xuebaunion@vip.163.com
3551 Trousdale Rkwy, University Park, Los Angeles, CA
留学生论文指导和课程辅导
无忧GPA:https://www.essaygpa.com
工作时间:全年无休-早上8点到凌晨3点
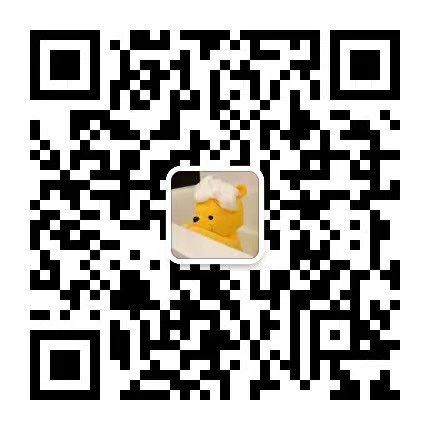
微信客服:xiaoxionga100
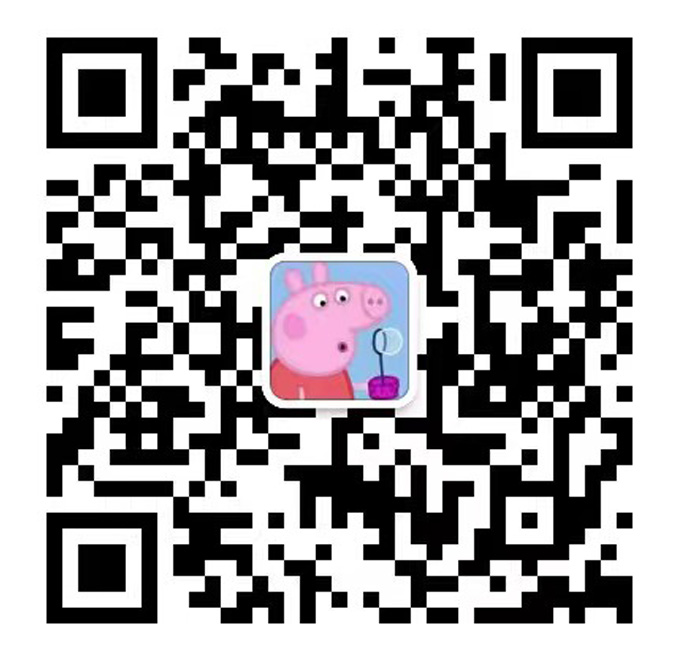
微信客服:ITCS521
STAT3500/7500 Assignment 3 (a) [5 marks] Let x = (wT1 , . . . , w T n ) T contain the observed values of a random sample W 1, . . . , W n i.i.d.∼ Fθ, (1) where Fθ denotes a distribution function indexed by the parameter vector θ = (θ1, . . . , θd) T . Suppose that the likelihood function L(θ) for θ belongs to the regular exponential family so that it is of the form L(θ) = b(x) exp{c(θ)T t(x)}/a(θ), (2) where c(θ) = (c1(θ), . . . , cd(θ)) T is the canonical parameter vector and its coefficient, t(x) = (t1(x), . . . , td(x)) T , is a (complete) sufficient statistic for θ. (i) [2 marks] Show that the score statistic (the gradient of the log likelihood for θ) can be expressed as ∂ logL(θ)/∂θ = (∇c(θ)T ) [t(x)− E{t(X)}], (3) where X denotes the random vector corresponding to x and ∇ = (∂/∂θ1, . . . , ∂/∂θd)T . (ii) [3 marks] The ML estimate θˆ of θ is a solution of the equation E{t(X)} = t(x), (4) that is, Eθˆ{t(X)} = t(x), (5) where Eθˆ denotes expectation using θˆ for θ. Establish this result in the case where the parameter θ is a scalar and is the canonical parameter; that is, c(θ) = θ. (b) [3 marks] Let x denote the complete-data vector in a EM framework adopted to calculate the ML estimate of a parameter θ on the basis of some observed data y whose distribution is indexed by θ. If the complete-data likelihood Lc(θ) belongs to the regular exponential family, then on the M-step of the (k+ 1)th iteration the updated estimate θ(k+1) of θ can be calculated as the solution of the equation E{t(X)} = t(k)(y), (6) where t(k)(y) = Eθ(k){t(X) | y}; (7) that is, θ(k+1) satisfies the equation Eθ(k+1){t(X)} = t(k)(y). (8) Establish this result in the case where c(θ) = θ. (c) [4 marks] Suppose that a random sample of size n is taken from a two-component mixture density, f(w; Ψ) = 2∑ i=1 pii fi(w;θi), (9) where Ψ = (pi1,θ T 1 ,θ T 2 ) T . (i) [2 marks] Show that the likelihood equation for Ψ can be manipulated into the form pˆi1 = n∑ j=1 τ1(wj; Ψˆ)/n, (10) θˆi = n∑ j=1 τi(wj; Ψˆ) ∂ log fi(wj; θˆi)/∂θi (i = 1, 2), (11) where τi(wj; Ψˆ) = pˆii fi(wj; θˆi) f(wj; Ψˆ) (i = 1, 2; j = 1, . . . , n). (ii) [2 marks] Suggest a method for solving these equations iteratively to find the ML estimate Ψˆ of Ψ. (d) [6 marks] Let W 1, . . . , W n denote a random sample on the random variable W , which has a g-component normal mixture density, f(w; Ψ) = g∑ i=1 pii φ(w;µi,Σi), where φ(w;µ,Σ) denotes the p-dimensional multivariate normal density with mean µ and covariance matrix Σ. Here Ψ = (pi1, . . . , pig−1,θ T 1 , . . . , θ T g ) T , where θi contains the p elements of µi and the 1 2 p(p+ 1) distinct elements of the covariance matrix Σi for i = 1, . . . , g. It is proposed to apply the EM algorithm to find the ML estimate of the parameter vector Ψ as a solution of the likelihood equation, ∂ logL(Ψ)/∂Ψ = 0, where logL(Ψ) = n∑ j=1 log g∑ i=1 pii φ(wj;µi,Σi). In the EM framework adopted here, wj is viewed as a realization of the random variableW j drawn at random from a mixture of g Classes C1 . . . , Cg in proportions pi1, . . . , pig in which W has density φ(w;µi,Σi) in Class Ci (i = 1, . . . , g). Accordingly, we introduce z = (zT1 , . . . , z T n ) T as the missing-data vector, where zij = (zj)i is defined by zij = 1, if wj ∈ Ci, = 0, otherwise, for i = 1, . . . , g; j = 1, . . . , n. Hence the complete-data vector x is given by x = (yT , zT )T , where y = (wT1 , . . . , w T n ) T denotes the (observed) incomplete-data vector. (i) [2 marks] Give the complete-data likelihood Lc(Ψ) for Ψ. (ii) [2 marks] Calculate the Q-function, Q(Ψ; Ψ(k)), on the E-step of the (k + 1)th EM iteration. (iii) [2 marks] The form of the maximum likelihood (ML) estimate of Ψ obtained di- rectly by differentiation of the complete-data log likelihood logLc(Ψ) is known and it applies also when the known zero-one values of z1, . . . , zn are replaced by fractional values between zero and one. Use this result to derive the updated estimate Ψ(k+1) of Ψ on the M- step of the (k + 1)th EM iteration. (e) [12 marks] Another approach to the problem in (d) for calculating the ML estimate of Ψ on the basis of the observed data y is to use the fact that the complete- data density gc(x; Ψ) belongs to the regular exponential family. It can be shown that for gc(x; Ψ)) in the regular exponential form (2), the sufficient statistic statistic t(X) contains in the present context of a g-component normal mixture model the (g − 1) elements, n∑ j=1 Zij (i = 1, . . . , g − 1), the p elements in each of the g vectors, n∑ j=1 ZijW j (i = 1, . . . , g), and the 1 2 p(p+ 1) distinct elements in each of the g symmetric matrices, n∑ j=1 ZijW jW T j (i = 1, . . . , g). (i) [4 marks] Calculate E(t(X)} and E{t(X) | y} from the above expressions for the elements of t(X). Use the relationship (8) to derive the updated estimate Ψ(k+1) of Ψ on the (k + 1)th EM iteration. (ii) [4 marks] In the univariate case (p = 1) of the two-component normal mixture density, f(w; Ψ) = 2∑ i=1 pii φ(w;µi, σ 2 i ), where now Ψ = (pi1, µ1, µ2, σ 2 1, σ 2 2) T , verify that the complete-data density gc(x; Ψ) belongs to the regular exponential family. Give explicitly the values of the canonical parameter c(Ψ), the suffi- cient statistic t(x), a(Ψ), and b(x). (iii) [4 marks] Verify the result that E{t(X)} = ∂ log a(Ψ)/∂Ψ, using the expressions obtained above in e(ii).