xuebaunion@vip.163.com
3551 Trousdale Rkwy, University Park, Los Angeles, CA
留学生论文指导和课程辅导
无忧GPA:https://www.essaygpa.com
工作时间:全年无休-早上8点到凌晨3点
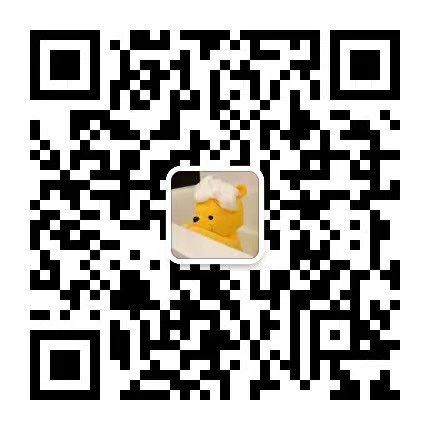
微信客服:xiaoxionga100
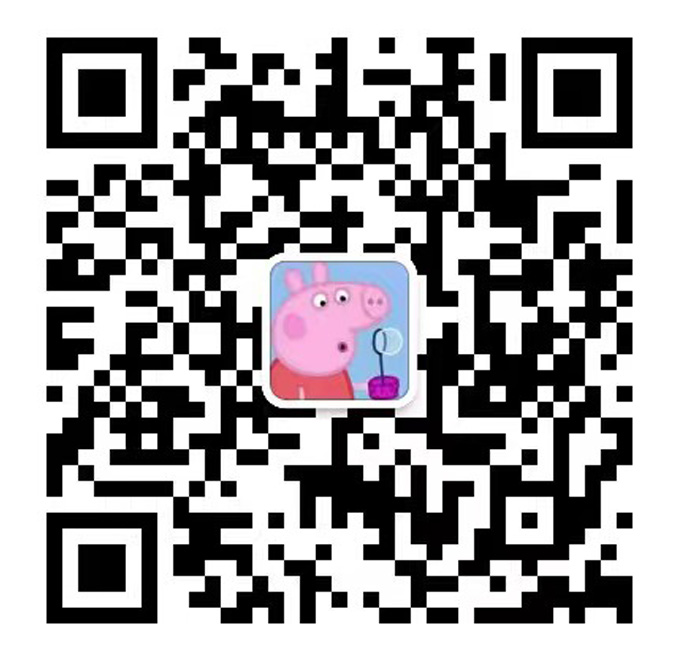
微信客服:ITCS521
EC393: Fall 2020 Midterm Exam – Suggested Solutions I. The Impact of QuestBridge on Economic Diversity QuestBridge (QB) is a national nonprofit that, according to their website, connects the nation’s most exceptional, low-income youth with leading colleges and opportunities. QuestBridge aims to increase the percentage of talented low-income students attending the nation’s best colleges and to support them to achieve success in their careers and communities. QB currently works with 42 Partner institutions such as Amherst, Bowdoin, Columbia, USC, Yale, and Williams, and – as of 2016 – Colby. QB student finalists apply to up to 12 schools in the fall of their senior year through QB’s own application system. If they are successfully matched with one of their ranked schools, they receive a binding early-decision acceptance and the partner institution guarantees a full scholarship. Partner institutions love working with QB – President Greene spent a good amount of time trying to get Colby into the organization – in part for its ability to serve as a recruiter for students who they may otherwise miss in the application process. We care about estimating the effect of being a QuestBridge school on economic diversity of the student body. As their stated goal is to increase the representation of low-income youth at selective colleges, one marker of this outcome is the percent of students who receive federal Pell grant funding. Pell grants provide college funding for students from families with incomes of approximately $50,000 a year or less. For reference, the median family income of students at Colby is around $235,000 a year and the median family income of QB students is $35,000 (across all partner schools). To look at this question, I went and pulled data on the top 50 liberal arts colleges from 2018, the most recent year available, and flagged the 21 schools in this set that are QB Partners. I then ran the following regression: = 0 + 1 + 2 ln( ) + (1) where is the percent of the first-year class that is Pell grant eligible, is an indicator equal to one for the 21 QuestBridge partners and 0 for the other schools, and ln( ) is the natural log of the endowment per student. For Colby specific reference, with 14% of first-year students in the 2018 class receiving Pell grants, an endowment of $880m, and enrollment of 1917 students, these values would look like: = 14; = 1; ln( ) = ln ( 880,000,000 1917 ) Column 1 below reports the results: Table 1: First-Year Pell Percent and QuestBridge (1) (2) QuestBridge Partner School -0.845 -0.845 (2.178) (2.178) ln(Endowment per Student) -2.250 (1.090) ln(Endowment per Student in $1m) -2.250 (1.090) Constant 24.002 20.884 (1.550) (1.724) Observations 50 50 R-squared 0.140 0.140 Standard errors in parentheses; *’s omitted 1. How do you interpret the estimates of ̂ and ̂ in column 1? (2 pts) Being a QuestBridge partner is related to a 0.845 percentage point reduction in the predicted first year Pell rate holding log endowment per student constant. A 1% increase endowment per student is related to a 0.0225 percentage point reduction in the predicted first year Pell rate holding QuestBridge partner status constant. 2. What do you conclude about the nulls of : = and : = ? (2 pts) (Note I prevented stars from appearing on the table above and the t-critical value for a 5% two- tailed test with 47 degrees of freedom, is 2.00). For 1 : the t-stat is |-0.845/2.178| which is far < than 2.00 so fail to reject For 2 : the t-stat is |-2.250/1.090| which is > than 2.00 so reject the null 3. **Let represent the QB indicator and the log endowment per student variable. Is the following true? How do you know. (2 pts) ∑ 1̂ 50 =1 = ∑ xs2̂ 50 =1 Yes, these both equal zero as the correlation between each x and the residuals in an OLS regression is 0. Note 1: saying that ∑ ̂ = 0 implies that the above is true is not correct as ∑ ̂ ≠ ∑ ̂ – i.e. you can’t pull out out of a sum that’s indexed by . Note 2: It’s true that the residuals sum to zero – it’s not true that each residual is zero. Saying all of the residuals are zero is the equivalent of saying R2 is always 1. 4. **Does the estimate in column 1 reflect the unbiased, ceteris paribus effect of QuestBridge on the first-year Pell rate? Why or why not? (3 pts) No. Because schools and QB choose who is in the program, there are likely factors in the error term that are correlated with QuestBridge Status that cause bias in the ̂1 estimate. Note 1: Saying that there are factors in the error term is not sufficient – it’s only if they’re correlated with the QuestBridge indicator that they’d be causing bias. Note 2: Saying QuestBridge was not randomly assigned is not sufficient – you need to tell us why not being randomly assigned creates a bias issue. 5. Would your answer to #4 change if I added controls for the admissions rate, cost of attendance, and median SAT scores to the regression in column (1)? Why or why not? (2 pts) No – unless we accounted for all of the variables in the original error term that were correlated with QuestBridge partnership, we’d still have a bias concern. Pulling these three out may help get us closer to ceteris paribus, but we’d likely not yet be there. Column 2 calculates the log-endowment per student after rescaling the endowment variable to be in millions of dollars per student. For example, with Colby’s $880m endowment, this would be ln ( 880 1917 ) in Column 2 instead of ln ( 880,000,000 1917 ) in Column 1. Everything else is left the same between the regressions in columns 1 and 2. 6. Why are the two endowment coefficients the same after rescaling? (2 pts) Because we’re looking at the natural log of endowment per student, we care about percent changes so the units of the actual variable we’re taking the log of do not matter. A published study on this question built a panel dataset of the top-50 Liberal Arts Colleges from 2000 to 2014 (so a total sample of 750 observations, 50 schools x 15 years). Their QuestBridge indicator is similar to what I used above – it turns on to 1 when the QB Partner joins and stays on for all years after. This is similar to your problem set question that tracked states before and after they passed medical marijuana laws. 7. **What is the counterfactual of interest when examining the causal effect of QuestBridge on first-year Pell rates? How does this research design differ in its approach compared to the single 2018 cross-section of 50 observations I used above? (4 pts) For a school that’s a QuestBridge partner, we’d like to know what the first year Pell rate would be at that same school if they were not a QB partner. And vice versa for a non-QB school; we’d like to know what their FY Pell rate would look like if they were a QB partner. The 2018 cross section estimates this by comparing across or between schools – i.e. we use information on somebody like Middlebury (non-QB) to compare to Colby (QB). This updated research design allows for that same comparison but also adds the ability to compare a school to itself before vs. after joining QB. As part of this project the authors took a particular interest in schools, like Colby, with no-loan financial aid policies. These schools meet the full demonstrated financial need of applicants (as determined by the US government) without using loans – you may still end up with loan debt if you need to take out loans on top of the government’s calculated expected financial contribution for your family, but it will likely be significantly less than at other institutions. They estimate the following regression with an indicator that marks if school s in year t is a QB partner, and is an indicator for having a no-loan financial aid policy. is a vector of additional school level controls that includes log-endowment per student. = 0 + 1 + 2 + 3 + 4 + (2) Their results are in column 1 below. Table 2: FY Pell Percent and Financial Aid (1) QuestBridge Partner -0.21 (0.45) QuestBridge Partner X No-loan School 1.91 (0.20) No-loan School 0.53 (0.15) Observations 750 Standard errors in parentheses 8. Why do they need to have the No-loan indicator by itself as well as the interaction? (2 pts) This guarantees that the combined interactive effect of QBxNo-loan is accurately measured as it allows for a shift in FY Pell rates just for being a No-loan school. Note: referring to “slopes” here is a bit misleading as these are all binary so better to say “marginal effect.” For example “letting the slopes vary” vs. “letting the marginal effect of QB vary” 9. **What do the results tell you about the impact of QB Partnership at schools with no- loan programs? How does this compare to what you learned from Table 1? (3 pts) QB partners with no-loan programs experience a 1.91 point greater effect on their FY Pell rate than QB Partners without no-loan financial aid programs. This is quite different than the -0.845 found in Table 1; the difference could be because of the interactions with no-loan, the sample changing, the additional control variables or some combination of those differences. Note: it is not true that the effect of QB at no-loan schools is 1.91 points – that is the difference in the effect of QB at schools w/o no-loan policies (the -0.21) and schools with no- loan policies. The effect of QB at schools with no-loan policies is 1.70 (-0.21 + 1.91). Colby joined QuestBridge in 2015 (or, more accurately, QuestBridge accepted Colby in 2015). This means the 2016 entering class was the first to include QuestBridge scholars. I pulled Colby’s first- year class Pell numbers from 2011-2020 and ran the following regression where is an indicator equal to 1 for 2016 onward and 0 before: = 0 + 1 + (3) I got the following results: ̂ = 10.4 + 3.2 10. What do ̂ and ̂ represent? (3 pts) ̂0 is the average FY Pell rate for 2011-2015, before Colby was a QB partner ̂1 is the difference in the average FY Pell rate for 2016-2020 relative to 2011-2015, after Colby was a QB partner. (So the FY Pell average between 2016-20 was 13.6% compared to 10.4% pre-QB). 11. Is (, ) = ? How do you know? (2 pts) No. The cov(fypell, QB) is the numerator of the 1 estimate which is 3.2 so the covariance cannot be zero. If instead of the regression in equation (3), we estimated the following: = 0 + 1( ) + where ( ) is an indicator for when Colby was not a QB Partner pre-2016: 12. How would ̂ compare to ̂? Why? (2 pts) It would be equal to −1 as it would show the post-QB average of 13.6 (which would be ̂0) was reduced by 3.2 percentage points pre-QB down to 10.4. II. 2016 Election With 26 days left to the 2020 election, let’s take a look back at what happened 4 years ago. The data for this exercise comes from the 2016 American National Election Survey (ANES). This is information on a representative sample of the voting-eligible population at the time of the 2016 election. I’ve selected out those who actually voted (~60% of the voting age population), so everyone in the data either voted for Hillary Clinton, Donald Trump, or a third-party candidate. Recall that President Trump lost the popular vote to Hillary Clinton 46% to 48%, but won the Presidency due to the distribution of the vote across states and the electoral college system. Let’s first look at the relationship between voting for President Trump and age. 13. Estimate a nonparametric regression of voting for Trump against age. Paste in your graph here: (2 pts) 14. Based on your graph, explain if it appears President Trump gained over 50% of votes in any age range. If so, where? (2 pts) I added the line at 50% in the graph above to help see this – it looks like around 70 it crosses, so for those 70 and above. Now we’ll look at a set of multivariate LPMs predicting who voted for President Trump. 15. Estimate the following ( ) = 0 + 1 + 2 2 + and report your results in column 1 of your table. (2 pts) See column 1 of results table. 16. Are age and age2 each statistically significant at the 5% level? Are they jointly statistically significant? (3 pts) They are not – the p-value for 0: 1 = 0 is 0.076 and the p-value for 0: 2 = 0.657; both are greater than 0.05 so fail to reject the nulls implying neither is statistically significant. They are jointly significant though as the p-value for the joint null of 0: 1 = 2 = 0 is < 0.01 so reject that null implying joint statistical significance. 17. Briefly explain how your answer to the first part of #16. is consistent with your answer to the second part of #16. (2 pts) age, and age2 are highly correlated. This means that when we look at their individual coefficients and ask “how much variation in age is left after holding age2 fixed?” the answer is “very little.” This inflates the individual standard errors for 1and 2 as the 2 in their denominator is very close to 1. The joint test is looking at the overall predictive point of age and age2 combined so is not impacted by this – as you saw in the first picture, there’s a clear positive relationship between age and voting for President Trump that’s captured by their joint statistical significance. It’s a bit tough to interpret the actual ̂’s from the quadratic specification, so let’s use the age category indicators, or brackets, instead. There are indicators for being in the following age groups: 18-34; 35-49; 50-64; 65 and over. 18. Estimate a LPM of voting for President Trump against the age brackets and report your results in column 2 of your results table (2 pts) See column 2 – you needed to include 3 of the 4 age group indicators; any 3 would have been fine. 19. **How do you interpret the coefficient estimate on the age 50 to 64 indicator? Is it statistically significant? How do you know? (3 pts) (This would be dependent on what age category you omitted – I omitted 18-34 so that becomes the reference point that’s measured in the constant). Those between the age of 50 and 64 were 13.8 percentage points more likely to vote for President Trump than those between 18 and 34. It is statistically significant as the p-value is less than 0.01 20. Generate predicted probabilities and plot them against age. Paste your graph here: (2 pts) 21. Explain why your predicted probabilities here take this specific shape in relation to what you estimated in #18. (2 pts) The estimated regression forces everyone in the same age bracket to have the same predicted probability of voting for President Trump which creates a step-looking picture where there are discrete jumps at the age cut offs of 34, 49, and 64 and then flat between those points. It follows the general upward trend (increasing steps) of the picture in 13. It was clear in the 2016 election returns that the race of the voter mattered in predicting their vote. The variable white is an indicator for self-identifying as a white voter. 22. Repeat your regression in #18 including interactions of the age groups indicators with the white variable to measure the probability of voting for President Trump in each of the different age-white/non-white groups. (i.e. white 18-34 year olds, non-white 18-34 year olds, etc.). Report your results in column 3 of your results table. (2 pts) See column 3 – there are now 8 groups to care about (4 age groups X 2 race categories). You needed 7 coefficients to get all of these. 23. **Based on your regression results, what fraction of 18-34 year old white voters and non- white voters voted for Trump? (2 pts) 18-34 year white voters: 43.3% 18-34 year non-white voters: 12.1% Based off of what I estimated the 12.1% comes from the constant and the 43.3% from the constant plus the age1834Xwhite interaction coefficient. Your regression may have looked different but could still deliver these same numbers. 24. Based on your results, the difference between white and non-white voters was largest in which age group? How do you know? (3 pts) The largest interaction term is age65plusXwhite – this tell us the difference is largest between white and non-white voters in this group. There is much, much more in the dataset than age and race. On this final question, I want you to use the ANES data to test some interesting hypothesis of yours regarding the 2016 Presidential election. I’ve cleaned and coded the variables you’ll see at the top of the dataset if you describe the data like self-reported gender, education level, marital status, and income bracket, but you’re free to use anything in here. The only requirements for this question are that you: - Use a LPM - Look at something actually interesting to you - Include at least two x-variables 25. Report your results in column 4 of your results table and BRIEFLY interpret the findings in words (4 pts).Lots of good answers here. (1) (2) (3) VARIABLES votetrump votetrump votetrump age3549 0.067** 0.078 (0.028) (0.050) age5064 0.138*** 0.093* (0.027) (0.048) age65plus 0.168*** 0.072 (0.027) (0.056) age1834Xwhite 0.312*** (0.042) age3549Xwhite 0.284*** (0.042) age5064Xwhite 0.344*** (0.038) age65plusXwhite 0.372*** (0.047) age 0.006* (0.003) age2 -0.000 (0.000) Constant 0.188** 0.335*** 0.121*** (0.076) (0.020) (0.035) Observations 2,768 2,768 2,768 R-squared 0.021 0.016 0.097 Standard errors in parentheses *** p<0.01, ** p<0.05, * p<0.1 Exam stats: Distribution (in % - your score out of 60); highest: 99.6%; mean of 84.6; median of 85.4 Letter grade breakdown As 24% Bs 56% Cs 16% < Cs 4%