xuebaunion@vip.163.com
3551 Trousdale Rkwy, University Park, Los Angeles, CA
留学生论文指导和课程辅导
无忧GPA:https://www.essaygpa.com
工作时间:全年无休-早上8点到凌晨3点
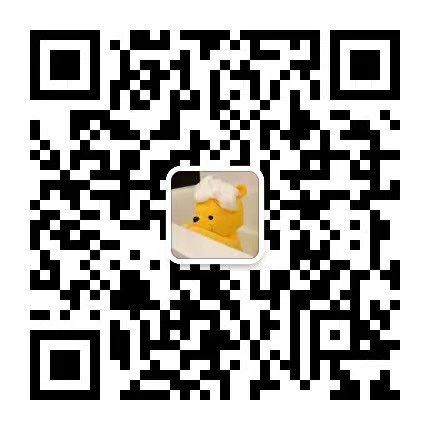
微信客服:xiaoxionga100
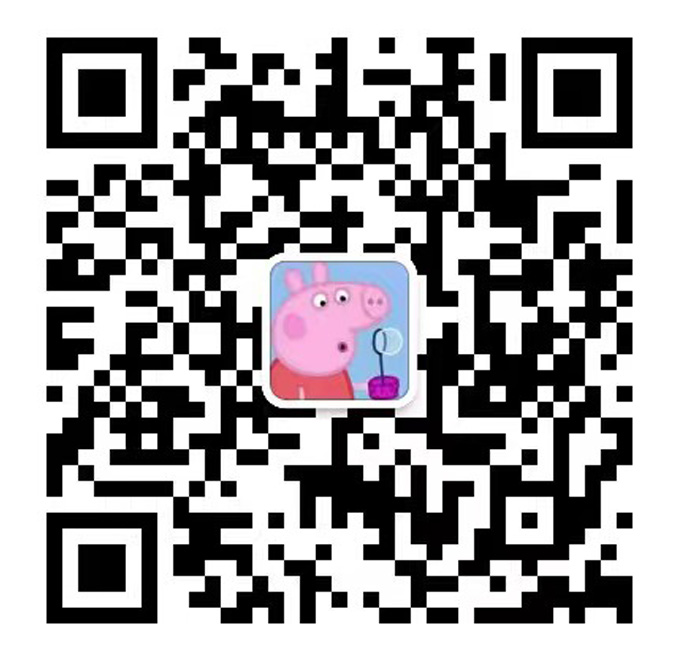
微信客服:ITCS521
All questions below relate to the following scenario: X = (X1, . . . , Xn) consist of independent and identically distributed Poisson(θ) random variables, for some unknown θ ∈ Θ = (0, ∞). 1. Write down the likelihood. 2. Determine the Cram´er-Rao Lower Bound to the variance of an unbiased estimator of θ. 3. Show that the sample mean ¯X = 1n Pni=1 Xi is minimum variance unbiased. 4. Specify exactly the uniformly most powerful test at level 0.05 of the null hypothesis H0 : θ = 1 against the alternative hypothesis H1 : θ < 1 when n = 4; note that ee4 ≈ 0.0183. 5. Consider the decision problem with decision space D = Θ = (0,∞) and loss function L(d|θ) = (d d θ)2 . Determine the form of dflat(X), the Bayes procedure using the “flat prior” weight function w(θ) ≡ 1 and show that its risk is given by Eθ [L (dflat(X)|θ)] = θ2n + 1n2 . 6. For the same decision problem in the previous question, for any 0 ≤ a < b < ∞, determine limn→∞ max a≤θ≤b nEθ [L (dflat(X)|θ)] . turn to page 3 Useful Formulae Probability distributions Discrete distributions • Bernoulli X has a Bernoulli (p) distribution if P(X = 1) = p, P(X = 0) = 1 鮶 p. E(X) = p, V ar(X) = p(1 鮶 p). • Binomial X has a Binomial (n, p) distribution if for x = 0, 1, . . . , n, P(X = x) = 鮶nxpx (1 鮶 p)n−x. E(X) = np, V ar(X) = np(1 鮶 p). • Poisson X has a Poisson (λ) distribution if for x = 0, 1, . . . , P(X = x) = e−λλx/x!. E(X) = V ar(X) = λ. Continuous distributions • Uniform X ∼ U(a, b), b > a, then X has density fX(x) = 1/(b 鮶 a) for x ∈ (a, b), 0 otherwise. E(X) = (a + b)/2, V ar(X) = (b 鮶 a)2/12. • Normal X ∼ N(0, 1), then X has density fX(x) = (2π)鮶1/2e−x2/2. E(X) = 0, V ar(X) = 1. Y ∼ N(µ, σ2 ), then (Y 鮶 µ)/σ ∼ N(0, 1). • Gamma X ∼ Gamma(α, β), then X has density fX(x) = 1 βαΓ(α)xα鮶1e−x/β for x > 0 , Γ(·) is the Gamma function, Γ(α) = (α 鮶 1)!, Γ(1) = 1. E(X) = αβ, V ar(X) = αβ2 . Here β is a scale parameter; 1/β is also called the rate parameter. • Exponential X ∼ Exponential(β) is the same as X ∼ Gamma(1, β). Here the scale parameter β is also the mean. • Inverse Gamma X has an Inverse Gamma(α, λ) distribution, then X has density fX(x) = λαe−λ/x xα+1Γ(α) for x > 0 . Note then that Y = X−1 has an ordinary gamma distribution with shape α and rate λ; E(X) = λ/(α 鮶 1), V ar(X) = λ2/ (α 鮶 1)2(α 鮶 2). • Beta X∼Beta(α, β), X has density fX(x) = xα鮶1 (1 鮶 x)β鮶1 B(α, β) for 0 < x < 1 , B(α, β) = Γ(α)Γ(β) Γ(α+β) is the beta function; E(X) = α/(α + β), V ar(X) = αβ (α + β)2(α + β + 1) . 5 • Pareto X has a Pareto(α, m) distribution, then X has density fX(x) = αmα xα+1 for x ≥ m , E(X) = αm/(α 鮶 1) for α > 1 (+∞ otherwise), V ar(X) = αm2 (α 鮶 1)2(α 鮶 2) for α > 2 (+∞ for 1 < α ≤ 2, undefined otherwise). Convergence • Convergence in distribution: A sequence of random variables X1, X2, . . . is said to converge in distribution to the continuous CDF F if for any sequence xn → x and real x as n → ∞, P(Xn ≤ xn) → F(x). If this holds then it also holds with ≤ replaced by <. If F(·) is the N(0, σ2 ) CDF we also write Xn d→ N(0, σ2 ). • Central limit theorem: If X1, . . . , Xn are iid random variables with mean µ and variance σ2, then as n → ∞, Pni=1 Xi 鮶 nµ √ nσ2 d→ N(0, 1). • Asymptotically Normal: If √n (Xn 鮶 µ) d→ N(0, σ2 ) then we write Xn ∼ AN µ, σ2n and say the sequence {Xn} is asymptotically normal with asymptotic mean µ and asymptotic variance σ2n . • Delta Method If Xn ∼ AN µ, σ2n and the function g(·) has derivative g0(µ) at µ then g (Xn) ∼ AN g(µ), g0(µ)2σ2 n . Transformation of random variables • One variable: Suppose X has density f(x), consider y = u(x) where u(·) is a differentiable and either strictly increasing or strictly decreasing function for all values within the range of X for which f(x) 6= 0. Then we can find x = w(y), and the density of Y = u(X) is given by g(y) = f(w(y)) · |w0(y)| for all y with corresponding x such that f(x) 6= 0, and 0 otherwise. • Extension of one variable: Suppose (X1, X2) has joint density f(x1, x2), consider Y = u(X1, X2). If fixing x2, u(·, x2) satisfies the conditions in the one-variable case, then the joint density of (Y, X2) is given by g(y, x2) = f(x1, x2) · ∂x1 ∂y , where x1 needs to be expressed in terms of y and x2. Fixing x1 is similar.