xuebaunion@vip.163.com
3551 Trousdale Rkwy, University Park, Los Angeles, CA
留学生论文指导和课程辅导
无忧GPA:https://www.essaygpa.com
工作时间:全年无休-早上8点到凌晨3点
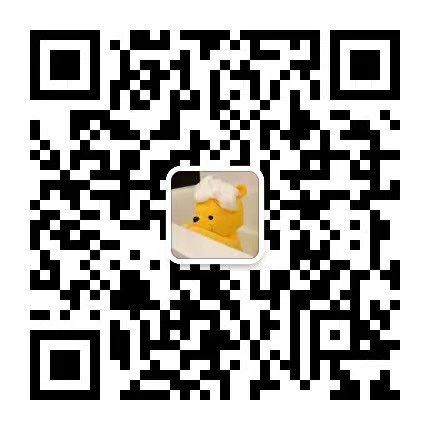
微信客服:xiaoxionga100
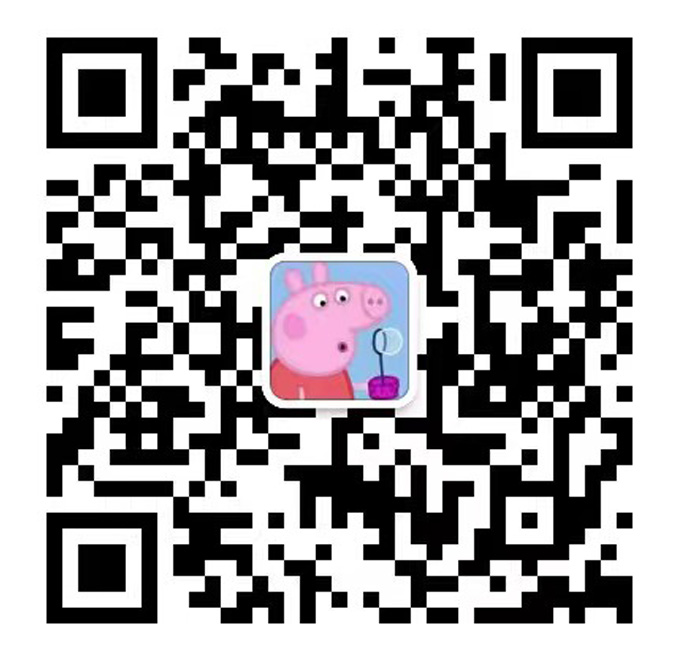
微信客服:ITCS521
1 Data Analytics Project for AVIA2601 You are given two datasets which contain flight delay records between January-March 2018 and July-September 2018 from the Head of Data Analytics of FAA to conduct analysis. The summer in 2018 was a busy time for the US airline industry and hence, many flights were delayed due to different reasons. This was similar to the winter season in early 2018. For reporting purposes, FAA asked US carriers to report delay causes by the following five groups: Delay Cause Group (variable name*) Notes CarrierDelay Carrier Delay, in Minutes WeatherDelay Weather Delay, in Minutes NASDelay National Air System Delay, in Minutes SecurityDelay Security Delay, in Minutes LateAircraftDelay Late Aircraft Delay, in Minutes (*For the full data dictionary, please check the readme.html file that comes with the data you downloaded.) You, as a data analyst in FAA, is to harvest as much insights as possible from the available data and advise the Head of Data Analytics, Dr. Wu on how to improve flight scheduling policies and operations of the airline industry in the US. Hence, your insights are critical in this project in shaping up the new schedule and future operations policy for the US airspace. It is noted that passenger satisfaction is a top priority for FAA and flight OTP (on-time performance) is one of the key factors that affect passenger satisfaction. So, any insights on flight delays, flight scheduling, and aircraft ground operations at airports are essential for future policies, schedule improvement, operational improvement, airport capacity planning, and passenger satisfaction. It is also suspected that seasonality played a role in flight on- time performance. Hence, you are given the winter (Jan-Mar 2018) and the summer (Jul-Sep 2018) season data for cross analysis between seasons. 2 Data source & data dictionary: You can download the data directly from TranStats of the Bureau of Transportation Statistics (https://transtats.bts.gov/Homepage.asp). Follow the following procedure: 1. Click the Aviation mode (under Data Finder on the left menu); 2. Choose ‘Airline On-time Performance Data’ from the list; 3. Choose ‘Reporting Carrier On-Time Performance (1987-present)’ link; 4. On the left menu under Data Tools, choose ‘Download’; 5. Choose All categories, choose year-2018 and choose month. Also click ‘Prezipped file’ and ‘%missing’, then click the download button to download 2018 OTP data. You can only download data by month, so you need to change the ‘month’ in the menu and download for Jan-Mar and Jul-Sep 2018. You shall have 6 files in total. Check the screen shot below for settings. The July file I downloaded was about 291.7MB and came with a ‘readme.html’ data dictionary. The ‘readme’ file can be opened in any web browser and it’s your ‘data dictionary’. Please read your data and the data dictionary carefully before embarking on your data project. It’s more complicated than you think 3 Part #1- Milestone A: Data Exploration and Visualisation Milestone Part #1-A There are three parts in your data project and there are two milestones for your Part #1. Your job for Milestone #A (Part #1-A) is to explore this dataset and provide meaningful insights to Dr. Wu. You are free to explore the data with Python (but NO Excel and NO PySpark SQL!). The following tasks must be conducted: a) Descriptive statistics for the On-Time Performance (OTP) of all reporting airlines: you can group by airports, departure or arrival, delays, aircraft tail number, delay causes, taxi in and out delays … etc. Use your creativity and aviation knowledge to explore and organise your insights. b) Comparison among airlines in the same dataset by meaningful ways such as the same departure airport, or the same period of departure/arrival time. o What can you observe from the data? o Did seasonality play a role in OTP in 2018? o Were some airports affected more by weather than others? c) What factors contributed to delays in 2018 in the US and how airlines were affected? For example, how did taxi delays contribute to overall flight delays including taxi- out/taxi-in delays? You can group the insights by ports, by time slots, or by airlines; up to your creativity. o Can you advise Dr. Wu on delay improvement directions, airport capacity bottlenecks, and passenger satisfaction outcomes? o How do these insights affect future ops and policy making in the US? 4 Assessment criteria Compulsory tasks listed above for each milestone must be done. Finishing this will give you a Pass mark. To gain higher marks, then you will need to explore the data further and make meaningful analysis or modelling based on the available data. Cross-season comparison would be insightful and is encouraged. Dr. Wu is looking for meaningful discussions on your results/models, so pay attention to result discussions and insight analysis. Simple reporting of statistics will give you a so-so PS mark, so be aware (and be kindly warned). Beautiful visuals will help but beautiful charts alone won’t get you too further up from the PS mass; use visuals as tools to help you tell your stories. Hence, focus on the stories that you can tell. “Go further and trouble yourself in this project because that’s where gold (mark) is!”- Dr. Wu 5 Submission guide All submissions must be done on Moodle; please check Moodle for exact deadlines. Please also follow the submission guide: 1. Codes: You are required to submit the original Jupyter Notebook file and other associated files including output files such as graphs. Do NOT submit the original data file. The Jupyter Notebook file is to verify your codes by the assessor so make sure you provide sufficient ‘comments’ in your Notebook. If your codes don’t run and cannot generate the same results as shown in your report, then you will receive a FL mark. 2. Summary report: Data insights and modelling discussions should be provided in a summary report (not in the working Jupyter file) for ease of reading and report writing. Size of the report doesn’t matter but quality discussions and insights do because they will give you higher marks! Simply reporting results will give you a PS mark only. The soft copy of your report MUST be in PDF format and contained in ONE single PDF file only for submission (20% off penalty, if you don’t follow this document preparation rule). 3. File naming convention for submission: a. Name your report file in the following format: zID_report_PartX- Milestone_Y.pdf; b. Name your Jupyter working file in the following format: zID_codes_PartX- Milestone_Y.ipynb. 6 Submission check list for you: Create a new folder and name it by ‘ZID_PartX-MilestoneY’ Create a sub-folder and name it by ‘Codes’. Copy all the contents of your working Jupyter Notebook folder over, except the OTP data file. Create another sub-folder and name it by ‘Reports’. Copy your ZID_reportMilestoneX.pdf report file over. Zip the ‘ZID_ PartX-MilestoneY’ folder. o For Mac users, you can right click and choose “Compress ZID_ PartX- MilestoneY” to create a zip ball for submission. o For Win users, you may try WinZip or 7-Zip or other similar tools. When all the above are ticked, then submit the ZID_PartX-MilestoneY.zip on Moodle before deadline. Update your LinkedIn profile by adding Machine Learning and AI Analytics to your profile. Sit back, admire your report, enjoy your achievement, and relax (with whatever you’d like)! (Warning: binge drinking after report submission is not encouraged!) Penalties to late submissions are heavy; 10% reduction per day, so be on time! Don’t submit at the last minute because it’s usually a lot ‘bumpier’ at the last minute before a deadline (and everything could go wrong)! Have fun and enjoy! ^_< Dr. C. Wu CAO, FAA