xuebaunion@vip.163.com
3551 Trousdale Rkwy, University Park, Los Angeles, CA
留学生论文指导和课程辅导
无忧GPA:https://www.essaygpa.com
工作时间:全年无休-早上8点到凌晨3点
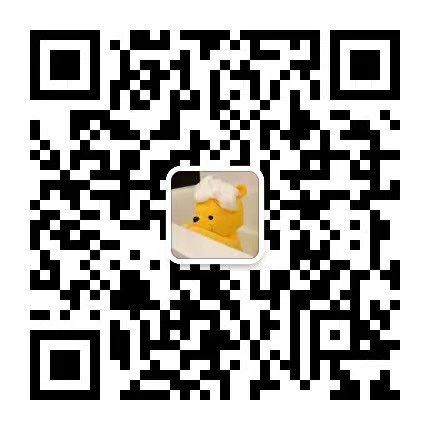
微信客服:xiaoxionga100
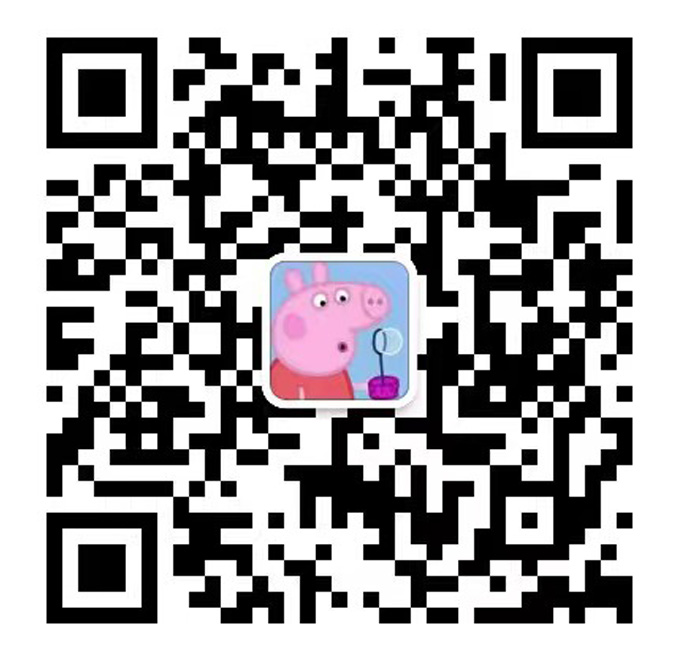
微信客服:ITCS521
COMP3160 ARTIFICIAL INTELLIGENCE Assignment 2 (Weight: 20%)
Evolutionary Algorithms for Adversarial Game Playing Draft Submission
Due: 11:55pm, Oct 29, 2021 (Friday, Week 12) Final Submission Due:
11:55pm, Nov 05, 2021 (Friday, Week 13) The goal of this assignment is
to appreciate the efficacy of Evolutionary Algorithms, specif- ically
Genetic Algorithm (GA), in the context of game theory. You will be using
the DEAP package for Genetic Algorithm in order to evolve strategies
for repeatedly playing variants of The Tragedy of the Commons (TC).
Basically, there is a group of farmers (herdsman) in a village, and
there is a community grazing area (“Commons”). If the farmers limit the
number of cows that each of them is allowed to graze in the Commons,
everyone prospers. If a few farmers flout the convention, they benefit
at some cost to others. But if too many farmers flout the convention,
then everyone suffers due to over-grazing. This game is quite pertinent
to the sustainability issue. It is interesting in this context to read
the obituary, Eli- nor Ostrom: An uncommon woman for the commons, that
Kenneth J Arrow and colleagues wrote for an unusual political scientist
who received Nobel Prize in economics. The Tragedy of the Commons is
originally described by Hardin1 as follows: As a rationale being, each
herdsman seeks to maximize his gain. Explicitly or implic- itly, more or
less consciously, he asks, “what is the utility to me of adding one
more animal to my herd?” This utility has a negative and a positive
component. 1. The positive component is a function of the increment of
one animal. Since the herdsman receives all the proceeds from the sale
of the additional animal, the positive utility is nearly +1. 2. The
negative component is a function of the additional overgrazing created
by one more animal. Since, however, the effects of overgrazing are
shared by all [. . . ], the negative utility for any particular
decision-making herdsman is only a fraction of 1. Adding together the
component particular utilities, the rational herdsman concludes that the
only sensible course for him to pursue is to add another animal to his
herd. And another; and another... But this is the conclusion reached by
each and every rational herdsman sharing a commons. Therein is the
tragedy. Each man is locked into a system that compels him to increase
his herd without limit – in a world that is limited. Ruin is the
destination towards which all men rush, each pursuing his own best
interest in a society that believes in the freedom of the commons.
Freedom in a commons brings ruin to all. We will look at two very
simplistic models of this imagined scenario. Both of them are two-player
games. In the first model (TC1), we assume a small grazing common land
that can comfortably support two cows altogether, and each cow brings 5
units of utility to its owner. If one more 1Hardin, G. “The Tragedy of
the Commons.” Science 1968, 162, 1243–1248. 1 cow is allowed to graze,
the cows are not adequately fed, and the benefit from each cow reduces
by 1. If instead two more cows are allowed in, the feed is fully
inadequate for every cow because of overgrazing, and they bring zero
benefit each to their owners. It is assumed that there is a tacit
understanding between the two farmers that they will cooperate, that is,
will allow in only one cow each. The payoff matrix for this model is
given as follows: Table 1: The Tragedy of the Commons: TC1 ROW C D
COLUMN C (5, 5) (4, 8) D (8, 4) (0, 0) Payoffs to: (Column, Row) The
second model (TC2) changes the narrative to some extent. The local
council de- cides that the two farmers can take a lease of part of the
“commons” for a nominal fee, and use that part exclusively for grazing
their own cows. However there is a constraint: each can take an
independent decision of whether to lease 1 3 of the commons, or 1 4 .
Either way, part of the commons will be left un-leased, and they will
have equal grazing right over that un-leased part. Here we assume that
the total commons has an area of 24, and each unit area provides one
unit of benefit. Again there is the tacit understanding that the two
farmers will cooperate, that is each will take out a lease on 1 4 of the
commons. However there is incentive to defect, as the payoff matrix
shows below. For instance, if one farmer cooperates, and the other
defects, the cooperator receives 24 4 + 24−( 24 4 + 24 3 ) 2 = 11 units
of benefit, while the defector will receive 24 3 + 24−( 24 4 + 24 3 ) 2 =
13 units. Table 2: The Tragedy of the Commons: TC2 ROW C D COLUMN C
(12, 12) (11, 13) D (13, 11) (12, 12) Payoffs to: (Column, Row) 2 Task
Specification The main issue is the representation of a strategy for
playing a game like TC1 or TC2 as a bit string. Two papers are provided
in the Assignment folder that will help you with this. You are
recommended to start with the paper, an easy read, by Adnan Haider. You
might want to also read the paper by Golbeck, if you find the topic
interesting. Although it is possible to represent players/strategies in
very many different ways, for parity in marking, you are strongly
advised to employ the following representation: Figure 1: Representation
of two players. With d as the memory depth, m = 22d. Total length of a
player/chromosome: 22d + 2d. 1. BACKGROUND KNOWLEDGE ASSESSMENT [5
marks] (a) Consider the Cryptocurrency Mining. You may want to read this
New Yorker article about the environmental impact of Bitcoin mining.
Which of the two models of the Tragedy of the Commons, TC1 and TC2, is
closer in spirit to this situation? Answer this question in no more than
50 words. (b) Find all Dominant Strategy Equilibria, Nash Equilibria
and Pareto Optimal Strategy Profiles for the game TC1. (c) Find all
Dominant Strategy Equilibria, Nash Equilibria and Pareto Optimal
Strategy Profiles for the game TC2. (d) Analyse the results you obtained
in items 1b and 1c above. Describe any inter- esting difference not
noted between them. Why did you find those differences interesting?
Answer this question in no more than 50 words. (e) Axelrod’s tournaments
of Iterated Prisoner’s Dilemma show that that co-operation among
players can emerge in a world of selfish agents. What do you ex- pect
would happen if different strategies were to play Iterated TC1
(henceforth (ITC1) instead? What would you expect in the case of
Iterated TC2 (henceforth (ITC2)? Explain your answer in no more than 50
words. Give particular at- tention to any difference in what you woudl
expect in these two cases. 3 2. IMPLEMENTATION IN PYTHON [10 marks] (a)
Implement the function: payoff_to_player1(player1, player2, game):
returns payoff Note: player2 is the adversary, and payoff is determined
by latest moves obtained from respective appropriate memory locations
and the payoff matrix for the relevant game (TC1 or TC2). Assume
memory-depth of 2. (b) Implement the function: next_move(player1,
player2, game, round): returns player1’s move Note: player1’s next move
is based on both the players’ earlier moves and player1’s strategy
(encoded in player1’s chromosome). The move to be returned can be C/D or
0/1 depending on your representation. Note that in early rounds some
default moves are made. Assume memory-depth of 2. (c) Implement the
function: process_move(player, move, memory_depth): returns success /
failure Note: player’s relevant memory bits are updated based on its
latest move move and its memory depth. (d) Implement the function:
score(player1, player2, m_depth, n_rounds, game): returns score to
player1 Note: player1 iteratively plays the game game against player2
for n rounds number of rounds, and its score is returned. (e) You will
be using the DEAP package for genetic evolution of strategies. As- sume a
memory depth of 2. i. Implement genetic evolution of strategies for
playing ITC1. ii. Modify the code you wrote as part of Task 2(e)i above
so as to genetically evolve strategies for playing ITC2. 4 3. ANALYSIS
[5 marks] (a) What is the best ITC1-strategy that you generated in Task
2(e)i via GA? What parameters (fitness function, type of crossover,
mutation rate, etc.) did you use, and why? (b) Describe in English the
behaviour of that ITC1-strategy (that you identified in Task 3a above).
Does it align with the prediction you outlined in Task 1e earlier?
Explain in no more than 50 words. (c) What is the best ITC2-strategy
that you generated in Task 2(e)ii via GA? What parameters (fitness
function, type of crossover, mutation rate, etc.) did you use, and why?
(d) Describe in English the behaviour of the ITC2-strategy (that you
identified in Task 3c above). Does it align with the prediction you
outlined in Task 1e ear- lier? Explain in no more than 50 words. (e)
Take an appropriate sustainability issue, and outline in no more than 50
words the implications that your results have in that context. What to
Submit, and When You will submit two files:
1.