xuebaunion@vip.163.com
3551 Trousdale Rkwy, University Park, Los Angeles, CA
留学生论文指导和课程辅导
无忧GPA:https://www.essaygpa.com
工作时间:全年无休-早上8点到凌晨3点
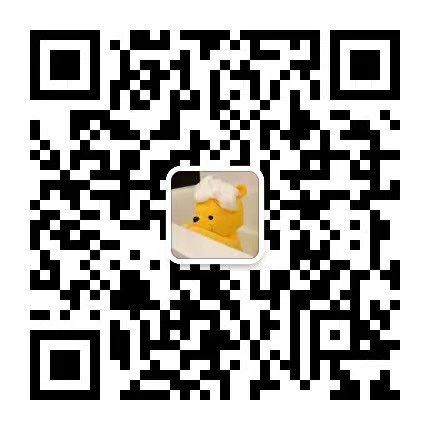
微信客服:xiaoxionga100
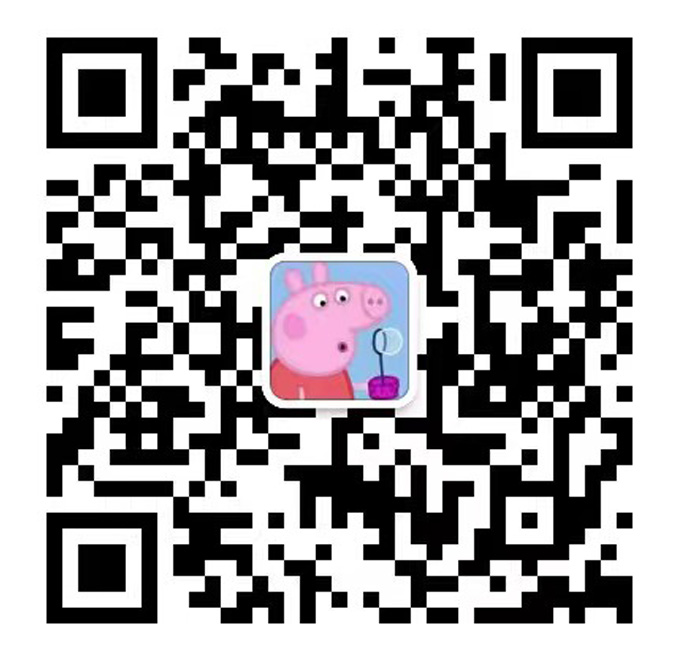
微信客服:ITCS521
IFT 6758 Project: Milestone 1 Released: Sep. 17, 2021 Due date: Oct. 15, 2021 The goal of this milestone is to give you experience with the data wrangling and exploratory data analysis phases of a data science project, which are often where you will spend most of your time during a data science project. You will gain experience with some of the common tools used to retrieve and manipulate data, as well as build confidence in creating tools and visualizations to help you understand the data prior to jumping into more advanced modeling. Broadly, the outline for this milestone is to use NHL stats API to retrieve both aggregate data (player stats for a given season) and “play-by-play” data for a specific time period and to generate plots. You will begin with creating simple visualizations from the aggregate data that do not require much preprocessing, and then move to creating interactive visualizations from the play-by-play data which will involve more work to prepare. There will be a small number of simple qualitative questions to answer throughout the tasks that will relate to the tasks outlined. Finally, you will present your work in the form of a simple static web page, created using Jekyll. Note that the work you do in this milestone will be useful for future milestones, so make sure your code is clean and reusable - your future self will thank you for it! A note on Plagiarism 2 NHL data 3 Motivation 4 Learning Objectives 5 Deliverables 5 Submission Details 6 Tasks and Questions 6 1. Warm-up (10%) 7 2. Data Acquisition (25%) 7 3. Interactive Debugging Tool (bonus 5%) 9 4. Tidy Data (10%) 11 5. Simple Visualizations (25%) 11 6. Advanced Visualizations: Shot Maps (30%) 12 7. Blog Post (up to 30% penalty) 14 Group Evaluations 14 Useful References 15 1 Due: IFT 6758 2021 - Milestone 1Oct. 15, 2021 A note on Plagiarism Using code/templates from online resources is acceptable and it is common in data science, but be clear to cite exactly where you took code from when necessary. A simple one line snippet which covers some simple syntax from a StackOverflow post or package documentation probably doesn’t warrant citation, but copying a function which does a bunch of logic that creates your figure does. We trust that you can use your best judgement in these cases, but if you have any doubts you can always just cite something to be safe. We will run some plagiarism detection on your code and deliverables, and it is better to be safe than sorry by citing your references. Integrity is an important expectation of this course project and any suspected cases will be pursued in accordance with Université de Montréal’s very strict policy. The full text of the university-wide regulations can be found here. It is the responsibility of the team to ensure that this is followed rigorously and action can be taken on individuals or the entire team depending on the case. 2 Due: IFT 6758 2021 - Milestone 1Oct. 15, 2021 NHL data The subject matter for this project is hockey data, specifically the NHL stats API. This data is very rich; it contains information from many years into the past ranging from metadata about the season itself (eg. how many games were played), to season standings, to player stats per season, to fine-grained event data for every game played, known play-by-play data. If you’re unfamiliar with play-by-play data, the NHL uses this exact data to generate their play-by-play visualizations, an example of which is shown below. For a single game, roughly 200-300 events are tracked, typically limited in scope to faceoffs, shots, goals, saves, and hits (no passes or individual player location). Note that there is a logical way the games are assigned a unique ID, which is described here (take care to note the difference between regular season and playoff games!). Figure 1: Sample play-by-play data for game 2017020001 at the beginning of the 1st period. Each event contains an identifier (e.g. “FACEOFF”, “SHOT”, etc.), a description of the event, the players involved, as well as the location of this event on the ice (drawn on the ice rink to the far right). The raw event data contains more information than this. You can explore this game’s play-by-play here. The time of event, event type, location, players involved, and other information is recorded for each event and the raw data is accessible through the play-by-play API. For example, the raw data for the above play-by-play can be found here: https://statsapi.web.nhl.com/api/v1/game/2017020001/feed/live/ A snippet of the raw event data can be seen in Figure 2. You will need to explore the data and read the API docs to figure out exactly what you will need. 3 Due: IFT 6758 2021 - Milestone 1Oct. 15, 2021 Figure 2: Raw JSON data obtained from the NHL stats API for the same events in Figure 1. Note that there are other events between the desired ones - that will be up for you to explore! Although technically undocumented, there is a very detailed unofficial API document maintained by the community, which should be the first place you look for information about the API. Motivation While we understand some people may not be sports fans, we think this is an exciting dataset to work with for a number reasons: 1. It is a real-world dataset that is used by professional data scientists, some of which are employed by the NHL teams themselves, and others run their own analytics businesses. 2. During the hockey season, data is updated live as games are in progress! This gives the opportunity to interact with new data frequently, giving you some insight as to why “pipelining”, and writing clean and reusable code is critical in a successful data science workflow. 4 Due: IFT 6758 2021 - Milestone 1Oct. 15, 2021 3. It is very rich, as discussed above. 4. It is “clean” in the sense that the API is consistent and you will not have to deal with parsing or cleaning nonsensical data. 5. It is “messy” in the sense that all of the raw data is in JSON, and not immediately suitable for use in a data science workflow. You will need to “tidy” the data into a usable format, which is a significant portion of many data science projects. Because the data already comes in a consistent format, we think this is a good balance between giving you some work to do to clean the data, while not being unreasonable. 6. Hockey is often a great conversation facilitator here in Canada (and especially Montreal). If you’re new to Canada, this is a great way to learn a little bit about our culture :) Even if you are not a hockey fan, we hope that you will find this project experience interesting and educational. We think that playing with real-world data is more rewarding and far more representative of the data science workflow than working with prepared datasets such as those available on Kaggle. If you are particularly proud of your project, some of the deliverables will teach you how to host your content in a publicly accessible way (via Github pages), which can help you in your future internship or job hunts! Learning Objectives ● Data acquisition and cleaning ○ Understand what a REST API is ○ Programmatically downloading data from the internet using Python ○ Format the raw data into useful tables of data ○ Get familiar with the idea of “pipelining” your work; i.e. creating logically separated components such as: ■ Download and save data ■ Load raw data ■ Process raw data into some format ● Data exploration ○ Explore the raw data and understand what it looks like ○ Build simple interactive tools to help you work with the data more efficiently ● Visualization and Exploratory Data Science ○ Gain some intuition and answer some simple questions about the data by looking at visualizations ○ Use Matplotlib and Seaborn to create nice figures ○ Create interactive figures to communicate your results more effectively Deliverables You must submit BOTH: 1. Blog post style report 2. Your team’s codebase that is reproducible 5 Due: IFT 6758 2021 - Milestone 1Oct. 15, 2021 Instead of a traditional report written in LaTeX, you will be asked to submit a blog post which will contain discussion points and (interactive!) figures. We will provide a template and instructions by , so don’t worry about having to figure it all out by yourself. At a high level,Sep. 22, 2021 you will use Jekyll to create a static web page from Markdown. This is a very simple way to create nice looking pages, and could be very useful for you to create blog posts in the future if you are interested, or wish to buff up your resume in a job hunt. Although we will not be deploying these pages to the public1, it is very simple to use github pages to publish your content. You’re more than welcome to do this at the end of the course! Submission Details To submit your project, you must: Publish your final milestone submission to the master or main branch (You must do this first before downloading the ZIPs!) Submit a ZIP of your blog post to gradescope Submit a ZIP of your codebase to gradescope Add the IFT6758 TA Github account (@ift-6758) to your git repo as a viewer To submit a ZIP of your repository, you can download it via the Github UI: Remember that this method does not download the whole git repo, just the master or main branch. Make sure all of your code is committed to the master branch before downloading the ZIPs. Tasks and Questions The tasks required for milestone 1 are outlined here. The overall description of what is required is described at the beginning of each task. The Questions section of each task will outline 1 A caveat about Github pages is that even if your repo is private, any published pages are public. You may not even be able to publish a page from a private repo if you didn’t get your free student Github Pro account. Because we don’t want groups to have their pages visible to one another while they are working on the project, you should not publish the pages you create and instead render them locally. 6 Due: IFT 6758 2021 - Milestone 1Oct. 15, 2021 content that is required in the blog post. This may either be interpretation questions, or you may have to produce figures or images to include in the blog post. We try to write most of the things that are required in the report in bold, but make sure you answer everything asked of you in each question. We do not expect long responses for the questions, in most cases a few sentences will suffice. 1. Warm-up (10%) Let's start with some very simple plots for visualizing player statistics to get your feet wet. This part will be a bit different than the next sections due to it being rather tedious to get player stats from the NHL API. While it's certainly possible, it's much easier to just scrape a webpage that already tabulates the exact data that we want. Because this is a bit disconnected from the next tasks, we provide a function to scrape the data and format it into a DataFrame for you to work with. You will still need to be mindful of NaNs however! Questions We’ll try to explore goaltenders and consider who was the best goaltender of the 2017-2018 season. Use the provided function to download the goalie stats for the 2017-2018 season. 1. Sort the goalies by their save percentage (‘SV%’), which is the ratio of their shots saved over the total number of shots they faced. What issues do you notice by using this metric to rank goalies? What could be done to deal with this? Add this discussion to your blog post (no need for the dataframe or a plot yet). Note: You don’t need to create a fancy new metric here. If you’d like, you can do a sanity check against the official NHL stats webpage. You also don’t need to reproduce any particular ranking on the NHL page; if your approach is reasonable you will get full marks. 2. Filter out the goalies using your proposed approach above, and produce a bar plot with player names on the y-axis and save percentage (‘SV%’) on the x-axis. You can keep the top 20 goalies. Include this figure in your blog post; ensure all of the axes are labeled and the title is appropriate. 3. Save percentage is obviously not a very comprehensive feature. Discuss what other features could potentially be useful in determining a goalie’s performance. You do not need to implement anything unless you really want to, all that’s required is a short paragraph of discussion. 2. Data Acquisition (25%) Create a function or class to download NHL play-by-play data for both the regular season and playoffs. The primary endpoint of interest is: https://statsapi.web.nhl.com/api/v1/game/[GAME_ID]/feed/live/ 7 Due: IFT 6758 2021 - Milestone 1Oct. 15, 2021 You will need to read the unofficial API doc to understand how GAME_ID is formed. You could open up the endpoint in your browser to check out the raw JSON to explore it a little bit (Firefox has a nice built-in JSON viewer). Use your tool to download data from the 2016-17 season all the way up to the 2020-21 season. You can implement this however you wish, but if you need guidance here are some tips: 1. This is a public API, and as such you must be mindful that someone else is paying for the requests. You should download the raw data and save it locally, and then use this local copy to derive tidy/usable datasets from it. 2. Do not commit the data (or large binary blobs) to your GitHub repo. This is bad practice, git is for code, not file storage. While it may be possible for the dataset you will be working with, it likely won’t be when you work on larger-scale projects in industry or academia. Larger git repos become slow to clone and work with. Note that because of the way git works, once you commit and push a file, simply removing it and committing the deletion won’t actually delete the file; you’ll need to actually rewrite the git history which becomes risky. A good way to accidentally avoid committing files is to use a .gitignore file, and add whatever file pattern you may want (such as *.npy or *.pkl). 3. A nice pattern could be to define a function that accepts the target year and a filepath as an argument and then checks at the specified filepath for a file corresponding to the dataset you are going to download. If it exists, it could immediately open up the file and return the saved contents. If not, it could download the contents from the REST API and save it to the file before returning the data. This means that the first time you run this function, it will automatically download and cache the data locally, and the next time you run the same function, it will instead load the local data. Consider using environment variables to allow each teammate to specify different locations, and having your function automatically retrieve the location specified by the environment variable so you don’t have to fight about paths in your git repository. 4. If you wanted to get even fancier, you could consider pushing this logic into a class which implements the logic suggested in (3). This lends itself nicely to how the data is separated by hockey seasons, and it would allow you to add logic that would generalize to any other season that you may wish to analyze in a clean and scalable way. To get even fancier still, you could consider overloading the “add” (__add__) operator on this class to allow you to add the data between seasons to a common data structure, allowing you to aggregate data across seasons. This is absolutely not required, these are just some ideas to inspire you! You are encouraged to be creative and apply your old data structures/OOP knowledge to data science - it can make your life a lot easier! 5. Writing docstrings for your functions is a good habit to get into. 8 Due: IFT 6758 2021 - Milestone 1Oct. 15, 2021 Questions 1. Write a brief tutorial on how your team downloaded the dataset. Imagine :) that you were searching for a guide on how to download the play-by-play data; your guide should make you go “Perfect - this is exactly what I was looking for!”. This can be as simple as copying in your function and an example usage, and writing one or two sentences describing it. 3. Interactive Debugging Tool (bonus 5%) When working with new data, it’s often useful to create simple interactive tools to help you go through the data and prototype implementations. One useful tool is ipywidgets, which allows you to very quickly and easily create HTML widgets within a Jupyter notebook cell. A common use case for these widgets is to apply them as decorators, and use them to specify function arguments. For example, if you want to retrieve information that resides in an element of an array, you can use an IntSlider to control the index which is passed into this function. You then can define logic in this function to display your image; if your list is a list of image paths, you can load up the image and show it via matplotlib. These widgets can also be nested, allowing you a high degree of flexibility with very little effort. For full bonus points, implement an ipywidget that allows you to flip through all of the events, for every game of a given season, with the ability to switch between the regular season and playoffs. You may print whatever information you find useful, such as game metadata/boxscores, and event summaries, or even drawing the event coordinates on an ice rink figure where applicable. This is meant to help create a tool that is useful to you, so you will not be graded on the outputs you produce. Questions 1. Take a screenshot of the tool and add it to the blog post, accompanied with a brief (1-2 sentences) description of what your tool does. You do not need to worry about embedding the tool into the blogpost. Note: A nice sanity check for this is to cross reference a specific game to the data available on the NHL website, an example of which can be found here. You’ll notice the coordinates of the event are also drawn, which allows you to confirm if your figures are valid. You’re going to have to figure out how to convert from the coordinates specified in the data to drawing them on a figure anyway, so it’s recommended that you do this before moving on to the aggregate shots because you might make a mistake! For inspiration, a screenshot of the one I quickly created can be found below. You do not need to copy this layout; feel free to include whatever information you find useful! 9 Due: IFT 6758 2021 - Milestone 1Oct. 15, 2021 An example of an interactive widget that you can create to explore the data. This was created using simple ipywidgets and stock matplotlib. 10 Due: IFT 6758 2021 - Milestone 1Oct. 15, 2021 4. Tidy Data (10%) Now that you’ve obtained and explored the data a bit, we need to format the data in a way that will make it easier to do data science (i.e. tidy the data)! We generally want to work with nice Pandas dataframes rather than raw data, so here you are tasked with processing the raw event data from every game into dataframes that will be usable for the subsequent tasks. You may find this endpoint useful: https://statsapi.web.nhl.com/api/v1/playTypes Create a function to convert all events of every game into a pandas dataframe. For this milestone, you will want to include events of the type “shots” and “goals”. You can ignore missed shots or blocked shots for now. For each event, you will want to include as features (at minimum): game time/period information, game ID, team information (which team took the shot), indicator if its a shot or a goal, the on-ice coordinates, the shooter and goalie name (don’t worry about assists for now), shot type, if it was on an empty net, and whether or not a goal was at even strength, shorthanded, or on the power play. Questions 1. In your blog post, include a small snippet of your final dataframe (e.g. using head(10)). You can just include a screenshot rather than fighting to get the tables neatly formatted in HTML/markdown. 2. You’ll notice that the “strength” field (i.e. even, power play, short handed) only exists for goals, not shots. Furthermore, it doesn’t include the actual strength of players on the ice (i.e. 5 on 4, or 5 on 3, etc). Discuss how you could add the actual strength information (i.e. 5 on 4, etc.) to both shots and goals, given the other event types (beyond just shots and goals) and features available. You don’t need to implement this for this milestone. 3. In a few sentences, discuss some additional features you could consider creating from the data available in this dataset. We’re not looking for any particular answers, but if you need some inspiration, could a shot or goal be classified as a rebound, or a shot off the rush? 5. Simple Visualizations (25%) Lets now use the tidied data to create some simple distributions over the aggregate data. Questions 1. Produce a histogram OR BARPLOT of shot types over all teams in a season of your choosing. Overlay the number of goals overtop the number of shots. What appears to be the most dangerous type of shot? The most common type of shot? Add this figure and discussion to your blog post. 11 Due: IFT 6758 2021 - Milestone 1Oct. 15, 2021 2. What is the relationship between the distance a shot was taken and the chance it was a goal? Produce a figure for each season between 2018-19 to 2020-21 to answer this, and add it to your blog post along with a couple of sentences describing your figure. Has there been much change over the past three seasons? Note: there are multiple ways to show this relationship! If your figure tells the correct story, you will get full marks. 3. Combine the information from the previous sections to produce a figure that shows the goal percentage (# goals / # shots) as a function of both distance from the net, and the category of shot types (you can pick a single season of your choice). Briefly discuss your findings; e.g. what might be the most dangerous types of shots? 6. Advanced Visualizations: Shot Maps (30%) The final set of visualizations that you will create are shot maps for a given NHL team, for a given year and season. This will be much easier If you’ve completed the bonus task of creating an interactive debugging tool and drew the event coordinates on the ice rink. A great example of these plots, with a detailed description of how to read them, can be found on the hockeyviz website (which is a great resource for many things about hockey data science). Note that you will have to create these figures from scratch; for this milestone you cannot use any library that generates domain specific (hockey) figures for you. You will be provided with a sample ice rink image that has the correct ratio. To create these figures, you must: - Ensure you can work with the event coordinates correctly. This includes ensuring the shots are on the correct side of the rink (due to period changes, or start on different sides during a game), as well as being able to map from physical coordinates to pixel coordinates on the figure. - Compute aggregate statistics of shot locations across the entire league to compute league averages - Group shots by team, and use the league averages computed above to compute the excess shots per hour. You can choose to represent this as either a raw difference in goals between the teams, or a percentage. - Make appropriate choices to bin your data when displaying it. You could also consider using smoothing techniques to make your shot maps more readable. A common strategy is to use kernel density estimation with a Gaussian kernel. - Make the plot interactive, with options to select the team and season. The easiest way to do this is using something like plotly or bokeh. A nice simple demo of what you could do with plotly can be found here. 12 Due: IFT 6758 2021 - Milestone 1Oct. 15, 2021 Source: www.hockeyviz.com. Example offensive shot map for the San Jose Sharks, over the 2017-2018 You do not need to compute the expected goals for/60, or number of minutes in the offensive zone. Questions 1. Export the plot to HTML, and embed it into your blog post. Your plot must allow users to select any season between 2016-17 and 2020-2021, as well as any team during the selected season. Note: Because you can find these figures on the internet, answering these questions without producing these figures will not get you any marks! 2. Discuss (in a few sentences) what you can interpret from these plots. 3. Consider the Colorado Avalanche; take a look at their shot map during the 2016-17 season. Discuss what you could say about the team during this season. Now look at the shot map for the Colorado Avalanche for the 2020-21 season, and discuss what you could conclude from these differences. Does this make sense? Hint: look at the standings. 13 Due: IFT 6758 2021 - Milestone 1Oct. 15, 2021 4. Consider the Buffalo Sabres, which have been a team that has struggled over recent years, and compare them to the Tampa Bay Lightning, a team which has won the Stanley for the past two years in a row. Look at the shot maps for these two teams from the 2018-19, 2019-20, and 2020-21 seasons. Discuss what observations you can make. Is there anything that could explain the Lightning’s success, or the Sabres’ struggles? How complete of a picture do you think this paints? Note: the point of this exercise is to get you comfortable with using the standard Python libraries to create visualizations. You cannot use any tool that creates domain-specific (i.e. hockey) visualizations for you. You are free to rely on stock libraries (matplotlib, seaborn, plotly, bokeh, etc) to generate these plots. 7. Blog Post (up to 30% penalty) To wrap everything all up, create a blog post using the provided template containing all of the required figures, answers, and discussion mentioned in the previous section. You will not need to actually deploy the blogpost so that it’s publicly accessible, but instructions will be provided for how to do this if you would like to show off your awesome project on your resume after the course is done! If you do not submit your content in a blog post format (e.g. as a jupyter notebook), you cannot score better than 70% on your milestone. We suggest getting started getting a working environment for the blog post early on, as it will be much easier to answer questions and add figures in the blog post as you are working on the project, rather than trying to get this set up right before the deadline! Once your environment is up and running it’s very simple to work with, but we anticipate getting setup may take a little bit of time! Group Evaluations In addition to the evaluations mentioned above, for each milestone you will be asked to score yourself and your teammates with respect to how much you think everyone contributed to this milestone. For a team of size n, everyone in the team will have n x 20 points to allocate between everyone in your group (including yourself). In an ideal situation, everyone will contribute to the project equally and thus everyone will assign 20 points to every teammate. However, in the case where some people contributed less than others, you could assign them less points and give those points to who you think contributed more as a result. If you assign someone a score between 19-21, no explanation is required. If you assign someone a score outside of this range, you will be required to submit a short paragraph explaining why this score is warranted. Extreme cases will result in an instructor following up with the team to resolve any potential difficulties. This could include auditing git history in your repositories. 14 Due: IFT 6758 2021 - Milestone 1Oct. 15, 2021 Your final mark will then be scaled by the average of all of the scores that were assigned to you (divided by 20). As an example, we show a non-ideal case where the workload was not fairly distributed across the group. Take a group of 4, where the final project score was 95% and everyone gave person A 20 points, their grade would not be affected: [(20 + 20 + 20 + 20) / 4] / 20 = 1.0 * 95% = 95% However for this same group, if person B did not contribute much, their final grade may suffer: [(15 + 16 + 16 + 15) / 4] / 20 = 0.775 * 95% = 73.625% Perhaps person C & D picked up the slack, and their scores reflect this - their final grades may be bumped up as a result. This example is summarized in the table below. In general we do not expect teams to have any issues. We hope that by laying out a clear method for evaluating each other and how this may directly affect your grade, people are incentivised to cooperate and contribute equally to the project. In the event of any conflict or concerns with the group, we encourage you to try to resolve it as quickly as possible. If you require the support of the instructors to resolve any issues or concerns, please contact us as early as possible to resolve it. Table 1: Sample group evaluations for a team of 4, which scored 95% on the milestone. (down) person who gave a score (across) Person assigned a score A B C D A 20 15 21 24 B 20 16 22 22 C 20 16 22 22 D 20 15 21 24 Multiplier 1.0 0.775 1.075 1.15 Final Score 95% 73.625% 102.125% 109.25% Useful References ● IFT6758 Hockey Primer ● Unofficial NHL API Documentation ● Cookiecutter Data Science (A useful tool to help template your git repos) 15