xuebaunion@vip.163.com
3551 Trousdale Rkwy, University Park, Los Angeles, CA
留学生论文指导和课程辅导
无忧GPA:https://www.essaygpa.com
工作时间:全年无休-早上8点到凌晨3点
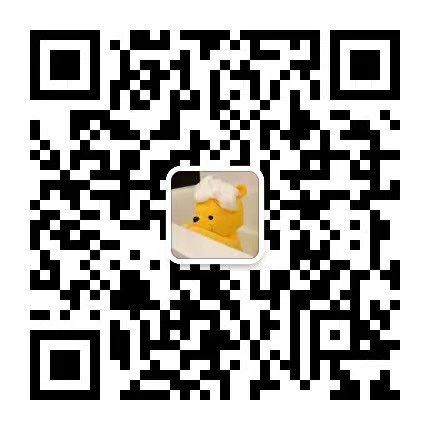
微信客服:xiaoxionga100
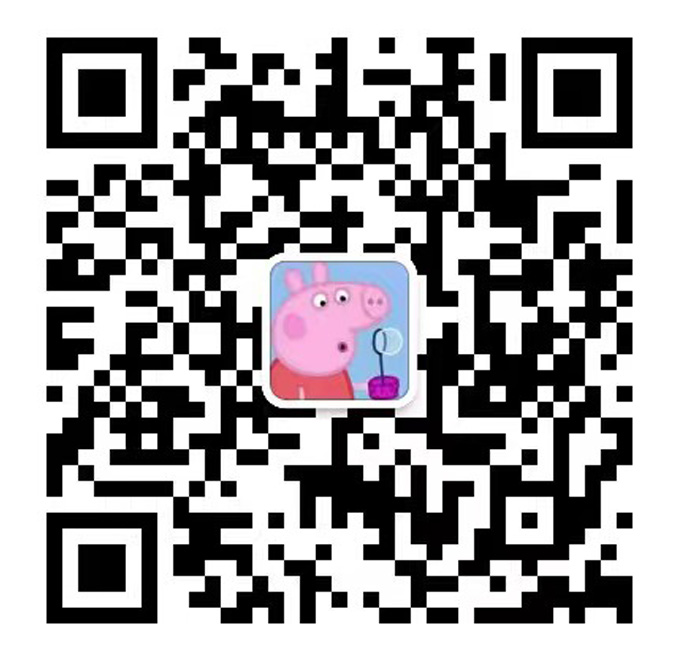
微信客服:ITCS521
MSFI 435 Module 9: Data Page 9-1 9. Data Objectives Discuss the need of and the hardships in collecting data for an empirical analysis Analyze the determinants of executive compensation Analyze the uncertainty in predictions extracted from regression models MSFI 435 Module 9: Data Page 9-2 9.1. An Examination When Data is Ready You are interested in what are the determinants of executive compensation. In particular, you want to examine whether the compensation of the Chief Executive Officer (CEO) or a public company is related to (or depends on) the performance of the firm she manages. Figure 9-1. Table with data ready to go Suppose you have access to a table with information on CEO compensation (let’s say, the total amount of cash the CEO took home in year the 2000) and the performance of its firm (let’s say, the average monthly return on the firm’s stock, plus the measure of return on asset, both measured in 1999), as shown in Figure 9.1. Then you can run a regression model and examine your idea. For example, you can formally test the hypothesis that (past) performance matters for CEO compensation. If you are interested in performance as proxied by stock market performance, then you are testing H0: βAVGRET = 0 Ha: βAVGRET ≠ 0 If you are interested in whether any of the proxies for performance matters, then you can run a multiple hypotheses test as in H0: βAVGRET=βROA=0 Ha: βAVGRET ≠ 0 or βROA ≠ 0 You can examine the magnitude of the coefficients, the t-statistics based on single hypothesis, the F-statistic for multiple hypotheses, the explanatory power of your model, etc. The econometrics of your analysis—the set of statistical tools employed in your study—comes directly from our discussion of multiple regression models. Later on, though, the danger of an omitted variable bias comes to mind, and you realize that this regression model is too simplistic—that you need to add other explanatory variables. For example, you might need to control for the size of the firms, as larger firms are more complex and thus iiROAiAVGRETi ROAAVGRETTOTALPAY 0 MSFI 435 Module 9: Data Page 9-3 should award higher compensation to their CEOs. No problem: You just imagine the table in Figure 9.1 expanded to include a column for firm size and you run an expanded regression with firm size as a control (or explanatory) variable. Notice, though, that this expanded model does not bring any new requirement in terms of the econometrics of your study. At this point, running your study is, shall we say, simple, in the sense that we have “all” we need in terms of statistics. We are ready to implement any empirical analysis: Think of two measures and we can run a regression model to examine their relationship! Not so fast. Of course, there is a catch here. The econometrics of running a regression model can be in fact simple, but running a regression model is merely the last step of a study. To get there, one needs to perform other steps beforehand, and some of these steps can be quite complex and laborious. In particular, we assumed the data for the regression model, represented in Figure 9.1, was ready to go. This obviously did not happen by magic. A lot of work is required with respect to data definition (which data do we need in our analysis?), data collection (where can we find the data?), and data manipulation (how can we combine data from different sources in order to get the table in Figure 9.1?). In this module, we will examine one specific study from start to finish, with a special emphasis on data preparation for the study. You will realize that most of the effort in an applied analysis comes from the steps that take place before any statistical test is ever performed. 9.2. Steps in an Empirical Analysis In this course, we examine questions one can be answer with the analysis of data. The applied analytical approach involves several common steps. After defining a question, we need to choose the proper data and the set of statistical tests necessary to address the question. We then have to implement the tests and analyze the results. We briefly discuss each of these steps, in the context of a study of the determinants of executive compensation. Step 1: Description of the Study We want to analyze the determinants of executive compensation. Particularly, we want to examine what drives compensation of CEOs in U.S. public companies. CEO compensation is a much- discussed topic in the media—for example, with claims that such compensation is going through the roof, or that it is not necessarily linked to shareholders’ interests. Our main idea is to test whether CEO compensation is related to the performance of the firm. One prevailing view of management theory is that the CEO compensation should be linked to firm performance: You pay more when the firm performs well. This is our main hypothesis to be tested. You realize, though, that there are many other potential determinants of CEO compensation. As mentioned before, larger firms are more complex and might demand more effort from CEOs. Thus, firm size could be a determinant of compensation, and we need to add it as a control variable in our analysis. On the same token, firms with larger growth opportunities are deemed more complex and should better reward their executives Finally, some CEO characteristics—such as MSFI 435 Module 9: Data Page 9-4 experience—can be a determinant of CEO compensation. Therefore, proxies for firm growth and CEO characteristics need to be added as control variable as well. We need to examine many determinants of executive compensation—firm performance, firm size, growth prospects, CEO characteristics, etc. In this study, we focus on the effects of firm performance on compensation, while treating the other determinants of compensation as control variables. However, all these variables will be equally right-hand side (RHS) variables in a regression model. Whether a variable is your main focus or merely a control variable depends on your objective. For example, if you were interested in the CEO characteristics as the main driver of compensation, then you would treat firm performance as a control variable. Step 2: Data Requirements A brief reading of the description of the study in step (1) highlights some data needs. For example, we need data on CEO compensation, measures of firm size and of its growth prospects, and measures of CEO characteristics. A thorough search is required in order to identify whether and where this data are available, in which format, etc. Section 9.3 will discuss this step in more details. Step 3: Statistical Tools Requirements Having your main idea on hand, and the availability of data, the next step is to determine which set of statistical tools will be employed. You can anticipate that you will run a regression model where the dependent variable is the level of CEO compensation and the explanatory variables are firm performance, firm size, etc. Step 4: Implementation After establishing the data you have and the models you want to run, you need a plan to implement the analysis. This will involve a series of steps to take you from raw data into outputs from statistical tests. In our example here, these steps will prepare a table like the one shown in Figure 9.1, such that a regression model can be easily executed from the table. We discuss this step in section 9.4. Step 5: Analysis of Results This last step is the most important of the study. Here you will analyze the results of your statistical tests and extract the inferences from the study. This is discussed in section 9.5. 9.3. A Detailed Analysis of Data Requirements An extensive search uncovered three sources for the data required for our study of executive compensation. Figure 9.2 shows the tables with the data we will use in this study. First, we need data on CEO compensation. The COMPENSATION_DATA table contains such data. For each firm and year, it shows the age of the CEO (CEOAGE), the CEO salary, total cash pay (salary plus bonus) and total pay (salary plus bonus plus stock and options). The compensation variables are measured in thousands of dollars. MSFI 435 Module 9: Data Page 9-5 Figure 9-2. Tables with the data necessary for the study of executive compensation Data on firm returns come from the table MSF (an acronym for monthly stock file) in the CRSP database. It contains, for each firm and each month, the return of the firm’s stock price in that month (RET), the price (in US$) of each stock at the end of the month (PRC) and the number (in thousands) of outstanding shares (SHROUT). A firm is identified by the variable PERMNO in this table. Take the first visible row in the MSF dataset. It shows that the firm identified by PERMNO=10001 had a stock price of $9.94 on January 31st, 1990. The stock return from the day before was – 0.019=–1.9%, and the firm had about 1 millions shares outstanding on that day. MSFI 435 Module 9: Data Page 9-6 Finally, we need accounting data from the FUNDA dataset in COMPUSTAT. A portion of the dataset is represented in the figure. It shows, for each firm and each fiscal year, the amount of sales (SALE) and firm’s total assets (AT). All dollar variables are measured in millions of dollars. Here, a firm is identified by the variable GVKEY. One important task of the data requirement analysis is to figure out the primary key of each source of data. The primary key is the identifier of each table—what uniquely identifies each row of the table. In the table with executive compensation, notice that a firm identifier is not the primary key, since we have more than one row for each firm. Instead, what uniquely identifies each observation in the table is a pair of firm identifier and a period. Notice that the primary key is hinted by the way we describe a table. For the table with executive compensation, we had the description “for each firm and year, it shows …” which implies that the pair of firm and year uniquely describes a row. For the MSF table, the primary key is the pair of firm identifier (PERMNO) and period (DATE). For the FUNDA, the primary key is the pair of firm identifier (GVKEY) and firm’s fiscal year (DATADATE). The primary keys will be important to determine how to combine different tables. It also highlights one common difficulty of analyses involving multiple sources of data. Notice that while both MSF and FUNDA have data on firms, the firm identifier is different in each table. This is inevitable as data comes from different sources, and the providers of the data choose to use different identifiers for each entity. In this case, a firm is identified by the variable PERMNO in the MSF table and by the variable GVKEY in the FUNDA table. 9.4. Statistical Tools and Implementation We now get into the details of the implementation. Our main idea is to run a regression roughly expressed as Many decisions are needed in order to narrow down the exact format of the regression model and its data needs. What measures of compensation? There are many measures of compensation. We will examine the following measures: the base salary, the bonus, the amount of stocks and options, and the total pay. We can extract these measures from the variables in the COMPENSATION_DATA table. How to measure firm performance? We adopt two measures: a measure of financial performance based on stock market performance (the average monthly return over the year) and a measure of accounting performance based on return on assets. Which proxies for firm size? The regression model above refers to a general measure of size. We have different variables that can proxy for the size of the firm: total assets (AT), sales (SALE), and market value of equity. Which one should we adopt in our regression? One can argue that each variable is a iiSIZEiROAiAVGRETi ...SIZEROAAVGRETONCOMPENSATI 0 MSFI 435 Module 9: Data Page 9-7 reasonable proxy for firm size. Since a priori we do not know which ones are preferable, we can adopt all of them in our model. Which proxy for growth opportunities? It is common to use the ratio of market value of equity to book value of equity as a proxy for growth opportunities. These measures are obtained from the dataset FUNDA: the variable BKVLPS contains book value per share and PRCC_F contains market value per share. We adopt this proxy as well. Which period to use? We have roughly 10 years of compensation data. We could run the regression using the entire sample, but we will see later that this can be problematic. Instead, we opt to run the regression on one single cross-section period—the year 2000. Should we use log values of the variables? We adopt log measures of proxies for firm size and growth opportunities. As before, we use logarithm to account for possible nonlinearity in the relationship between the dependent and independent variables. For compensation measures, we will explore two alternatives, with and without the log specification. Interpretation of the coefficients needs to take into consideration this choice. Should we use contemporaneous measures of RHS and LHS variables? No. We will lag by one year the independent variables that proxy for firm performance, firm size and firm growth options. For example, we will regress compensation measured in year 2000 on the proxies for firm performance measured during the year 1999. Our regression setup thus serves a predictive role: given the measures of performance, firm size, and growth options, we can predict the compensation in the following year. Lagging RHS variables is a common approach to address endogeneity concerns related to reverse causality. Specifically, if we regress compensation measured in year t on performance measured in year t as well, it is difficult to establish whether performance is driving compensation or whether compensation is driving performance. Notice that examining whether compensation is driving performance is also a very important question, but not the one being explored in this analysis. With these details on what exactly we are examining, we are ready to implement our analysis. Our main effort involves preparing a table like the one in Figure 9.1 from the original data in the tables shown in Figure 9.2. With the table in Figure 9.1 ready to go, we can then run regression models. We implement this study with an algorithm formed by the following steps: 1. Read the data on executive compensation 2. For each firm and year, compute average return in the previous year 3. For each firm and year, collect accounting data measured in the previous year 4. Define regression variables 5. Run the regressions MSFI 435 Module 9: Data Page 9-8 We now discuss each step and how to implement them in SAS. The complete code appears in section 9.7. Step 1. Read the data on executive compensation This could be simply a DATA step reading a SAS dataset. However, data on executive compensation was supplied in an Excel spreadsheet (names “compensation_data.xlsx” (available on Canvas). We can use the PROC IMPORT statement to read an Excel file into a SAS dataset: proc import datafile="U:\Teaching\MSFI 435\Data\compensation_data.xlsx" out=lib435.compensation_data_sas replace; sheet='Compensation'; Notice we referred to the spreadsheet inside Excel that contains the data. We also request SAS to create a permanent dataset COMPENSATION_DATA_SAS in the library title LIB435. We then proceed to read this new dataset into a temporary dataset named D. At this point, everybody should be familiar with each dataset, either through the use of PROC PRINT, PROC CONTENTS, or via opening of the dataset using the Table View feature of the SAS Windows Environment. We include in our code a PROC CONTENTS for each dataset used in the examination. Step 2. For each firm and year, compute average return in the previous year Recall that we are lagging most of the RHS variables—hence the need to compute the average return in the year prior to the year when compensation data is observed. Lines 23-64 process this requirement. The primary key of our compensation data is the pair (PERMNO, YEAR). Thus, for each such pair, we collect monthly return data in the previous year. Notice that, as usual, this is being implemented in the WRDS server. The return data is stored in the dataset D_RET. The PROC MEANS in line 45 then generates a table with the average return computed, as established through the BY clause, for each pair of PERMNO and YEAR. Since D_RET contains monthly return for all months in the previous year, the average return, stored in AVGRET, refers to the average return in the previous year. The output is stored in the dataset D_STATS. (As always, PROC MEANS requires the dataset to be pre-sorted; this is accomplished by the ORDER BY specification in the previous PROC SQL.) Finally, the PROC SQL starting in line 50 combines the contents of D_STATS back into our main dataset D. We end the procedure by downloading the data away from the WRDS servers. Step 3. For each firm and year, collect accounting data measured in the previous year We now combine our main dataset D with the accounting variables in dataset FUNDA. Care is required in that now the firm identifier to be used is GVKEY, so we first collect the GVKEY for each firm (as we had done in Module 7). Again, we combine compensation data in year t with MSFI 435 Module 9: Data Page 9-9 accounting data in year t–1. The whole procedure, which mimics the one we used in module 7, appears in lines 66-131. Step 4. Define regression variables The DATA step in line 134 defines the variables. First, we need to define BONUS and STOCKOPTION. Second, we need to define the logarithm versions of the measures of compensation, firm size, and firm growth options. Step 5. Run the regressions The regression models are implemented starting on line 164. Notice that before running the regression we restrict the sample to have observations from year=2000 only, and we also request an output containing some basic summary statistics on the variables available in dataset D (when the PROC MEANS has no VAR statement, it means it refers to all variables in the dataset). 9.5. Analysis of Results We show in Figure 9.3 the summarized results of regression models explaining the logarithm version of SALARY, BONUS, OPTIONS, and TOTALPAY. For each coefficient, we show the estimate and its t-statistic (in brackets). Let’s look at the first column, related to the regression model For now we are ignoring all other control variables. The results suggest a strong influence of past performance on total pay. Both the coefficients on average return and return on assets are significantly different from zero. Since we use the log version of the dependent variable, analyzing the effect of performance involves a log-lin specification: the OLS coefficient will measure a percentage change in the dependent variable for each one-unit change in the independent variable. Since AVGRET is expressed in percentage terms (line 138 shows that returns are multiplied by a 100), a one-unit change in average return means an increase in 1%. Thus, the regression result suggests that a 1% extra in average returns in the previous year increase the total compensation package of the firm’s CEO by a rate of 0.0128, or 1.28%. For the measure of return on assets, again we report a significant effect on total compensation: an extra 1% in return on asset implies a rate of increase of 0.0070, or 0.70%, in total compensation for the firm’s CEO. However, the omitted variable bias shows up here as well. When we expand the regression with measures of firm size, growth options and CEO age, the coefficient on return on assets is no longer significant, and the coefficient on average returns is in fact magnified. As for the control variables, we observe significantly positive effects of firm size and growth options. To interpret these relationships, we now have to account for the fact that both the dependent and the independent variables rely on the log specification—that is, we have a log-log specification. Now, the rate of change in total pay for a 1% change in the measure of size equals iiROAiAVGRETi ROAAVGRETLTOTALPAY 0 MSFI 435 Module 9: Data Page 9-10 the coefficient divided by 100. The estimated value of 0.1426 for the coefficient on LSALE thus implies that a 1% difference in sales in the previous year implies an increase of 0.1426% in total pay in the current year. For total assets, a 1% increase is associated with an increase of 0.2786% in compensation. Figure 9-3. Regressions results explaining the log specification of compensation measures We then break total compensation into its components—salary, bonus, and stocks and options— and analyze each one in separate. The most striking aspect is that while the two proxies for past performance do not seem to matter at all for salary, they are highly significant for the bonus component. In fact, a more formal test of the statement that the two proxies of past performance are irrelevant in the determination of salary can be accomplished. For that, we test H0: βAVGRET=βROA=0 Ha: βAVGRET ≠ 0 or βROA ≠ 0 This is implemented by a TEST statement within a PROC REG (see lines 189-191). The results indicate a F-statistic of 0.12, with a p-value of 0.8852, so indeed we fail to reject the null that past performance has no say in the salary portion of executive compensation. MSFI 435 Module 9: Data Page 9-11 Figure 9-4. Regressions results explaining the raw measures of compensation Should we examine the compensation measures without the log specification? Figure 9.4 shows such specification. The advantage of this alternative is that it provides changes in compensation based on dollar values. Which one to use, though, depends on the assumptions of your model. Is it reasonable to assume a linear relationship between average returns and dollar amounts of compensation, or between average returns and rates of changes in compensation? All these inferences depend on the assumptions of the linear regression model being valid. We check for them. We perform the various tests of misspecification discussed in module 8. For simplicity, we discuss the results for the model explaining the log of salary. Results from the White test show no sign of heteroscedasticity; from the Durbin-Watson test we see no sign of autocorrelation; and the execution of the RESET test does not suggest the need of inclusion of nonlinearities in our models. Does the study stop here? The answer is, unfortunately, no. There are many other determinants of CEO compensation that are not controlled for in our examination. For example, it has been shown that many other CEO characteristics—such as CEO tenure; whether the CEO holds the position of Chairman of the Board of Directors; how many connections in the industry a CEO has—also influence compensation. Extra care is, thus, required to make sure these omitted variables are not MSFI 435 Module 9: Data Page 9-12 bringing bias to our study—or else we need to include them in the study! But this, we leave for another opportunity.1 9.6. A Detour: Prediction We can use a regression model to predict outcomes. In our analysis of executive compensation, we can use our model to predict the compensation of a CEO or group of CEOs given the characteristics that drive compensation—the characteristics we adopted in our model. Say we observe a firm having some level of firm size, of past performance, of growth opportunities, etc, and we would like to know what compensation could we predict from our model for that firm’s CEO. We have already performed similar exercises in different setups: Recall our model in assignment 6 explaining real estate sales prices. We used the model to predict sales prices. For a house that has its land appraised at $39,000, its construction (home value) appraised at $149,000, and with a living area of 1,950 square feet real, the model predicts the sales price to be $181,760.20. Or, in the model explaining hourly wage in module 5, we could predict the wage given some level of education. We discussed that for a worker with 12 years of education, the model predicts her wage to be –4.47+1.28*12=10.89. Notably absent in those analyses was the uncertainty regarding that prediction. After all, we based the prediction on estimates obtained from one specific sample—the sample used to run the regression model. If we reestimated the model with a different sample, the prediction would have been different. In other words, a prediction is a random variable. As such, it has a distribution, with its expected value and variance. All that we did in the exercises above was to compute the expected value of that random variable. Now we expand the discussion and think about the variance associated with the prediction. We want to incorporate the uncertainty of our prediction in the analysis. In particular, we want to answer the question about how close our prediction is to the true value of the variable under analysis. We can do that by building a confidence interval for the true value of the variable. Here we need to make a distinction between two types of prediction: one is the prediction of the outcome (the dependent variable in our model) for a single observation, and the other is the prediction of the mean value of the dependent variable for the group of observations having some fixed values of the independent variables. To make the distinction more clear, take the model of wage as a function of years of education, 1 Many other inferences were ignored in this discussion. We did not pay much attention to the effects of CEO age (results are mixed) or to the resiliency of the size proxies in each of the components of the CEO compensation package. One can also argue that some empirical choices in section 9.4 might not be the best ones. Why, for example, focus on past year’s performance alone? An alternative would be to extend performance to include, let’s say, the previous 3 years. These, and other, robustness checks and extensions are left as an exercise for the reader. MSFI 435 Module 9: Data Page 9-13 where Yi is wage and Xi is years of education. There are two types of predictions that we can take from the model: 1. Suppose we want to ask the question “What would workers with 12 years of education earn on average?” Here we are not concerned with one single observation, but rather with all observations with some specific value(s) of the independent variable(s). This is known as the “predicted mean response.” 2. Now suppose a new worker appears in the market and she has 12 years of education. We want to use the model to predict her wage. This is the predicted wage for one specific observation, sometimes referred to as a “prediction of a future value, or individual prediction”; Let’s focus first on the predictor of the mean response. All we need to predict is the amount β0+β1Xi. We can use the coefficient estimates 0 and 1 from our model to get this prediction. The prediction of the mean response given some baseline level Xi=Xa (in our case, Xa=12) is thus =0+1Xa. Given the random variable nature of OLS coefficients, our predictor function is also a random variable. Since the OLS coefficients are unbiased, we derive that E[]= β0+β1Xa. Also, it can be shown that the variance of this estimator is given by which we can approximate as Moreover, as usual, in large samples the distribution of this estimator can be approximated as normal. This gives us a way to compute confidence intervals for the mean response. From the approximation we can derive that a 95% confidence interval for the mean response β0+β1Xa is iii XY 10 n i i a )XX( )XX( n )ˆ(Var 1 2 22a 1Y )s.Yˆ,s.Yˆ( aaaa 961961 n i i a )XX( )XX( n ss 1 2 222a 1 aaa ,XN~Yˆ 00 MSFI 435 Module 9: Data Page 9-14 For our baseline level of Xa=12, such interval becomes (10.48,11.31). Recall the concept of a 95% confidence interval: it means that 95% of intervals built this way (or, 95% of the samples would yield intervals that) would contain the true mean wage β0+β1Xa . Let’s now examine the predictor of the outcome for an individual observation. Here we want to predict the outcome Yi=β0+β1Xi+εi for some specific worker having Xi=Xa, or Ya=β0+β1Xa+εa. Given that the expected value of the error term is zero, our best predictor of this future value is again =0+1Xa. However, when assessing the variance of this prediction we need to account for the variance of the error term. It can be shown that, under some mild conditions, the random variable can be approximated as a standard normal, where we have So, again we can derive a confidence interval for the quantity under analysis, Ya. For a confidence level of 95%, we have For our baseline level of Xa=12, such interval becomes (–2.89, 24.68). Again, from the concept of a 95% confidence interval, 95% of the samples would yield intervals containing the true wage for the specific worker with 12 years of education. In summary, according to the wage model the best predictor for the mean wage of workers with 12 years of education is $10.89, while at the same time the best predictor for the wage of an individual worker with 12 years of education is also $10.89. On the other hand, a 95% confidence interval for the mean wage of workers with 12 years of education is (10.48,11.31), while a 95% confidence interval for the actual wage of a specific worker with with 12 years of education is (– 2.89, 24.68). While point estimates of the two types of predictions are the same, their confidence intervals differ quite a lot. In particular, the large confidence interval of “individual” predictions illustrates the difficulty of learning about one single outcome. Figure 9.5 illustrates this point. It shows all the workers surveyed in our wage study. See that the actual wages for someone with 12 years of experience can be as large as $38.45 and as low as $1.15 (though the bulk of the observations rests between the 1st-percentile= $3.08 and the 99th-percentile=$27.70). For the overall sample, the standard deviation of the error term is about $7, which roughly implies a two standard deviation new,a aa s YˆY n i i a )XX( )XX( n ss 1 2 222newa, 11 )s.Yˆ,s.Yˆ( new,aanew,aa 961961 MSFI 435 Module 9: Data Page 9-15 band around the predicted wage $10.89 yields an interval of (–3.11,24.89), not far from our estimates. Figure 9-5. All the data points in the wage vs. education study Notice how “individual” predictions fare much worse than predictions about the mean effect. This is just natural, since in the mean prediction the idiosyncratic effects of each observation tend to cancel out as we mix the many observations with the same level of the independent variable. The expressions to compute confidence intervals have to be generalized in the case of the multiple regression model. As they involve operations on matrices, we will skip the details here. The good thing is that you do not need to worry about these expressions. A statistical package like SAS can provide these confidence intervals as part of a regression procedure. The following syntax represents one such code, this time to compute confidence intervals for the wage regression: proc reg data=d; model wage=education; output out=reg_data predicted=p residual=r lcl=l ucl=u lclm=lm uclm=um; Here as part of the OUTPUT OUT= statement one can ask SAS to produce the confidence intervals for mean response (variables created through the syntax LCLM= and UCLM=) and for an individual outcome (variables created through LCL= and UCL=). The syntax in the last PROC REG in the code analyzing executive compensation prepares a similar output. Find below the output for one observation from the subsequent PROC PRINT. It shows MSFI 435 Module 9: Data Page 9-16 the prediction for observations with AVGRET=12.12, roa=12.48, LSALE=9.36, LAT=9.03, lbm=2.49 and CEOAGE=45. If we want a 95% confidence interval for the salary of a CEO of a specific firm with those characteristics, we get (397.16,1432.16). However, if we are looking for the mean salary of CEOs working for firms having those characteristics, a 95% confidence interval would be given by (870.91, 958.42). avgret roa lsale lat lmb CEOage 12.121140 12.2481 9.3696 9.0384 2.40934 45 salary p l u lm um 103.85 914.66 397.163 1432.16 870.91 958.42 9.7. SAS Summary This module worked for the first time on an example of the following SAS feature: PROC IMPORT The TEST statement in a PROC REG Options LCL, UCL, LCLM, and UCLM in PROC REG 9.8. The Complete Code We show in the figure below the complete code used in this module. 1 2 3 4 5 6 7 8 9 10 11 12 13 14 15 16 17 18 19 20 21 22 23 24 25 26 27 /****************************************************** Name: m9_analyze_compensation_w Date: 12/31/2012 Description: combine data in order to examine the determinants of executive compensation ******************************************************/ options ls=80 nodate nonumber; libname lib435 'U:\Teaching\MSFI 435\SAS\lib'; proc import datafile="U:\Teaching\MSFI 435\data\compensation_data.xlsx" out=lib435.compensation_data_sas replace; sheet='Compensation'; proc contents data=lib435.compensation_data_sas; data d; set lib435.compensation_data_sas; run; *Measure past year average return; %let wrds=wrds-cloud.wharton.upenn.edu 4016; options comamid=TCP remote=WRDS; signon username=_prompt_; rsubmit; MSFI 435 Module 9: Data Page 9-17 28 29 30 31 32 33 34 35 36 37 38 39 40 41 42 43 44 45 46 47 48 49 50 51 52 53 54 55 56 57 58 59 60 61 62 63 64 65 66 67 68 69 70 71 72 73 74 75 76 77 78 79 80 81 proc upload data=d; run; proc sql; create table d_ret as select a.*, b.ret from d as a,crsp.msf as b where a.permno=b.permno and year(b.date)=a.year-1 order by permno,year; proc means data=d_ret noprint; by permno year; output out=d_stats mean(ret)=avgret; proc sql; create table d as select a.*, b.avgret from d as a left join d_stats as b on a.permno=b.permno and a.year=b.year; proc download data=d; run; endrsubmit; proc sql; create table d_acct as select a.permno, a.year, b.gvkey from d as a,lib435.mapping_permno_gvkey as b where a.permno=b.permno and b.begin_year<=a.year<=b.end_year; run; %let wrds=wrds-cloud.wharton.upenn.edu 4016; options comamid=TCP remote=WRDS; signon username=_prompt_; MSFI 435 Module 9: Data Page 9-18 82 83 84 85 86 87 88 89 90 91 92 93 94 95 96 97 98 99 100 101 102 103 104 105 106 107 108 109 110 111 112 113 114 115 116 117 118 119 120 121 122 123 124 125 126 127 128 129 130 131 132 133 134 135 rsubmit; libname comp '/wrds/comp/sasdata/nam'; proc upload data=d_acct; proc sql; create table d_acct as select a.*, b.bkvlps, b.prcc_f, b.csho, b.at, b.ni, b.sale from d_acct as a,comp.funda b where a.gvkey=b.gvkey and a.year-1=year(b.datadate) and indfmt="INDL" and datafmt='STD' and popsrc='D' and consol='C' order by permno,year,datadate desc; proc sort data=d_acct nodupkeys; by permno year; proc download data=d_acct; run; endrsubmit; proc sql; create table d as select a.*, b.bkvlps, b.prcc_f, b.csho, b.at, b.ni, b.sale from d as a left join d_acct as b on a.permno=b.permno and a.year=b.year; run; *Define regression variables; data d; MSFI 435 Module 9: Data Page 9-19 136 137 138 139 140 141 142 143 144 145 146 147 148 149 150 151 152 153 154 155 156 157 158 159 160 161 162 163 164 165 166 167 168 169 170 171 172 173 174 175 176 177 178 179 180 181 182 183 184 185 186 187 188 189 set d; avgret=100*avgret; roa=100*ni/at; bonus=cashpay-salary; stockoption=totalpay-cashpay; lsalary=log(salary); lstockoption=log(totalpay-cashpay); lbonus=log(cashpay-salary); ltotalpay=log(totalpay); mb=prcc_f/bkvlps; me=prcc_f*csho; lsale=log(sale); lat=log(at); lmb=log(prcc_f/bkvlps); data d; set d; where year=2000; data lib435.d_compensation; set d; run; proc means data=d nolabels n nmiss mean median min max; title "Summary statistics"; proc reg data=d; title "Regression results"; model ltotalpay=avgret roa; model totalpay=avgret roa; model ltotalpay=avgret roa lsale lat lmb ceoage; model totalpay=avgret roa lsale lat lmb ceoage; proc reg data=d; model lsalary=avgret roa; model salary=avgret roa; model lsalary=avgret roa lsale lat lmb ceoage; model salary=avgret roa lsale lat lmb ceoage; proc reg data=d; model lbonus=avgret roa; model bonus=avgret roa; model lbonus=avgret roa lsale lat lmb ceoage; model bonus=avgret roa lsale lat lmb ceoage; proc reg data=d; model lstockoption=avgret roa; model stockoption=avgret roa; model lstockoption=avgret roa lsale lat lmb ceoage; model stockoption=avgret roa lsale lat lmb ceoage; proc reg data=d; MSFI 435 Module 9: Data Page 9-20 190 191 192 193 194 195 196 197 198 199 200 201 202 203 204 205 206 207 208 209 model lsalary=avgret roa lsale lat lmb ceoage/ spec acov dw dwprob; test avgret=0,roa=0; proc autoreg data=d; model lsalary=avgret roa lsale lat lmb ceoage/reset; proc reg data=d; model salary=avgret roa lsale lat lmb ceoage; output out=reg_data predicted=p residual=r lcl=l ucl=u lclm=lm uclm=um; proc print data=reg_data (obs=10)noobs; var avgret roa lsale lat lmb ceoage salary p l u lm um; run; Figure 9-6. Complete SAS code examining the determinants of CEO compensation