1 ( )M Rx RMc R e f x dx e F M Again, the values cancel out and we get: 0 1 (1 ) (1 ) ( ) 1 ( ) M Rx RME X E Z R e f x dx e F M . Minimizing this w.r.t R usually involves numerical techniques. To illustrate, let’s assume exponential claims with mean 1 . In this case, 1E X and MeE Z . Our equation for the adjustment coefficient becomes: 0 11 (1 ) (1 ) MM Rx x RM Me R e e dx e e ( ) ( )1 11 (1 ) (1 ) R M M R Mee R e R Minimizing R w.r.t M is again quite difficult and requires numeric techniques. 48 Chapter 5: But first, An Interlude on Bayesian Statistics Here is some introductory Bayesian Statistics that is necessary before moving on to credibility theory… For a discrete distribution: Pr( & ) Pr( | ) Pr( ) Pr( | ) Pr( )A B A B B B A A Leading to “Bayes’ Theorem” Pr( | ) Pr( )Pr( | ) Pr( ) B A AA B B For example: Suppose, 5% of individuals have cancer. If an individual has cancer, a screening will show cancer with 98% probability. If an individual does not have cancer, the test will show cancer with a 1% probability. The test on a randomly selected individual shows cancer. What is the probability that individual has cancer? A=has cancer B=test shows cancer Pr( ) 0.05A Pr( | ) 0.98B A Pr( ) Pr( | ) Pr( ) Pr( |~ ) Pr(~ ) 0.98(0.05) 0.01(.95) 0.0585B B A A B A A Pr( | ) Pr( ) 0.98(0.05)Pr( | ) 0.837606837 Pr( ) 0.0585 B A AA B B It is not 98-99%, but only 83.76%. This is important and frequently misunderstood. Another method of solving this problem is perhaps more intuitive. Suppose there are 10,000 people in the population. Given the above probabilities, 500 have cancer and 9500 do not. Of the 500 with cancer, 490 will test positive and 10 will not. Of the 9500 without cancer, 95 will test positive and 9405 will not. This can be summarized in the following table: Positive Test Negative Test Cancer 490 10 No Cancer 95 9405 The probability that an individual with a positive test actually has cancer is 490 0.837606837 490 85 as before. We’ll mostly be dealing with continuous probability distributions for the rest of this section. The above analysis extends this way … 49 The “prior distribution” is the distribution over possible parameter values ( ) . The “model distribution” is the distribution of outcomes given the parameters | ( , )xf x . The “joint pdf” is , |( , ) ( , ) ( )x xf x f x . The “marginal distribution” is , |( ) ( , ) ( , ) ( )x x xf x f x d f x d Bayes Theorem: The “posterior distribution” is || | ( , ) ( ) ( | ) ( , ) ( ) x x x f x x f x d The “predictive distribution” for the next point y is | | || ( , ) ( )y x y xf y x f y d Example: I have drawn the following data points: 0.73, 0.63, 0.98, 0.77, 1.23, 2.29, 1.24, 1.18, 6.09 and 0.03 They came from an exponential distribution with mean “2”, although we’ll pretend you don’t know the mean and instead assume that the mean is drawn from a uniform distribution between 0 and 10. That is: 1( ) 10 for 0 10 Now 10 10 11 2 | 10 1 1 1 1( , ) ... i i x xx x xf x e e e e 10 1 10 1 10 | 10 100 1 1 10( | ) 1 1 10 i i i i x x x e x e d 50 We need 10 110 100 1 1 10 i i x e d so let 21 1d d so we get… 10 18 0.1 1 10 i i x e d Now let 10 1 i i u x so we get… 10 1 8 9 0.110 1 1 10 i i u x i i u e du x 10 1 8 7 6 5 4 3 2 910 0.1 1 1 8 56 336 1680 6720 20160 40320 40320 10 i i u x i i u u u u u u u u e x Now, since 10 1 15.17i i x , the integral is 89.47626428 10 and 15.17 | 10 1,055, 268.163( | )x ex for 0 10 The predictive distribution is: ( 15.17)15.17 10 10 | 10 110 0 1 1,055,268.163 1,055,268.163| y y y x e ef y x e d d Similar substitutions lead to: 9 10 0.1( 15.17) 1,055,268.163 15.17 u y u e du y 9 8 7 6 5 4 10 1,055, 268.164 9 72 504 3024 15120 15.17 u u u u u u y 3 2 0.1( 15.17) 60480 181,440 362,880 362,880 u y u u u e 9 8 7 10 1,055,268.164 0.1 1.517 9 0.1 1.517 72 0.1 1.517 15.17 y y y y 6 5 4 3504 0.1 1.517 3024 0.1 1.517 15120 0.1 1.517 60480 0.1 1.517y y y y 2 0.1 1.517181,440 0.1 1.517 362,880 0.1 1.517 362,880 yy y e 51 The graph of the posterior distribution of looks like this: And the graph of the predictive distribution for y , the next data point, looks like this: 0 0.1 0.2 0.3 0.4 0.5 0.6 0.7 0.8 0 2 4 6 8 10 12 Posterior of Theta Posterior of Theta 0 0.1 0.2 0.3 0.4 0.5 0.6 0.7 0 2 4 6 8 10 12 Predictive Exponential 52 The “exponential” curve is assuming an exponential distribution with the same mean. And how did we get the mean, you may ask … Well … Getting it from the predictive distribution is quite hard, but we could get it from the “Law of Iterated Expectations” |E Y E E Y E And E is much easier to obtain: 15.17 15.17 10 10 10 90 0 1,055, 268.163 1,055,268.163e eE d d Similar substitutions yield: 7 8 1.517 1,055, 268.163 15.17 uu e du 7 6 5 4 3 2 8 1.517 1,055,268.164 7 42 210 840 2520 5040 5040 15.17 uu u u u u u u e 1.89596063 Improper Priors It’s OK for to *not* be a valid pdf as long as 0 everywhere and |x stays finite, as it often does. In a sense, could be viewed as being infinitely small over an infinitely large region or something like that … One choice is 1 , the diffuse prior. In this case: | | |0 ( | ) ( | ) ( | ) x x x f x x f x d The numerator is the “likelihood function” from MLE estimation. And if the denominator converges, we’re all good to go. This means the *mode* of the posterior distribution with a diffuse prior is just the MLE estimator. 53 In our example: 10 1 10 1 10 | 100 1 ( | ) 1 i i i i x x x e x e d We then do our usual substitutions on the integral in the denominator to find: 10 1 100 1 i i x e d 89 010 1 1 u i i u e du x 910 1 40,320 i i x 79.476550829 10 We then find 15.17 | 10 1,055, 236.254( | )x x e and you can check that the mode does in fact occur at 1.517. The mean is at 7 8 0 1,055, 268.163 15.17 uu e du 8 1,055,268.163(5040) 15.17 1.89625 This is 1 2 n i i x n which is not a coincidence for an exponential distribution with a diffuse prior. Another improper prior, the non-informative vague prior 1 . This is scale invariant, meaning the probability of being between 1 and 2 is the same as between 10 and 20, 100 and 200 (and 5.2 and 10.4 for that matter). Now: 10 1 10 1 10 | 100 1 1 ( | ) 1 1 i i i i x x x e x e d We then do our usual substitutions on the integral in the denominator to find: 10 1 110 1 i i x e d 910 010 1 1 u i i u e du x 1010 1 362,880 i i x 75.622212094 10 54 We then find 15.17 | 11 1,778,659.331( | )x x e The mean is at 8 9 0 1,778,659.331 15.17 uu e du 9 1,778,659.331(40320) 15.17 1.685555556 This is 1 1 n i i x n which is again not a coincidence for an exponential distribution with a non-informative vague prior. We could even choose a “crazy” prior, 100 5 . I’ll use this to introduce the concept of a “conjugate prior”. This is the prior that, when combined with an “model distribution” produces a posterior of the same form as the prior. For an exponential distribution, the “conjugate prior” is an Inverse Gamma Distribution: 1( ) xf x x e for 0x 1 E X 2 2( ) 1 2 Var X The method of moments on our prior suggests we use 402 and 40,100 . 10 1 10 1 1 1 10 | 100 1 ( | ) 1 i i i i x x x e e e x de 10 1 10 1 ( 10) 1 ( 1 0 0) 1 i i i i x x e de 10 1( 10) 110 1 0 1 10 i i x i i x e 10 1( 10) 1 1010 1 10 i i xi i x e Which is inverse gamma with 10 and 10 1 i i x . 55 In this case, 412 and 40,115.17 which gives, for the posterior distribution: 97.60381995 1 E X 2 2( ) 4.8203090861 2 Var X Here are some other conjugate priors… Model: Binomial Conjugate Prior: Beta, 11( ) 1f x x x Model: Poisson Conjugate Prior: Gamma Model: Normal with known variance Conjugate Prior: Normal Model: Uniform [0, ] Conjugate Prior: Pareto Model: Pareto with known minimum Conjugate Prior: Pareto But wait, the outcome of the “Crazy” prior still seems crazy. Do we really believe there is a 95% probability that the true value of lies between 88.6 and 107.4? Well, I could have lied about the prior, 100 5 . Which is a nice segue into Bayesian Model Selection What if you believe there is a prior probability of 1% that I lied. Use an uninformed vague prior in this case. 110.01 0.99 e 56 10 1 10 1 10 | 10 1 0 1 1 10.01 0.99 ( | ) 1 10.01 0.99 i i i i x x x e x e d e e 1010 11 11 10 ( 10) 1 1010 10 1 1 10.01 0.99 362,8800.01 0 0 .99 1 ii ii xx i i i i e x x e ( 10) 1 402 15.17 4 15.17 11 9 12 10.01 0.99 5.622217094 10 0 40,100.99 (402 4 40,115.17 03 ... 411) ee 15.1715.17 11 9 2 ( 1 1 0) 110.01 0.99 5.622217094 10 9.7053594819 10 e e 15.1715.1 10 ( 10)7 11 9 1 10 15.17 0.99 10 10 15.17 1,778,659.331 5.622217094 10 e e 15.1715.17 10 ( 101 ) 12 11 1,778,659.3310.9999999999983 1.7762528 15.1 200 7 0 1 1 0 96e e So the posterior probability of me telling the truth is 121.776252820096 10 and of me lying is 99.99999999983% . You be the judge … Here’s another, perhaps more reasonable, example. Were these data points drawn from an exponential distribution or a uniform one? Assume a vague prior 1 for both the exponential parameter and the upper limit of the uniform distribution. The model distribution is: 57 15.1710 10 1 1| 0.50 0.50f x e if 6.09 And 15.1710 1| 0.50 0.50(0)f x e if 0 6.09 | 0 1| ( | ) 1| x f x x f x d The integral in the denominator is: 15.17 10 100 6.09 1 1 1 10.50 0.50e d d 15.17 11 110 6.09 1 10.50 0.50e d d 10 7 6.09 0.50(5.622212094 10 ) 0.50 10 7 90.50(5.622212094 10 ) 0.50 1.425040062 10 72.818231247 10 This makes: 15.17 | 7 11 7 11 0.50 1 0.50 1( | ) 2.818231247 10 2.818231247 10x x e 15.17 11 11 1,774,162.431 1,774,162.431e if 6.09 15.17 | 11 1,774,162.431( | )x x e if 0 6.09 The posterior for the exponential was (from page 50) 15.17 11 1,778,659.331e and the uniform posterior is 11 116.09 1 1 d 11 701,734,657.6 so the full posterior is: | ( | ) 0.997471748(Exp Posterior) 0.002528253(Uniform Posterior)x x This is almost certainly from an exponential distribution. Of course the conclusion depends on the premises. In this case, that means the priors. This still seems pretty reasonable, since 9 of the ten observations are below the midpoint, which would be very unusual for a uniform distribution. By the way, that small chance of a uniform posterior could turn out to be important. Suppose we want the expected value of the next clam: 58 11 1 10| ...E X X X . Using the law of iterated expectations, we find this is 11 | ,E E X M where M is a second parameter that equals 1 if the distribution is exponential and 2 if the distribution is uniform. 11 | ,1E X and 11 | , 2 2E X . 11 | , | 1 Pr 1 2 Pr 22E E X M E M M E M M Now | 1 1.685555556E M from previous work. 116.09 701,734,657.62 2 2 E M d 10 6.09 350,867,328.8 d 9350,867,328.8 6.099 3.38333333 11 | , 0.997471748 1.685555556 0.002528253 3.38333333E E X M 1.689847963 . The “Bayesian Premium” is larger than 1.517 (The MLE estimate for the exponential alone) for two reasons. First, mean of the posterior exponential is not the same as the MLE estimator. Second, there is a small chance of the uniform being the correct distribution and this small chance should be taken into account. Sometimes you need a point estimate. To do this, you need a loss function ˆ, . That is, just how bad is it for being wrong? Then minimize ˆ,E given the pdf | ( | )x x . Some possibilities: 1. 2 1 ˆ ˆ, n j j j ˆj Mean 2. 1 ˆ ˆ, n j j j ˆj Median 3. ˆ, 0 if ˆj j for all j and ˆ, 1 otherwise ˆj Mode How about some other choices? 4. Profit maximizing choice: Imagine the price you charge is ˆ and the number of units you sell is ˆn D . Your profit per unit is ˆ and so your loss function (the negative of the profit) is 1ˆ ˆ, ˆ ˆ ˆn n nD D D . If we are assuming an exponential distribution for claims and the vague prior: 15.171110 1,778,659.331ˆ, ˆ ˆn nD DE e d 59 15.17 15.17 10 111 0 0 1 1,778,659.331 1,778,659.331 ˆ ˆn D e d e d 7 7 1 9.476550829 10 5.622212094 10ˆ ˆn D 7 1 1.685555555 15.622212094 10 ˆ ˆn nD We then wish to minimize this w.r.t ˆ so take the derivative and set it equal to “0”. 7 1 1.685555555 15.622212094 10 0ˆ ˆn n n nD 1.685555555 1 0ˆ n n ˆ 1.685555555 1 n n Not surprisingly, you want to overshoot a bit. 60 Now, on to Credibility Theory proper: As far as I can tell, limited fluctuation credibility and greatest accuracy credibility are not on the CT6 syllabus. Therefore we will only deal with the Bayesian approach to credibility… We’ll say the expected value of the next claim is (1 )ZX Z M where X is the mean of the data and M is some prior belief or expectation, sometimes called the “Manual Premium”. We’ll justify this in a moment as well as determine the value of Z . Let’s take a quick example. Assume claims follow a Poisson distribution and our prior distribution for the parameter is a gamma distribution 1 e . In other words, this is the conjugate prior to the model distribution. If you have data that says n individuals produce j claims then the Bayesian conjugate prior rules imply the posterior distribution is also gamma with * j and * n . The law of iterated expectations implies that, prior to knowing the data, |i iM E X E E X E And after the Bayesian updating: * 1 1 *|n n jE X E E X E n To find Z , we solve: 1j jZ Z n n After some algebra, this solves for: nZ n . For another way of looking at this, you could view the situation as one where you already have claims for individuals and just add the new claims in. Example: Suppose 20 and 2,000 so the “Manual Premium” 0.01M . This means 2,000 nZ n . Now suppose your company has 1,000 policies and sees 17 claims. 1,000 1 1,000 2,000 3 Z so 1 1 2(0.017) (0.010) 0.0123333333 3nE X Alternatively, you could view this as a prior experience of 20 claims in 2,000 policies. You now have 37 claims in 3,000 policies and 1 37 0.0123333333,000nE X . 61 The Poisson/Gamma model/prior distributions are not the only ones with this property. In fact, there is a large class of models and conjugate prior distributions that work this way. Enough to justify this technique fairly broadly. For instance, suppose | ( , ) r x x p x e f x q and ( ) , k krq e r C k . This is called the “Linear exponential Family”. The posterior distribution has the same form: * * * | * * ( ) , k k r x q e r C k with *k k n and * n kX n k n k . I won’t prove it here, perhaps in an appendix later. Even more interestingly, the mean of the posterior predictive distribution follows the form 1 1n jE X Z Z Mn where M is the mean of the prior predictive distribution and 0 0 nZ n k where 0 kn M . Again, the proof is beyond the scope of the class but perhaps will appear in an appendix later. Our Poisson/Gamma model is of this type. If 1 ! p x x , q e and lnr we have: ln | 1 !( , ) ! x x x e exf x e x which is Poisson. The Prior is ( ) , k krq e r C k ln 1 , k ke e C k 1 , k ke C k . If we set k , and , k k C k k we recover the Gamma prior! Here’s another model of this type. If 1p x , 1q and r we have: | 1( , ) 1 x x x ef x e which is exponential. The Prior is ( ) , k krq e r C k , k ke C k . If we set 1k , 1 and 1 1 , k k C k k we recover the Gamma prior! 62 The Exponential/Inverse Gamma Model seen previously is also of this type (with the exponential parameter defined differently. If 1p x , q and 1r we have: | 1 1( , ) x x x ef x e which is exponential. The Prior is ( ) , k krq e r C k 2 1 , k ke C k ( 2) , k k e C k . If we set 1k , 1 and 1 1 , k k C k k we recover the Inverse Gamma prior: 1( ) xf x x e We’ve already seen an example of the Poisson/Gamma model. Let’s create an example of the exponential/gamma model and apply it to our Exponential/Gamma model. Let’s assume our ten data points: 0.73, 0.63, 0.98, 0.77, 1.23, 2.29, 1.24, 1.18, 6.09 and 0.03 And a manual premium of 2M . This would be the mean of the prior predictive distribution. By the law of iterated expectations, 1| 1 E X E E X E . Let’s set 2 for a relatively wide distribution. This means 1 1k and 2 1 . Using our conjugate prior methodology, we find * 11k k n and * 10 1(1.517) (2) 1.560909091 10 1 11 . This gives * * 1 12k , * * * 17.17k and, by the law of iterated expectations, * 1 * 1| 1.560909091 1n E X E E X E . Or, we could compute this directly as 1 2 15.17 1.5609090911 10nE X If we set 101 , 200 for a relatively tight distribution. This means 1 100k and 2 1 . Using our conjugate prior methodology, we find * 110k k n and * 10 100(1.517) (2) 1.956090909 10 100 110 . This gives * * 1 111k , * * * 215.17k and, by the law of iterated expectations, * 1 * 1| 1.956090909 1n E X E E X E . Or, we could compute this directly as 1 200 15.17 1.956090909100 10nE X 63 Another Member of the Linear Exponential Family is the Normal distribution with a Normal conjugate prior. Suppose our model distribution is normal with known standard deviation 1 but unknown mean (the parameter . If 2 2 12 1 2 x ep x , 2 2 12q e and 2 1 r we have: 2 2 1 2 1 2 2 2 2 2 1 1 2 2 1 2 2 2 21 | 2 1 1 2 1 1( , ) 2 2 x x x x x x e e f x e e e which is normal, as we want. The Prior is ( ) , k krq e r C k 2 2 2 1 12 2 1 1 , k k e e C k . If we set 2 1 2 2 k , and 2 2 222 2 1 , 2C k e we recover the Normal prior with mean and standard deviation 2 ! Given the number of models that belong to the linear exponential family, the general methodology of Assume a “Manual Premium” and a number of observations that produced it as sufficient to represent your prior, then use this linear method will be a very good approximation to the Bayesian truth. This leads us to the Empirical Bayes Model. This feels like magic to me, but this is a way of determining Bayesian credibility estimates with no prior at all, just the data, as long as you have multiple policies that might have different values of the underlying parameter. Let’s start with some preliminaries… Suppose you wish to construct the “Best Linear Estimator” of the mean of the predictive distribution 1n conditional on the data. We’ll try to minimize the expected squared error, that is: 2 1 0 1 n n j j j Q E a a x We solve the following equations: 1. 1 0 10 2 0 n n j j j dQ E a a x da 1 1 0 1 n n n j j j E E X a a E X from the law of iterated expectations 64 2. 1 0 1 2 0 n i n j j ji dQ E x a a x da 1 1 0 1 n i n i n i j i j j E X E X X a E X a E X X again from the law of iterated expectations. 3. Now, if we multiply The first boxed equation by iE X and subtract it from the second one, we find: 1 1 , , n i n j i j j Cov X X a Cov X X The first and third boxed equations can be solved to give a credibility premium. For example, let’s assume the jX are drawn from a joint normal distribution with jE X , 2jVar X and ,i jCov X X . None of the following analysis depends on normality, though. The first equation implies 0 1 n j j a a or 0 1 1 n j j aa . The third equation implies 1 1 n i j j a a for each value of 1...i n . This means 1 0 1 1 1 n j j i a aa . If we put that back into the first equation, we find: 0 0 1 aa n . This solves for 0 1 1 a n and therefore 1i a n for 1...i n . Now, 1 0 0 1 | ,..., n n n j j j E X x x a a x 1 1 1 1 n j j x n n 1 1 1 n X n n (1 )ZX Z Where 65 1 nZ n 1 n n n n k with 1k . There’s that functional form again … The Bühlmann Model: Let 0 ,..., nX X be i.i.d. conditional on some parameter . Define: |jm E X ”hypothetical mean” 2 |js Var X ”process variance” We can actually use this information to evaluate the “Best Linear Estimator” above. |j jE X E E X E m | |j j jVar X E Var X Var E X 2E s Var m ,i j i j j jCov X X E X X E X E X 2|i jE E X X E m 2| |i jE E X E X E m 22E m E m Var m ,i j i j Cov X X Var X Var X 2 Var m E s Var m 1k 1 1 2 1 E s Var m Var m 2E s Var m . In the case of the linear exponential family, this is not just the best linear estimator, but exactly correct! Now, if 2E s Var m this means the data tends to have more variability than the prior belief. Therefore, k is larger and Z is smaller, causing more weight to be put on the prior than on the data. Conversely, if 2E s Var m this means the data tends to have less variability than the prior belief. Therefore, k is smaller and Z is larger, causing more weight to be put on the data than on the prior. Here’s a quick way of thinking about it intuitively in the limits. Suppose you have a prior Pr 1 0.5 and Pr 2 0.5 . The model distribution is iX with 100% probability. You either see data of 1,1,1,1,1... or 2, 2, 2, 2, 2... with 50% probability. 1.5iM E X . 1 , 2 0s for both values of so 2 0E s . 0.25Var m and 0k . All the weight is on the means of the observed data, as it should be. The data carry complete information and the prior is useless once you see the data. 66 On the other hand, Suppose you have a prior Pr 1 1 and a model distribution where Pr 0.5iX and Pr 2 0.5iX . Your data will be a random and uncorrelated sequence of 1s and 2s. 1.5iM E X . 0 , 2 0.25s so 2 0.25E s . 0Var m and k . All the weight is on the prior “ 1 ” as it should be. The data carry no information. The method above is called “Buhlmann Credibility”. This method is exact for members of the linear exponential family. We can recover the models in this way: Normal/Normal: 2 21E s , 22Var m ; 2 2 1 2 2 E s k Var m ; |iM E E X E Poisson/Gamma: 2E s E ; 2( )Var m Var ; 2E s k Var m ; |iM E E X E Exponential/Gamma: 1 2 2 20 1 1 eE s E d 3 0 e d 2 2 2 1 2 ; 1Var m Var 2 2 1 1E E 22 1 0 1 1 2 e d 22 2 01 2 e d 22 1 1 1 2 22 1 2 1 2 2 2 2 1 2 2 1 1 2 22 1 ; 2 1 E s k Var m ; |iM E E X 1E 1 Exponential/Inverse Gamma: 2 2E s E 2E Var 2 2 21 2 1 2 2 2 2 2 2 1 2 1 2 1 2 ; Var m Var 2 22 1 ; 2 1 E s k Var m ; |iM E E X E 1 67 Let’s work out an example where the Bayesian estimate and the Buhlmann estimate are different. We’ll use something similar to our previous example where Pr 1 0.5 and Pr 2 0.5 , but the model distribution is Pr 1 0.1iX , Pr 0.8iX and Pr 1 0.1iX . This is pretty informative data. We’ll then assume that we see “1,1,1” as the first three data points. The Bayesian process would go this way: The posterior 0.5(0.8)(0.8)(0.8)Pr 1 0.5(0.8)(0.8)(0.8) 0.5(0.1)(0.1)(0.1) 0.2560 0.2565 0.998050682 And 0.5(0.1)(0.1)(0.1)Pr 2 0.5(0.8)(0.8)(0.8) 0.5(0.1)(0.1)(0.1) 0.0005 0.2565 0.001949318 The data is very informative, but not quite as information as in our previous example. 4 4 |E X E E X E 1.001949318 . The Buhlmann process would go like this: 2 0.2s in both instances, so 2 0.2E s . 0.25Var m as before. 2 0.20 0.8 0.25 E s k Var m ; 3 0.789473684 3 0.8 Z ; 1.5M E m and 4 (1) (1 )(1.5) 1.105263158E X Z Z . The Bayesian answer is the correct one in this case since we know more information that just 2E s and Var m . The Buhlmann answer is incorrect since the model/prior distributions are not from the linear exponential family. However, the Buhlmann answer is the best we can do with a linear combination of the data in the sense that 20 1 1 2 2 3 3 4 1 2 3| , ,E a a X a X a X E X X X X is minimized ex ante. Next, we need some good estimates of 2E s and Var m when the model distributions and/or priors are not known. It’s possible to estimate these from the data itself in the following manner. Assume there are r policies with n observations each. I won’t prove it, but 2 1 1 1ˆ 1 r n ij i i j v X X r n is an unbiased estimator for 2E s and 2 1 ˆ1ˆ 1 r i i va X X r n is an unbiased estimator for Var m . If aˆ is negative, it is customary to set 0Z . Let’s add two more policyholders to our sample set: PH1: 0.73, 0.63, 0.98, 0.77, 1.23, 2.29, 1.24, 1.18, 6.09, 0.03 68 PH2: 2.57, 5.36, 14.52, 3.14, 3.93, 0.27, 2.96, 5.00, 2.88, 2.24 PH3: 1.72, 4.77, 0.93, 3.94, 5.12, 3.07, 1.99, 3.66, 0.96, 1.12 I drew these numbers from exponential distributions with means 2, 6, and 4 but let’s pretend we don’t know that yet. All we know are the data points. We have 1 1.517X and the unbiased estimator for the variance of PH1 is : 10 2 1 1 1 1 2.916245555 9 jj X X . Also 2 4.287X and the unbiased estimator for the variance of PH2 is : 10 2 2 2 1 1 14.18935666 9 jj X X . Finally 3 2.728X and the unbiased estimator for the variance of PH3 is : 10 2 3 3 1 1 2.538995555 9 jj X X . 3 10 2 1 1 1ˆ 6.812199259 3 9 ij ii j v X X 3 2 1 ˆ1 6.812199259ˆ 1.928317 1.247097074 2 10ii va X X n And ˆ 5.462445066 ˆ vk a giving 10 0.646728248 10 Z k The Best Linear estimates of the next data points are: PH1: 0.646728248(1.517) (1 0.646728248) 2.8 1.985794 64 1 16 PH2: 0.646728248(4.287) (1 0.646728248) 2.8 3.777224 84 8 61 PH3: 0.646728248(2.728) (1 0.646728248) 2.8 2.768974 54 9 23 This is the “Non-parametric” model. We can compare it to some other possibilities. For instance, the “Semiparametric” model where |if x is known, but is unknown. Then, instead of estimating vˆ from the data, we estimate it from a model fit. Our examples are exponential, so the mean and standard deviation are equal. The three estimated variances are now 21.517 2.301289 , 24.287 18.378369 and 22.728 7.441984 . 1ˆ 2.301289 18.378369 7.4416984 9.373880667 3 v 3 2 1 ˆ1 9.373880667ˆ 1.928317 0.990928933 2 10ii va X X n 69 And ˆ 9.459690147 ˆ vk a giving 10 0.513882796 10 Z k The Best Linear estimates of the next data points are: PH1: 0.513882796(1.517) (1 0.513882796) 2.8 2.162074 54 7 28 PH2: 0.513882796(4.287) (1 0.513882796) 2.8 3.585534 84 2 76 PH3: 0.513882796(2.728) (1 0.513882796) 2.8 2.784384 54 9 96 A “Fully Parametric” model assumes both |if x and are known and uses the “Best Linear Estimator” technique. Let’s assume is 1/3 chance of 2, 1/3 chance of 4 and 1/3 chance of 6. We then get: 1ˆ 4 16 36 18.66666667 3 v 21 1ˆ 4 16 36 2 4 6 2.666666667 3 3 a And ˆ 7 ˆ vk a giving 0.51 80 1 8235 4 0 29Z k The Best Linear estimates of the next data points are: PH1: (1.517) (10.588235294 0.588235294 2.539411765) 4 PH2: (4.287) (10.588235294 0.588235294 4.168823529) 4 PH3: (2.728) (10.588235294 0.588235294 3.251764706) 4 A complete Bayesian approach would work like this. We need the posterior for all three policyholders: 10 1 10 1| i i x f x e PH1: 15.17 7210 1Pr | 2 4.961081917 10 2 x e 15.17 7410 1Pr | 4 0.214950403 10 4 x e 15.17 7610 1Pr | 6 0.013196121 10 6 x e 70 So: 71 3 7 7 71 3 4.961081917 10 Pr 2 | 0.9560134596 4.961081917 10 0.214950403 10 0.013196121 10 x 71 3 7 7 71 3 0.214950403 10 Pr 4 | 0.041422421 4.961081917 10 0.214950403 10 0.013196121 10 x 71 3 7 7 71 3 0.013196121 10 Pr 6 | 0.002542983 4.961081917 10 0.214950403 10 0.013196121 10 x And 11 0.9560134596(2) 0.041422421(4) 0.002542983(6) 2.093016776E X PH2: 42.87 11210 1Pr | 2 0.04792894 10 2 x e 42.87 11410 1Pr | 4 2.112754216 10 4 x e 42.87 11610 1Pr | 6 1.304528054 10 6 x e So: 111 3 11 11 111 3 0.04792894 10 Pr 2 | 0.013831463 0.04792894 10 2.112754216 10 1.304528054 10 x 111 3 11 11 111 3 2.112754216 10 Pr 4 | 0.609704312 0.04792894 10 2.112754216 10 1.304528054 10 x 111 3 11 11 111 3 1.304528054 10 Pr 6 | 0.376464225 0.04792894 10 2.112754216 10 1.304528054 10 x And 11 0.013831463(2) 0.609704312(4) 0.376464225(6) 4.725265525E X PH3: 27.28 9210 1Pr | 2 1.163920481 10 2 x e 27.28 9410 1.04114 1Pr | 62044 10 4 x e 27.28 9610 0.17534 1Pr | 57536 10 6 x e So: 71 91 3 9 9 91 3 0.488957486 1.041146204 1.1639 0.1 20481 10 Pr 2 | 1.163920481 10 10 1075345753 x 91 3 9 9 91 3 1.041146204 0.437380593 1 10 Pr 4 | 1. .041146204 0.17534575163920481 10 10 13 0 x 111 3 9 9 91 3 1.304528054 10 Pr 6 | 1.163920481 10 10 0.073661921 1.041146204 0.175345753 10 x And 11 0.488957486 0.437380593 0.073661(2) (4) (921 3.1694088716)E X Next Topic: Bühlmann-Straub Credibility. There are two ways real world data might be different from what we’ve assumed above. First, the number of data points may not be the same. Second, the “volume” giving rise to the claims may be different for different policies. In these case, you can view ijP as the “volume” on policy i in period j . This could be due to differing number of risks (on say a rental car fleet) or different lengths of time (say months vs. years). Let 1 in i ij j P P and 1 N i i P P . Let 1 in ij i ij j i P X X P and 1 1 inN ij ij i j P X X P . The Bühlmann-Straub best estimator for , 1ii nX is given by: 1iZX Z X with 1 1 ˆ ˆ i i n ij j n ij j P Z vP a We need new estimators for vˆ and aˆ . They are now: 2 1 1 1 ˆ 1 inN ij ij i i j N i i P X X v n 1 2 21 1 ˆ ˆ 1 N i N i i i i P a P P X X v N P See Loss Models, From Data to Decisions, 4th Edition (Klugman et al 2012) pg. 424. The Boland book and CT6 course readings are correct as well. They can be shown to be equivalent, which I will do in Appendix A. 72 Let examine our two cases in turn. First, the number of data points might not be the same for all policies. Imagine the data above, except that PH2 has only 8 observations (the final values of 2.88 and 2.24 are missing) and PH3 has only 6 values (the final values of 1.99, 3.66, 0.76 and 1.12 are missing). We can still get unbiased means and variances for each PH. We have 1 1.517X and the unbiased estimator for the variance of PH1 is : 10 2 1 1 1 1 2.916245555 9 jj X X . Also 2 4.71875X and the unbiased estimator for the variance of PH2 is : 8 2 2 2 1 1 18.16729821 7 jj X X . Finally 3 3.25833333X and the unbiased estimator for the variance of PH3 is : 10 2 3 3 1 1 2.807736667 5 jj X X . 1 1 10(1.517) 8(4.71875) 6(3.2583333) 2 3.0195833 4 33 inN ij ij i j P X X P 167.4559808ˆ 7.974094324 9 7 5 v 12 2 2 2.257756674 2.887167310 8 6ˆ 24 10 8 661 0.05700156 2 7.974094324 24 3a 1.91928ˆ 0405a 4.154 ˆ ˆ 731275vk a giving: 1 0.7064775594.1547311 275 10 0 Z 2 0.6581799154.1547312 8 758 Z 3 0.5908575854.1547312 6 756 Z If we use the actual mean of the data, we find the credibility premiums to be: 0.706477559 0.706477559 3.01958PH1: 3333 (1. 1.517) ( 951 ) 8041928 0.658179915 0.658179915 3.019PH2: (4.71875) ( 583333 4.13794 01 ) 07 6 0.590857585 0.5908575PH3: (3.25833333) (1 )85 3.019583333 3.160650582 73 There is one potential pitfall with the approach above. Namely, that the Total Credibility Premium would not match the historical losses. In our example, the total credibility premium would be: 10 1.958041928 8 4.137940706 6 3.160650582 71.64784842 And the total historical losses would be 72.47 . This was not the case when the total exposure on all three policies was the same. When we included all thirty data points, the total credibility premium was: 10 1.985791616 10 3.777228861 10 2.768979523 85.32 , which is the same as the total losses. If necessary, this problem can be solved by using the “Credibility Weighted Mean” of the data in place of the actual mean. For our example, the credibility weighted mean is: (1.517) (4.71875) (3.20.706477559 0.65817991 0.590857585 3.120775709 0.706477559 0.65817991 0.590857585 583333) This gives credibility premium for the three policyholders of: 0.706477559 0.706477559 3.12077PH1: 5709 (1. 1.517) ( 981 ) 7744161 0.658179915 0.658179915 3.120PH2: (4.71875) ( 775709 4.17253 91 ) 02 3 0.590857585 0.5908575PH3: (3.25833333) (1 )85 3.120775709 3.202052675 And the total historical premium would be: 10 1.987744161 8 4.172530293 6 3.202052675 72.47 in agreement with total historical losses. Now let’s try a case where the weights are unequal. We’ll assume the 10 data points for PH1 are all for one risk. The 8 data points for PH2 will be combined into 4 values for two risks each, with values 7.93, 17.66, 4.20 and 7.96. The 6 data points for PH3 will be 3 risks with 7.42, 2 risks with 9.06 and 1 risk with 3.07. Now what? 10 10 1 1 1 1 1 11 1 1.517 10 j j j j j P X X X P 4 4 2 2 1 2 1 12 7.93 17.66 4.20 7.96 2 2 2 2 2 4.71875 8 4 j j j j j P X X X P 3 3 3 1 1 3 7.42 9.06 3.073 2 3 2 1 3.258333333 6 j j j P X X P 74 1 1 inN ij ij i j P X X P 1 7.93 17.66 4.20 7.96 7.42 9.06 3.0715.17 2 2 2 2 3 2 24 2 2 2 2 3 2 1 3.019583333 These are the same numbers as before… 2 1 1 1 ˆ 1 inN ij ij i i j N i i P X X v n 2 2 210 42 2 1 1 2 2 3 3 3 1 1 7.42 9.06 3.072 3 2 3 2 1 9 3 2 j j j j X X X X X X X 14 81.11286417 5.793776012 1 2 21 1 ˆ ˆ 1 N i N i i i i P a P P X X v N P 12 2 2 2.257756674 2.887167361 0.057001563 5.7937760110 8 624 1 20 8 6 2 P 2.197618913 2.636 ˆ ˆ 387945vk a giving: 1 0.7913653842.6363871 945 10 0 Z 2 0.7521350332.6363879 8 458 Z 3 0.6947348872.6363879 6 456 Z If we use the actual mean of the data, we find the credibility premiums to be: 0.791365384 0.791365384 3.01958PH1: 3333 (1. 1.517) ( 831 ) 0490897 0.752135033 0.752135033 3.019PH2: (4.71875) ( 583333 4.29758 11 ) 61 1 0.694734887 0.6947348PH3: (3.25833333) (1 )87 3.019583333 3.185451288 75 We do have the same problem as before. The total historical premium would have been 10 1.830490897 8 4.297586111 6 3.185451288 71.79830558 which is different from the historical losses of 72.47. This problem can again be solved by using the credibility-weighted mean (1.517) (4.71875) (3.20.791365384 0.752135033 0.694734887 3.13341332 0.791365384 0.752135033 0.694734887 583333) This gives credibility premium for the three policyholders of: 0.791365384 0.79PH1: (1.517) (1 )1365384 3.13341332 1.854239772 0.752135033 0.752135033 3.13PH2: (4.71875) 341332 4.3(1 2 5) 5800 76 0.694734887 0.694734887 3PH3: (3.2583 .13341332 33 .333) 2201( 996111 ) And the total historical premium would be: 10 1.854239772 8 4.325800576 6 3.220199611 72.47 in agreement with total historical losses. 76 Chapter 7: Generalized Linear Models Let’s begin by revisiting the concept of the “Exponential Family” of distributions. A distribution belongs to an exponential family if: ,( , , ) x b c x a Xf x e We’ve already met the “Linear Exponential Family”: ( )( )( , ) ( ) r x X p xf x e q How is this the same, you might ask? Well, the parameters are actually defined differently. Let’s rename in the linear exponential family as L . If we define Lr and 1L r the “Linear Exponential Family” is reparameterized as: 1 ( )( , ) xX p xf x e q r We also make sure the functions 1a and , ( )c x d x are independent of giving ( , , ) x b d xXf x e Now we can see ( ) ln ( )d x p x and 1( ) lnb q r . We can now recharacterize our specific linear exponential family distributions in this way. For example: 1. The Poisson distribution. We found 1 ! p x x , q e and lnr . We now have 1a , ( ) ln eb e e and ( , ) ln ( ) ln !c x p x x . is now defined as lnLr . 2. The exponential distribution. We found 1p x , 1q and r . We now have 1a , 1( ) ln lnb and ( , ) ln ( ) 0c x p x . is now defined as Lr . We’ll save the normal distribution for later, since we can now use both parameters effectively. 77 This form is nice, since it can be shown that E X b and Var X a b . That is, the mean only depends on the parameter and affects the variance. For the Poisson distribution, lnE X b e e as expected, and ln1( )Var X a b e e , again as expected. For the exponential distribution, 1 1 1( 1)E X b as expected, and 2 2 1 11Var X a b as expected. Given the additional parameter we can move out of the “linear exponential family” and show that a number of two parameter distributions belong to the exponential family. For example: 1. The binomial distribution. First, we need to transform the regular binomial variable Z into the a new random variable ZX n , the “proportion of success” rather than the “number of successes”. We find ln 1 p p , n , 1( )a , ( ) ln 1b e , and ( , ) lnc x x . Therefore ln 1ln 1 ln1 ( , , ) x e x n X p p f x e l ln 1 ln n1 pp nn x n e nxe 1 1 1 z n p p p p n z 1 1 1 z n np p p z 1 nz zp z p n ! 1 11 1 p e pE X b p e p and 2 2 1 1 (1 )1( ) 11 1 p e p ppVar X a b n n ne p ! Alrighty then. 78 2. The normal distribution. We find , 2 , ( )a , 2 ( ) 2 b , and 21( , ) ln 2 2 xc x . Therefore 2 2 2 2 1 ln 2 2 ( , , ) x x Xf x e 2 2 2 2 2 2 1 2 1 ln 2 2 x x e e 2 2 2 2 2 2 1 2 x x e 2 2 ( ) 2 2 1 2 x e ! E X b and 2 2( ) (1)Var X a b ! 3. The gamma distribution. We find , , 1( )a , ( ) ln( )b , and ( , ) ( 1) ln ln lnc x x . Therefore ln ( 1)ln ln n1 l ( , , ) x x Xf x e ln ln( 1) ln lnx xe e e e e 1xe x 1 xx e ! 1 1( 1)E X b and 2 2 1 1( )Var X a b ! Where are we going with all this? Glad you asked. What we are doing is attempting to generalize the concept of “Linear Regression”. By way of review, Linear Regression works like this: You have a set of “Dependent Variables” iy that relate in some way to a set of dependent variables 1ix , 2ix , … , ipx . Assuming a linear dependence, we have 0 1 1 2 2 ...i i i p ip iy x x x . You then attempt to minimize the sum of the squared deviations between the dependent variable and the predictor. If the i are i.i.d. normal then OLS gives the same answer as the MLE estimates of the i parameters. Saying the i are i.i.d. normal is equivalent to saying that the iy are drawn from a normal distribution with mean 0 1 1 2 2 ...i i p ipx x x and variance 2 . Generalized Linear Models generalizes this procedure when the dependent variable can be assumed to be drawn from any distribution in the Exponential Family! 79 The “link” is through the canonical link function, which relates the parameter to the mean of the distribution. Remember, b so you get the “canonical link function” for a particular distribution by solving this for . For the “normal” model, we find E X b so the canonical link function is and you find the MLE estimates of the parameters where each iy is drawn from a normal distribution with mean 0 1 1 2 2 ...i i i p ipx x x and variance i . This is just ordinary least squares. If i is not constant, it is a weighted least squares regression. If, instead, you assume that iy values the proportions drawn from a binomial distribution, 1 eE b p e X which solves for 1e ep 1e p p ln 1 p p is the canonical link function. We then find the MLE estimates of the parameters where each iy is drawn from a binomial distribution with 0 1 1 2 2 ..n 1 .l i i p ipi i x xp p x and in known. This is the common “logit” model. If, instead, you assume that iy values the proportions drawn from a Poisson distribution, E X b e which solves for ln as the canonical link function. We then find the MLE estimates of the parameters where each iy is drawn from a Poisson distribution with 0 1 1 2 2 ...ln i p ipi ix x x . FInally, if you assume that iy values the proportions drawn from a gamma distribution (of which the exponential is a subset) 1E X b which solves for as the canonical link function. We then find the MLE estimates of the parameters where each iy is drawn from a Poisson distribution with 0 1 1 2 2 ...i i p ip i x x x . It is not necessary to use the “canonical link function”. However, the MLE math is “easier” in some sense if you do. There’s not necessarily any reason to believe that the particular link function is linear in the parameters in any particular case though. You could, in the most general case use the canonical link function and a non-linear function of the parameters and dependent variables (assuming you believe the iy values are actually random draws from the particular member of the exponential family that corresponds to that particular link function). How the mathematics easier? I’m glad you asked… 80 The log-likelihood function for a member of the Linear Exponential family is easy to write: 1 , n i i i i i i i y b c y a The MLE process would be to take the derivatives with respect to the parameters and set them equal to zero. I’ll define 0 1ix so the equations are easier to write out. We get 1p equations: 1 1 0 i i i in n j j i ij i ij i ij i i y b y x x a a since we are using the canonical link function. Let’s do some examples in turn. First, assume a normal distribution with constant variance 2 , so 2 1 0 n i ip i ip i y x x 1 1 n n i ip i ip i i y x x . In the normal model, 0 0 1 1 2 2 ...i i i i p ipi x x x x and we recover the usual OLS equations: 1 0 1 pn n i ij k ik ij i k i y x x x Suppose we have the following table of ijx values and iy outcomes for 6 observations: i 1ix 2ix iy 1 1 2 0.11 2 3 7 0.29 3 6 4 0.25 4 2 2 0.11 5 5 1 0.13 6 0 1 0.02 There are two parameters so there are three equations: 1) 6 6 6 6 0 0 0 0 1 1 0 2 2 0 1 1 1 1 i i i i i i i i i i i i y x x x x x x x 2) 6 6 6 6 1 0 0 1 1 1 1 2 2 1 1 1 1 1 i i i i i i i i i i i i y x x x x x x x 3) 6 6 6 6 2 0 0 2 1 1 2 2 2 2 1 1 1 1 i i i i i i i i i i i i y x x x x x x x Or, with numbers involved: 81 1) 0 1 20.91 6 17 17 2) 0 1 23.35 17 75 56 3) 0 1 23.62 17 56 75 You then solve the simultaneous equations to get: 0 0.003461538 ; 1 0.019048583 ; 2 0.033259109 Now suppose instead that the variances were different, that is 2i ia with 1 0.06 ; 2 0.05 ; 3 0.04 ; 4 0.03 ; 5 0.02 ; 6 0.01 . This will reproduce the “weighted least squares” methodology. The equations are now: 2 2 1 0 1 pn n i ij ik ij p i k ii i y x x x . This gives us three equations: 1) 0 1 2950.0277778 14913.88889 19950 20577.77778 2) 0 1 23185.5 19950 93322.22222 40900 3) 0 1 22267.555556 20577.77778 40900 47655.55556 Which solve for: 0 0.013108406 ; 1 0.021803414 ; 2 0.034529821 , similar but not identical. The difference in variances can be interpreted as a difference in the number of observations. For instance, if there were 100 observation in class 1 with 11 claims, 200 observations in class 2 with 58 claims, 300 observations in class 3 with 75 claims, 400 observations in class 4 with 44 claims, 500 observations in class 5 with 65 claims and 600 observations in class 6 with 12 claims, the means would be the same as reported in the table, but 2 2 100i i a i . The equations are now: 2 2 1 0 1 pn n i ij ik ij p i k ii i y x x x 2 2 1 0 1100 100 pn n i ij ik ij p i k i iy x ix x 1 0 1 pn n i ij p ik ij i k i iy x ix x This gives us three equations: 1) 0 1 22.65 21 58 47 2) 0 1 210.48 58 268 157 3) 0 1 28.93 47 157 177 Which solve for: 82 0 0.007044563 ; 1 0.020770073 ; 2 0.033899395 , again similar but not identical to the previous cases. All three of the above models imply an MLE estimate of the means of the 6 types of policyholders. This could also be viewed as the expected value of the next observation. Since all of the models above have 0 0 1 1 2 2 ...i i i i p ipi x x x x the means are easily found in the following table: i 1ix 2ix iy i Model 1 i Model 2 i Model 3 1 1 2 0.11 0.089028 0.077755 0.081524 2 3 7 0.29 0.293421 0.294011 0.292561 3 6 4 0.25 0.250789 0.255831 0.253173 4 2 2 0.11 0.108077 0.099558 0.102294 5 5 1 0.13 0.131964 0.130438 0.130705 6 0 1 0.02 0.036721 0.021421 0.026855 Things get a little trickier when using a different linear-exponential family. For instance, the binomial (logit) model uses: 1 0 n i ij i ij i i y x x a where ln 1i p p , 1( ) i a n and 1 i ii e p e . The canonical link function looks like 0 0 1 1 2 2ln ...1i i i i p ip p x x x x p . This creates the set of 1p equations: 1 1 1 i i n n i i ip i ip i i en y x n x e They are non-linear, but can be solved using an iterative least squares process … Let *p p p where *p is an initial estimate and p is small. A Taylor expansion for 1 i i e e can now be found which is linear in the p values: * * * *0 0 1 1 2 2 0 0 1 1 2 2... ...i i i i p ip i i i p ipx x x x x x x x * * * *0 0 1 1 2 2 0 0 1 1 2 2... ...i i i p ip i i i p ipi x x x x x x x xe e e * * * *0 0 1 1 2 2 ... 0 0 1 1 2 21 ...i i i p ipx x x x i i i p ipe x x x x And * * * *0 0 1 1 2 2 ... 0 0 1 1 2 21 1 1 ...i i i p ipi x x x x i i i p ipe e x x x x * * * * * * * *0 0 1 1 2 2 0 0 1 1 2 2... ... 0 0 1 1 2 21 ...i i i p ip i i i p ipx x x x x x x x i i i p ipe e x x x x 83 * * * * 0 0 1 1 2 2* * * * 0 0 1 1 2 2 * * * * 0 0 1 1 2 2 ... ... 0 0 1 1 2 2... 1 1 ... 1 i i i p ip i i i p ip i i i p ip x x x x x x x x i i i p ipx x x x ee x x x x e Therefore 1 i i e e * * * * 0 0 1 1 2 2 * * * * 0 0 1 1 2 2* * * * 0 0 1 1 2 2 * * * * 0 0 1 1 2 2 ... 0 0 1 1 2 2 ... ... 0 0 1 1 2 2... 1 ... 1 1 ... 1 i i i p ip i i i p ip i i i p ip i i i p ip x x x x i i i p ip x x x x x x x x i i ix x x x e x x x x ee x x x e p ipx * * * * 0 0 1 1 2 2 * * * * * * * * 0 0 1 1 2 2 0 0 1 1 2 2 * * * * 0 0 1 1 2 2 ... 0 0 1 1 2 2 ... ... 0 0 1 1... 1 ... 1 1 1 i i i p ip i i i p ip i i i p ip i i i p ip x x x x i i i p ip x x x x x x x x i ix x x x x x x xe e e x x e 2 2 ...i p ipx x * * * * 0 0 1 1 2 2 * * * * 0 0 1 1 2 2 ... 0 0 1 1 2 2... 1 ... 1 i i i p ip i i i p ip x x x x i i i p ipx x x x e x x x x e * * * * 0 0 1 1 2 2 * * * * 0 0 1 1 2 2 ... 0 0 1 1 2 2... 1 ... 1 i i i p ip i i i p ip x x x x i i i p ipx x x x e x x x x e * * * * 0 0 1 1 2 2 * * * * 0 0 1 1 2 2 ... 0 0 1 1 2 2... 1 ... 1 i i i p ip i i i p ip x x x x i i i p ipx x x x e x x x x e * * * * 0 0 1 1 2 2 * * * * 0 0 1 1 2 2 ... 0 0 1 1 2 2... ... 1 i i i p ip i i i p ip x x x x i i i p ipx x x x e x x x x e * * * * 0 0 1 1 2 2 * * * ** * * * 0 0 1 1 2 20 0 1 1 2 2 ... 0 0 1 1 2 2...... 11 ... 11 i i i p ip i i i p ipi i i p ip x x x x i i i p ipx x x xx x x x e x x x x ee Another way of looking at this is: * * * * 0 0 1 1 2 22 ... 1 1 1 i i i i i i i i i p ip e e e x x x x e e e Or * * 0 0 1 1 2 2* ... i i i i i i p ip i x x x x 84 This leads to 1p interlocking linear equations for the values of k * * * * * * * * 0 0 1 1 2 2 0 0 1 1 2 2 * * * * * * * *0 0 1 1 2 2 0 0 1 1 2 2 ... ... 2..1 1 0 1. ...1 1 i i i p ip i i i p ip i i i p ip i i i p ip pn n n i i ij ij k i ik ij i i k x x x x x x x x ix x x x x x xi x e enn y x e e x n x x Or * * * * * * * * 0 0 1 1 2 2 0 0 1 1 2 2 * * * * * * * *0 0 1 1 2 2 0 0 1 1 2 2 ... ... 2..1 1 0 1. ...1 1 i i i p ip i i i p ip i i i p ip i i i p ip pn n n i i ij ij k i ik ij i i k x x x x x x x x ix x x x x x xi x e enn y x e e x n x x Or: * 0 1 * * 1 1i i i pn n i i ij k i ik ij i k i n y x n x x Let’s start by seeding this with * * *0 1 2 0 . * * * * 0 0 1 1 2 2 * * * * 0 0 1 1 2 2 ... * ... 0.5 1 i i i p ip i i i p ip x x x x i x x x x e e and * * * * 0 0 1 1 2 2 * * * * 0 0 1 1 2 2 ... * * 2 ... 1 0.25 1 i i i p ip i i i p ip x x x x i i x x x x e e . We get the following three equations: 1) 0 1 27.85 5.25 14.5 11.75 2) 0 1 218.52 14.5 67 39.25 3) 0 1 214.57 11.75 39.25 44.25 Which solves for: 0 2.028178251 ; 1 0.083080292 ; 2 0.135597582 and these will be used as the new values of *0 , *1 , and *2 . This gives values of: *1 0.157904528 , *2 0.303697024 , * 3 0.271444487 , *4 0.169268208 , *5 0.185853861 and *6 0.130950503 , closer to the actual iy values. We continue on, getting the following three equations: 1) 0 1 21.32167719 3.151029861 9.869221273 8.163909574 2) 0 1 22.38657963 9.869221273 46.46156915 29.4190373 3) 0 1 21.96401923 8.163909574 29.4190373 34.43730029 Which solves for: 85 0 0.923613886 ; 1 0.092129313 ; 2 0.083221544 and the new values of the parameters are: *0 2.951792138 ; *1 0.175209605 ; *2 0.218819126 . After 7 steps, the process converges to: * 0 3.406518037 ; *1 0.239302529 ; *2 0.251763229 The MLE estimates of the means now looks like: i 1ix 2ix iy i Model 1 i Model 2 i Model 3 i Binomial 1 1 2 0.11 0.089028 0.077755 0.081524 0.065150 2 3 7 0.29 0.293421 0.294011 0.292561 0.283683 3 6 4 0.25 0.250789 0.255831 0.253173 0.276148 4 2 2 0.11 0.108077 0.099558 0.102294 0.081332 5 5 1 0.13 0.131964 0.130438 0.130705 0.123657 6 0 1 0.02 0.036721 0.021421 0.026855 0.040904 If one were to encounter a new set of inputs, 71 7x , 72 3x then the four models would produce different potential means: Normal Model 1: 7 0.236578947 ; Normal Model 2: 7 0.243104953 ; Normal Model 3: 7 0.240044134 ; Binomial Model: 7 0.273664197 ; The binomial model produces a result noticeably different than the others. We’ll illustrate the Poisson regression on some real data. The regression uses 1 0 n i ij i ij i i y x x a where lni , ( ) 1a and ii e . The canonical link function looks like: 0 0 1 1 2 2ln ...i i i i p ipx x x x . This creates the set of 1p equations: 1 1 i n n i ip ip i i ey x x They are non-linear, but can be solved using an iterative least squares process as for the binomial … Let *p p p where *p is an initial estimate and p is small. A Taylor expansion for ie can now be found which is linear in the p values: * * * *0 0 1 1 2 2 0 0 1 1 2 2... ...i i i i p ip i i i p ipx x x x x x x x 86 * * * *0 0 1 1 2 2 0 0 1 1 2 2... ...i i i p ip i i i p ipi x x x x x x x xe e e * * * *0 0 1 1 2 2 ... 0 0 1 1 2 21 ...i i i p ipx x x x i i i p ipe x x x x * * 0 0 1 1 2 2* ... i i i i i p ip i x x x x This leads to 1p interlocking linear equations for the values of k * * * * * * * *0 0 1 1 2 2 0 0 1 1 2 2... 0 . 1 .. 1 1 i i i p ip i i i p ip pn n x x x x xn i i ij i ij k i ik ij i i x k x i xn y x n x n xe e x Or * * * * * * * *0 0 1 1 2 2 0 0 1 1 2 2... 0 . 1 .. 1 1 i i i p ip i i i p ip pn n x x x x xn i i ij i ij k i ik ij i i x k x i xn y x n x n xe e x If we define * * * * 0 0 1 1 2 2 ...* i i i p ipx x x x i e this becomes: * 1 0 * 1 pn n i ij k ik ij i k i i i y x x x We will use annual data from hurricane seasons for the last 50 years. The table on the next page gives the number of named hurricanes in the Atlantic Ocean in every year from 1969 to 2018: 87 Year Hurricanes Year Hurricanes 1969 12 1994 3 1970 5 1995 11 1971 6 1996 9 1972 3 1997 3 1973 4 1998 10 1974 4 1999 8 1975 6 2000 8 1976 6 2001 9 1977 5 2002 4 1978 5 2003 7 1979 5 2004 9 1980 9 2005 15 1981 7 2006 5 1982 2 2007 6 1983 3 2008 8 1984 5 2009 3 1985 7 2010 12 1986 4 2011 7 1987 3 2012 10 1988 5 2013 2 1989 7 2014 6 1990 8 2015 4 1991 4 2016 7 1992 4 2017 10 1993 4 2018 8 We will assume hurricanes in a particular season follow a Poisson distribution and want to fit a regression equation: 2 0 1 2lni t t With 0t in 1969 and 49t in 2018. Let’s start by seeding this with *0 1.8468 87ln 7 68X and * *1 2 0 . * * *0 0 1 1 2 2* 6.34i i ix x xi e . We get the following three equations: 1) 0 1 20 317 7766.5 256294.5 2) 0 1 2530.5 7766.5 256294.5 9513962.5 88 3) 0 1 227552.5 256294.5 9513962.5 376701656.1 Which solves for: 0 0.141284954 ; 1 0.001086324 ; 2 0.000141831 and the new values of the parameters are: *0 1.705593815 ; *1 0.001086324 ; *2 0.000141831 . Iterating 5 times converges on: * 0 1.689124819 ; *1 0.002544836 ; *2 0.000109545 . These coefficients indicate that the number of hurricanes has been increasing and that the increase has been accelerating. A graph of Actual compared to Predicted Means is on the next page: 89 But are the coefficients “significant”? We need some kind of test for this… The “Log-Likelihood Test” will be used for this purpose. Suppose you have model p qM containing p q parameters and model pM containing p parameters. Twice the difference in the log-likelihoods of the models, 2 p q pM Ml l , is distributed as a chi-square distribution with q degrees of freedom. The log-likelihood for the exponential family is: 1 , n i i i i i i i y b c y a Let’s examine the normal distribution with constant variance first and see how far we can get. Recall: , 2 , ( )a , 2 ( ) 2 b , and 21( , ) ln 2 2 xc x . 1 , n i i i i i i i y b c y a 2 2 2 2 2 1 2 1 ln 2 2 i i in i i y y 2 2 2 2 1 2 1 ln 2 2 2 n i i i i i y y 2 2 2 1 ln 2 2 2 n i i i yn 90 We will compare this to something called the “saturated model”. This is a model where the number of parameters is equal to the number of data points n and the data is therefore reproduced exactly. Twice the difference in the log-likelihoods is: 2 2 1 2 2 1 n i in i i i i yy which is called the “scaled deviance”. This should be distributed as 2n p . The model could be viewed as “rejected” if the scaled deviance is too unlikely. Now recall our table of means for our original test sample: i 1ix 2ix iy i Model 1 i Model 3 i Binomial 1 1 2 0.11 0.089028 0.081524 0.065150 2 3 7 0.29 0.293421 0.292561 0.283683 3 6 4 0.25 0.250789 0.253173 0.276148 4 2 2 0.11 0.108077 0.102294 0.081332 5 5 1 0.13 0.131964 0.130705 0.123657 6 0 1 0.02 0.036721 0.026855 0.040904 Let’s test Model 1. If we assume 2 0.001 (that is, a variance of 1/ n for each line with 1000n ), our scaled deviance would be 0.739271255. This should be distributed as a chi-square with 3 degrees of freedom. The p-value is 0.863930348 which is well within the range where the model should not be rejected. For Model 3, twice the difference in the log-likelihoods can be shown to be 2 2 1 n i i i i y . This is the new definition of the scaled deviance. If we assume 2 2 1 100 100i i i , our scaled deviance would be 0.08402779. Again this should be distributed as a chi-square with 3 degrees of freedom. The p-value is 0.993682697 which is well within the range where the model should not be rejected. Recall for the binomial model: ln 1 p p , n , 1( )a , ( ) ln 1b e , and ( , ) lnc x x . 1 , n i i i i i i i y b c y a 91 l 1 1 n ln 1 ln 1 ln1 i i p i n pi i i i i i i y e n y p n p n 1 ln ln 1 ln 1 1 i i i n i i i i i ii i n n y np p n y p p 1 1 lln 1n ln1ln n n i i i i i i i i i i i i ip p p n n y n y n n y 1 1 1 lln 1n ln n n i i i i i i i i i i i n n y n y y p n p For the saturated model, i ip y so the log-likelihood is 1 1 ln 11 ln ln n n i i i i i i i i i i i n n yy y yn y n Taking the twice the difference gives the Scaled Deviance as: 1 1ln 1 2 1 lni i i i n i i i i i y yn y p n y p Our scaled deviance would be 16.22403782. Again this should be distributed as a chi-square with 3 degrees of freedom. The p-value is 0.001020137 and the model should be rejected at the 10%, 5% and 1% level. By the way, the form 1 1ln 1 2 1 lni i i i n i i i i i y yn y p n y p is not as different from 2 2 1 n i i i i y as it looks. If one assumes small deviations, i i iy p p we find 1 1ln 1 2 1 lni i i i n i i i i i y yn y p n y p 1 1l 1 1 2 lnn i i i ii i i i i n i i i in n p p p pp p p p p p 1 2 1 lnln 1 1 1 i i i i i i i i n i i i p pp p p p p n n p 2 2 1 1 1 2 1 1 2 1 2 i i i ii i i i n i i ii i i i p p p pp p p p p p p p n n 92 2 2 2 2 1 1 1 2 1 2 1 2 i i i ii i i i i n i i i i p p p p p p p p p n p n 2 1 1 1 1i i i n i i pn p p 2 1 1 i i i i n i p p p n 2 2 1 n i i i i y Where i ip and 2 1 i i i i p p n Using this approximation, our scaled deviance would be 15.6368526. Again this should be distributed as a chi-square with 4 degrees of freedom. The p-value is 0.001345901 and the model would be rejected at the 10%, 5% and 1% level. Let’s move on to an analysis of the hurricane model. Recall for the Poisson model ln , 1a , ( )b e and ( , ) ln !c x x . 1 , n i i i i i i i y b c y a 1 ln ln ! 1 n i i i i i y y 1 1 ln ln ! n n i i i i i i y y For the saturated model, i iy so the log-likelihood is 1 1 ln ln ! n n i i i i i i y y y y Taking the twice the difference gives the Scaled Deviance as: 1 1 2 ln 2 n n i i i i i ii yy y Our scaled deviance would be 58.10001197. This should be distributed as a chi-square with 46 degrees of freedom. The p-value is 0.108703215, implying we should not reject the model at the 10%, 5% or 1% level. 93 Again, this is approximately 2 2 1 n i i i i y which can be shown by the Taylor expansion: 1 1 2 ln 2 n n i i i i i ii yy y 1 1 2 ln 2 n n i i i i i i ii 1 1 2 ln 1 2 n n i i i i i ii 2 1 12 2 n i i i i i i i i 2 2 1 12 2 n i i i i i i i 2 1 n i i i 2 2 1 n i i i i y Where i i and 2i i Using this approximation, our scaled deviance would be 59.21051663. Again this should be distributed as a chi-square with 47 degrees of freedom. The p-value 0.091437109, implying we should reject the model at the 10% level, but not the 5% or 1% level. But is the 2t term really necessary? Well, the twice the difference between the loglikelihood of our original model and the model without the 2t term should be distributed as a chi-square distribution with one degree of freedom. Adding the 2t term should improve the scaled deviance by more than chance if it is relevant. For the reduced model, we find *0 1.64271258 ; *1 0.008057745 ; Our scaled deviance for the reduced model is 58.23205003. The scaled deviance for the original model was 58.10001197. The difference is 0.132038057. The probability that a difference this large or larger would arise by chance is 0.7163282. This implies the 2t is not necessary. What about the t term? For the “constant” model we find *0 1.846878768 . Our scaled deviance for this model is 62.500914. The difference from the t -only model is 4.268863972. The probability that a difference this large or larger would arise by chance is 0.038816873. This implies the t is probably necessary. 94 Chapter 8: Decision Theory There is a rich theory of games and decisions in economics. In this course, we’re primarily going to deal with relatively simple one-period simultaneous full-information two-person zero-sum games. The simplest representation is something like this: Player B 1b 2b Player A 1a 1,1L 1,2L 2a 2,1L 2,2L Player A can choose action 1a or 2a and, simultaneously Player B chooses action 1b or 2b . The players choose simultaneously and their strategies are unknown to the other player until revealed. You then look in the appropriate box and reward the players with “scores”. For instance, if Player A chooses 2a and Player B chooses 1b , Player A receives 2,1L and Player B receives 2,1L . We wish to determine the optimal strategies for Player A and B in the sense that they maximize the expected value of their score. A “Nash Equilibrium” exists if there is a saddle point. That is, if there is an element that is both the smallest in its row and largest in its column. If both players pick that strategy, neither has any incentive to deviate. They will lose “points” if they do. Sometimes, a payoff matrix may have multiple saddle points and multiple equilibria. An example of a matrix with a saddle point is: Player B 1b 2b 3b Player A 1a 9 1 0 2a 7 5 6 3a 4 3 2 Those numbers in boxes are the smallest in their rows. The numbers in red are the largest in their columns. The 5 in the middle is a saddle point. If Player B chooses 2b , Player A does best by choosing 2a and getting 5 points. If she deviates by choosing 1a or 3a , she gets only 3 or 1 point and has no reason to deviate. On the other hand, if Player A chooses 2a , Player B does best by choosing 2b and losing 5 points. If he deviates by choosing 1b or 3b , he loses 6 or 7 points and has no reason to deviate. There are no other Nash Equilibrium in this game, which can be seen by the fact that there are no other numbers in the table that are both boxed and red. 95 So what can we do if there are no saddle points? Well, first we look for dominated options. If one strategy is at least as good as another and sometimes better, that strategy can be discarded. In the matrix above, 1b is dominated. No matter what strategy Player A is using, Player B would prefer strategy 2b over strategy 1b since he loses fewer points in all cases. Similarly, strategy 3a is dominated. No matter what strategy Player B is using, Player A would prefer strategy 2a over strategy 3a since she gains more points in all cases. This leaves the reduced matrix: Player B 2b 3b Player A 1a 1 0 2a 5 6 We can see in this reduced matrix that strategy 2a now dominates 1a leading to: Player B 2b 3b Player A 2a 5 6 And finally, 2b dominates 3b leading to the same answer as the Nash Equilibrium. A slight change removes the possibility of eliminating dominated strategies: Player B 1b 2b 3b Player A 1a 9 1 0 2a 7 5 6 3a 2 3 7 There is now no dominant strategy for either player. However, 5 is still the only Nash equilibrium. What happens if there is no Nash Equilibrium after removing dominated strategies? Here’s an example of a payoff matrix with no dominated strategies and no saddle point: Player B 1b 2b 3b Player A 1a 9 1 0 2a 7 6 5 3a 2 3 7 96 One possible criterion to use is the “Mini-max” criterion. That is, you assume (unrealistically) that the other player will read your mind and do the worst thing to you. Then you pick the row that minimizes this bad outcome. For instance, in the above matrix, if Player A picks 1a , her worst payout is “0”. If she picks 2a , her worse payout is “5” and if she picks 3a , her worst payout is “2”. The mini-max criterion suggests playing 2a so she gets at least “5” if Player B reads her mind. Similarly, if Player B picks 1b his worst outcome is “-9”. If he picks 2b his worst outcome is “-6” and if he picks 3b his worst outcome is “-7”. The mini-max criterion suggests playing 2b in order to lose no more than “6” if player A reads his mind. This leads to the outcome 2a - 2b . Since this is not a Nash Equilibrium, this strategy is not robust. If Player B sees that Player A is always picking 2a , he changes to 3b in order to lose only “5”. Player A can stick with the mini-max strategy, but would do better to switch to 3a to win “7”. Then Player B changes to 1b to lose only “2”. And round and round we go. This leads to the idea of finding “mixed” Nash equilibria by using random strategies. A “mixed” strategy is one where the player picks randomly. That is, picking strategy 1 with probability 1p , strategy 2 with probability 2p and so on. Occasionally, you’ll find that a “pure” strategy is dominated by a mixed one, and that mixed one can be removed. Our current example is not of that type, but here’s an example that is like that: Player B 1b 2b 3b Player A 1a 5 21 0 2a 15 14 8 3a 10 14 22 There is clearly no saddle point. However, consider a strategy for Player B where he plays 1b with probability p and 3b with probability 1 p . If Player A chooses 1a , the payoff to B is 5 p . Player B prefers small scores and 5 p is always less than 21, so he prefers this mix to strategy 2b . If Player A chooses 2a , the payoff to B is 15 8(1 ) 8 7p p p . Since Player B prefers small scores, he prefers this mix to strategy 2b whenever 8 7 14p or 6 7 p . 97 If Player A chooses 3a , the payoff to B is 10 22(1 ) 22 12p p p . Since Player B prefers small scores, he prefers this mix to strategy 2b whenever 22 12 14p or 2 3 p . Therefore, this mixed strategy dominates strategy 2b for 2 6 3 7 p and strategy 2b can be eliminated, reducing the game to: Player B 1b 3b Player A 1a 5 0 2a 15 8 3a 10 22 Now, since Player prefers High Scores, she can remove strategy 1a as an option, and reduce the game to: Player B 1b 3b Player A 2a 15 8 3a 10 22 The game cannot be reduced further. What now? Let’s look at the game from Player B’s point of view. Suppose he commits in advance to playing 1b . Player A will always play 2a and Player B will score -15 every time. If he commits in advance to playing 3b , Player A will always play 3a and Player B will score -22 every time. But imagine Player B adopts a “mixed” strategy, playing 1b 90% of the time and 3b 10% of the time. If Player A chooses 2a , she will win 14.3 in expectation. If she chooses 3a , she will win 11.2 in expectation. She will therefore choose 2a . In this case, Player B will score -14.5 in expectation, so this strategy is better than 100% 1b ! Similarly, imagine Player B chooses 1b 10% of the time and 3b 90% of the time. If Player A chooses 2a , she will win 8.7 in expectation. If she chooses 3a , she will win 20.8 in expectation. She will therefore choose 3a . In this case, Player B will score -20.8 in expectation, so this strategy is better than 100% 3b ! 98 As Player B slowly lowers the probability of playing 1b from 100%, Player A continues to choose 2a and wins 10 5p . As player B slowly raises the probability of playing 1b from 0%, Player A continues to choose 3a and wins 22 12 p . If 8 7 22 12p p , Player A is completely indifferent between choosing 2a and 3a and wins 8 7 22 12p p no matter what she does. This occurs at 14 0.736842105 19 p and Player A wins 13.15789474 in expectation no matter what she does. Similarly, Player B scores -13.15789474 no matter what Player A does. The graph below shows the outcome to Player B assuming Player A plays optimally: The best result for Player B occurs where *Player A* is indifferent between her options. Therefore, Player B should 1b 14/19 of the time and 3b 5/19 of the time. Similarly, the best result for Player A occurs where *Player B is indifferent between his options. If you assume Player A plays 2a with probability p and 3a with probability 1 p . This occurs when -24 -22 -20 -18 -16 -14 -12 -10 0 0.1 0.2 0.3 0.4 0.5 0.6 0.7 0.8 0.9 1 Player B Outcomes 99 10 5 22 14p p or 12 0.631578947 19 p . She wins 13.15789474 regardless of Player B’s choices. Put “Augmented Rock Paper Scissors” example here. This brings up some interesting issues in “statistical” games or games against “Nature”. In the current game, Player B is an actual opponent, trying to make the best score for himself. Player A should react accordingly. Suppose, however, that player B is “nature” and picks state 1b with probability 25% and 3b with probability 75%. Looking at the graph above, Player A should *always* pick 3a , which gives an expected score of 19. Her “mixed” strategy above would only give 13.15789474 and the strategy of always picking 2a is terrible, being worth 9.75 in expectation. In other words, one should play differently against disinterested nature than an optimizing opponent. Two examples. Suppose someone has 100 cards, with a 70% of being blue and 30% of being red. You call out “blue” or “red” before each card is turned over and get $1 for every right answer. Many people randomize, saying “blue” about 70% of the time and “red” about 30% of the time. This gives an expected payout of $100 0.7 0.7 0.3 0.3 $58 . Calling out “blue” every time has an expected payout of $70 . It usually doesn’t pay to randomize against a disinterested opponent. If this seems counterintuitive, imagine the situation where you are told there is, say, a 70% of rain. You don’t carry an umbrella 70% of the time and not carry it 30% of the time. Instead, you determine a threshold percentage, above which you should *always* carry an umbrella and below which you should *never* carry an umbrella.